An adversarial variational inference approach for travel demand calibration of urban traffic simulators.
SIGSPATIAL/GIS(2022)
摘要
This paper considers the calibration of travel demand inputs, defined as a set of origin-destination matrices (ODs) , for stochastic microscopic urban traffic simulators. The goal of calibration is to find a (set of) travel demand input(s) that replicate sparse field count data statistics. While traditional approaches use only first-order moment information from the field data, it is well known that the OD calibration problem is underdetermined in realistic networks. We study the value of using higher-order statistics from spatially sparse field data to mitigate underdetermination, proposing a variational inference technique that identifies an OD distribution. We apply our approach to a high-dimensional setting in Salt Lake City, Utah. Our approach is flexible---it can be readily extended to account for arbitrary types of field data (e.g., road, path or trip data).
更多查看译文
关键词
travel demand calibration,adversarial variational inference approach,urban traffic simulators
AI 理解论文
溯源树
样例
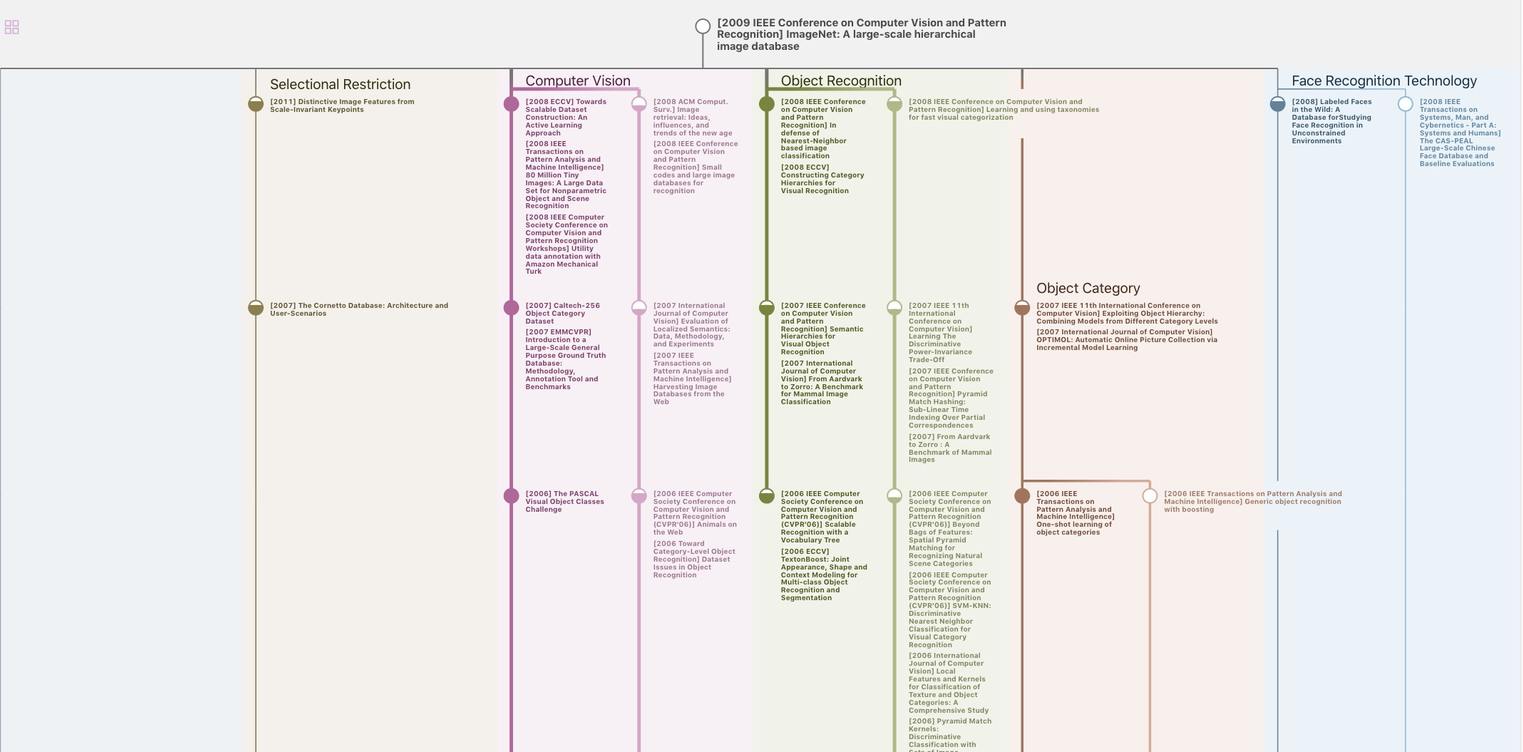
生成溯源树,研究论文发展脉络
Chat Paper
正在生成论文摘要