Factorized deep generative models for end-to-end trajectory generation with spatiotemporal validity constraints.
SIGSPATIAL/GIS(2022)
摘要
A growing number of research areas such as location-based social networks, intelligent transportation systems, and urban computing utilize large amounts of trajectory data for benchmarking data management approaches and analysis methods. Given the general lackness of available large datasets, realistic synthetic trajectory datasets become important. This work proposes deep generative models for trajectory data that can learn disentangled models for sophisticated latent patterns. Existing methods rely on predefined heuristics and cannot learn the unknown underlying generative mechanisms. The proposed novel deep generative VAE-like models factorize global and local semantics (habits vs. random routing change). We further develop new inference strategies based on variational inference and constrained optimization to encapsulate spatiotemporal validity. New deep neural network architectures are developed to implement generative and inference models with dynamic latent priors. The proposed methods represent significant quantitative and qualitative improvements over existing approaches as demonstrated by extensive experiments. The software is made publicly available 1 .
更多查看译文
AI 理解论文
溯源树
样例
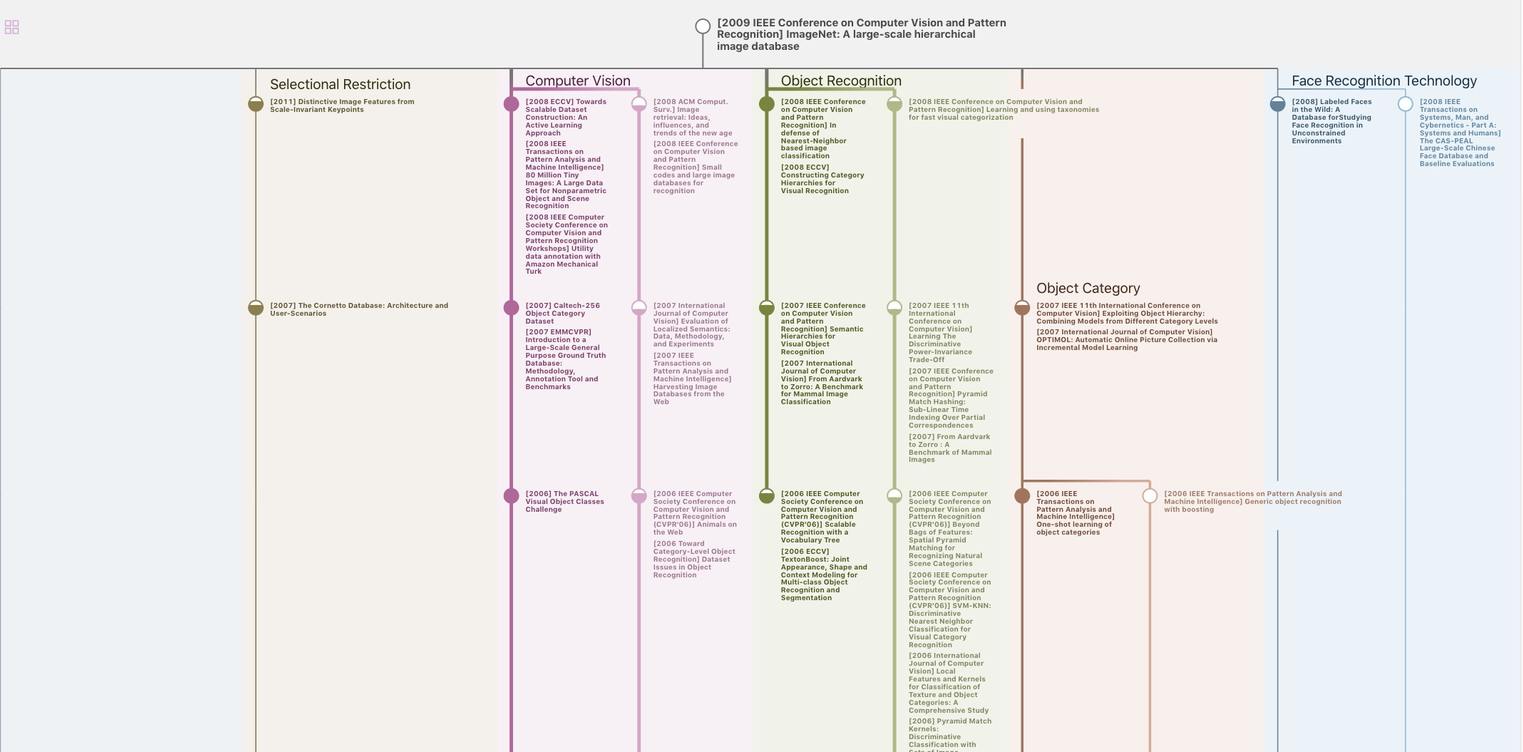
生成溯源树,研究论文发展脉络
Chat Paper
正在生成论文摘要