Mining Taxonomy-aware Colocations: A Summary of Results
30TH ACM SIGSPATIAL INTERNATIONAL CONFERENCE ON ADVANCES IN GEOGRAPHIC INFORMATION SYSTEMS, ACM SIGSPATIAL GIS 2022(2022)
Abstract
Given a collection of Boolean spatial feature-types, their instances, a neighborhood relation (e.g., proximity), and a hierarchical taxonomy on the feature-types, taxonomy-aware colocation pattern discovery finds the subsets of feature-types or their parents frequently located together. Taxonomy-aware colocations are important due to their use in taxonomy-reliant societal applications in ecology (e.g., finding new symbiotic relationships across food-chain), spatial pathology (e.g., immunotherapy for cancer), etc. Due to the taxonomy, the number of candidate patterns increases considerably (i.e., exponential in the number of colocated instances, where a subset of instances have a parent-child relation). Existing algorithms for mining general colocations are not designed to use taxonomy and will incur redundant computations across the hierarchy. We propose a taxonomy-aware colocation miner (TCM) algorithm which uses a user-defined taxonomy to find taxonomy-aware colocation patterns. We also propose TCM-Prune algorithm that prunes duplicate colocations instances having a parent-child relation. Experiments with synthetic and real data sets show that TCM and TCM-Prune can find colocation patterns missed by the traditional approach (i.e., the ones which do not take hierarchy into account), and TCM-Prune can remove duplicate colocation instances.
MoreTranslated text
Key words
Colocation,Taxonomy-aware pattern mining,Pruning
AI Read Science
Must-Reading Tree
Example
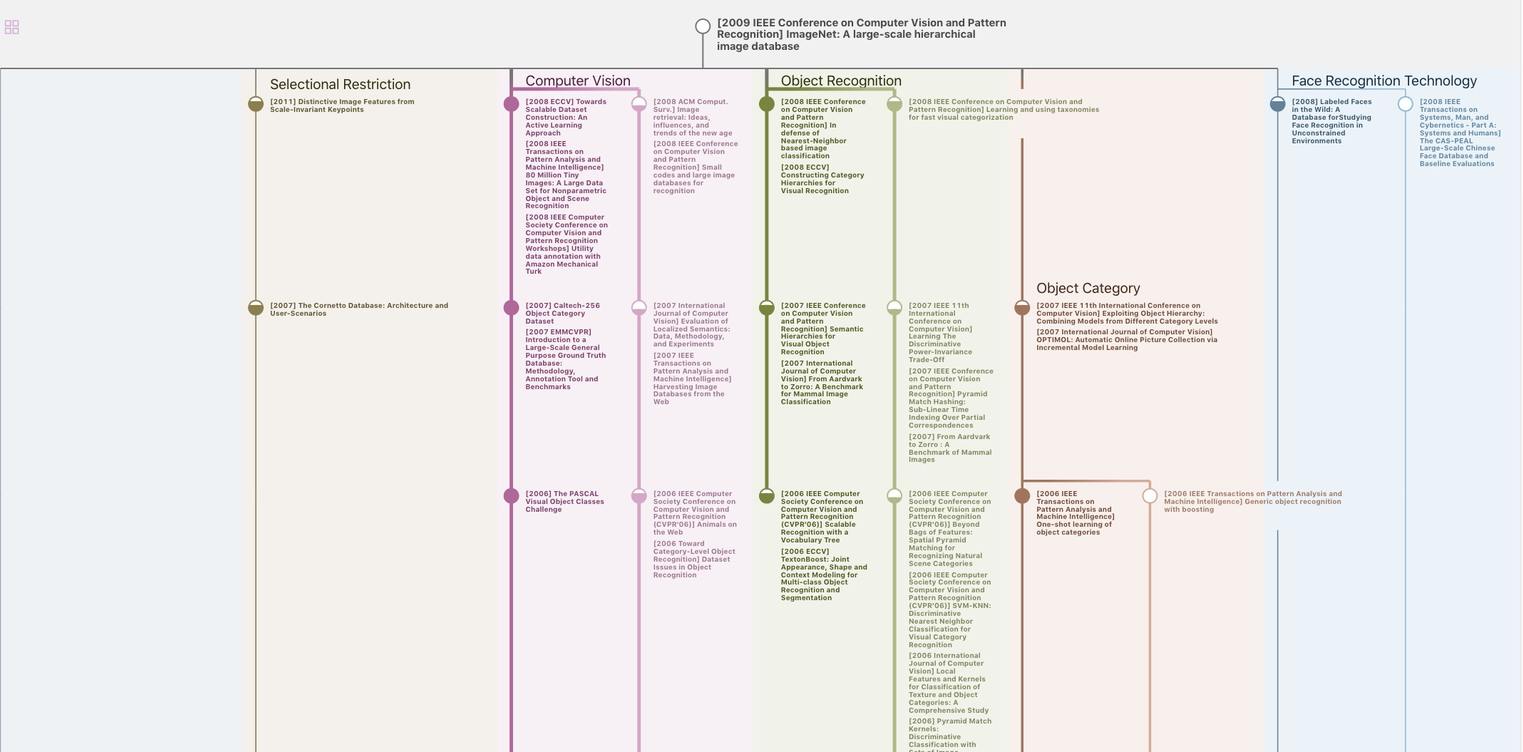
Generate MRT to find the research sequence of this paper
Chat Paper
Summary is being generated by the instructions you defined