FL-MFGM: A Privacy-Preserving and High-Accuracy Blockchain Reliability Prediction Model.
BlockSys(2022)
摘要
Reliability prediction mechanism plays an important role in selecting reliable peers for blockchain users. Traditionally, reliability prediction approaches are performed by collecting a large amount of user data and conducting centralized training. Although these methods can achieve high accuracy, they may lead to malicious attacks by third-party services on users' private data. As a privacy-preserving approach to addressing privacy leakage caused by centralized training, federated learning can enable users to learn a shared prediction model by providing gradients to a central server instead of raw data. However, uploading complete gradients directly may be exposed to a gradient attack and cause privacy leakage. To address the privacy data leakage from centralized training and prevent the attack threat from uploading gradients, we propose a privacy-preserving and high-accuracy blockchain reliability prediction model, namely FL-MFGM. Based on federated learning architecture, the model protects user privacy by uploading the gradients of matrix factorization. Specifically, in order to enhance the privacy-preserving capability of our model, we employ gradient compression with momentum correction for federated matrix factorization. To validate the effectiveness of our approach, sufficient experiments are conducted, which indicate that our approach can achieve higher prediction accuracy than other approaches with privacy-preserving based on federated learning architecture.
更多查看译文
关键词
Blockchain, Reliability prediction, Privacy protection, Federated learning, Matrix factorization
AI 理解论文
溯源树
样例
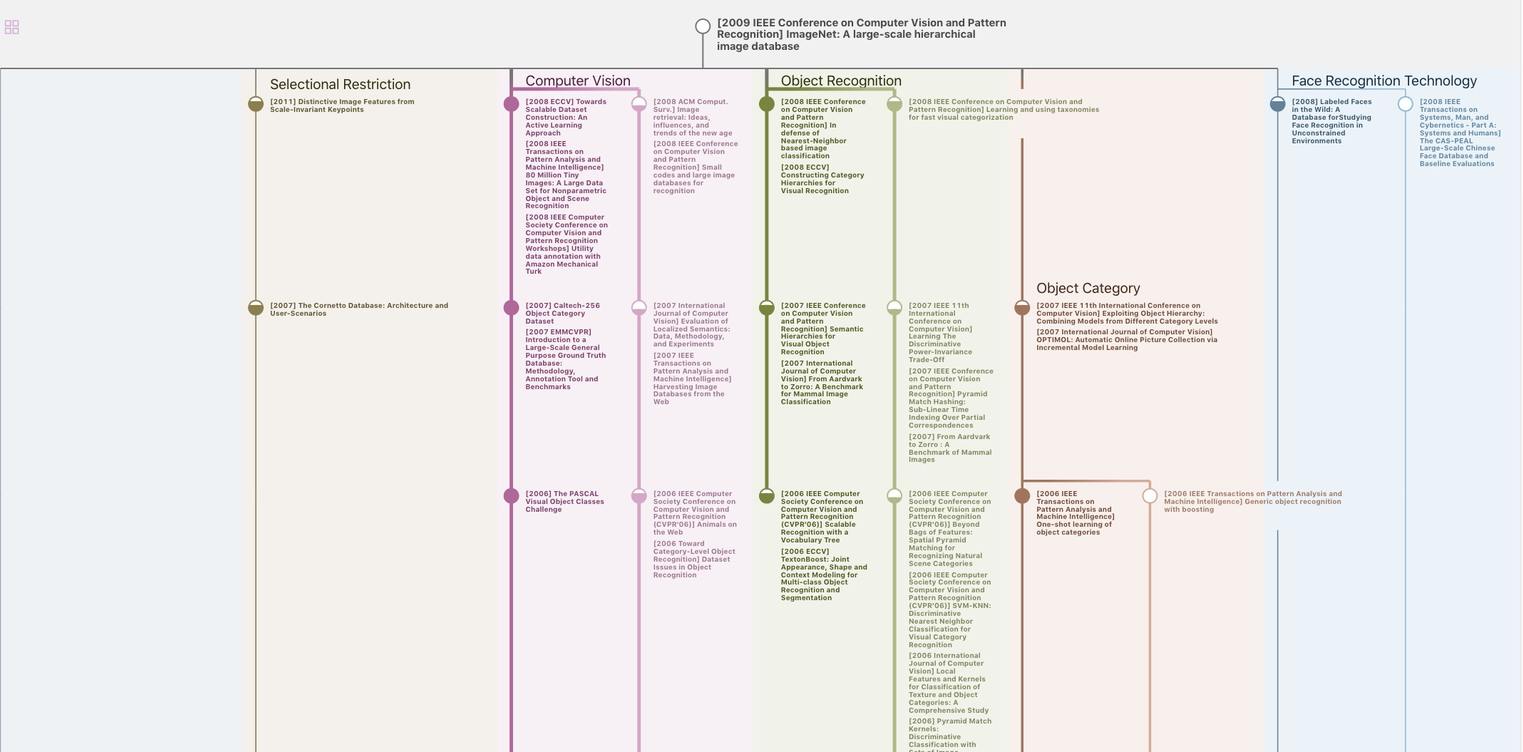
生成溯源树,研究论文发展脉络
Chat Paper
正在生成论文摘要