An improved quantum algorithm for data fitting
PHYSICA A-STATISTICAL MECHANICS AND ITS APPLICATIONS(2023)
摘要
As researchers focus on the field of quantum machine learning, there are more and more quantum algorithms for data fitting. However, the majority of quantum algorithms for data fitting are only applicable to sparse matrices, which bring a large number of limitations to their application. In this paper, to solve this problem, we propose a quantum algorithm for data fitting for general matrices, not only sparse and dense matrices. The quantum algorithm is mainly composed of three subroutines which are generating target state, quantum state tomography and estimating fit quality. The time complexity of the quantum algorithm is O (kappa 4 parallel to A parallel to Fpolylog(MN)(M ' 2 log(M ')2/epsilon 3+ 1/(epsilon epsilon 2))), where A is an element of RMxN, kappa is the conditional number of matrix A dagger A is an element of RNxN, ||A||F is the Frobenius norm of A, M ' is the maxima number of fit functions in allowing fit, epsilon is the fitting error, and epsilon is the error of fit quality estimation. Moreover, our quantum algorithm is independent of the sparsity of the matrix, so our algorithm has wide application in dense matrices and poses a new state of the art for solving dense data fitting. (c) 2023 Elsevier B.V. All rights reserved.
更多查看译文
关键词
Quantum algorithm,Quantum machine learning,Data fitting
AI 理解论文
溯源树
样例
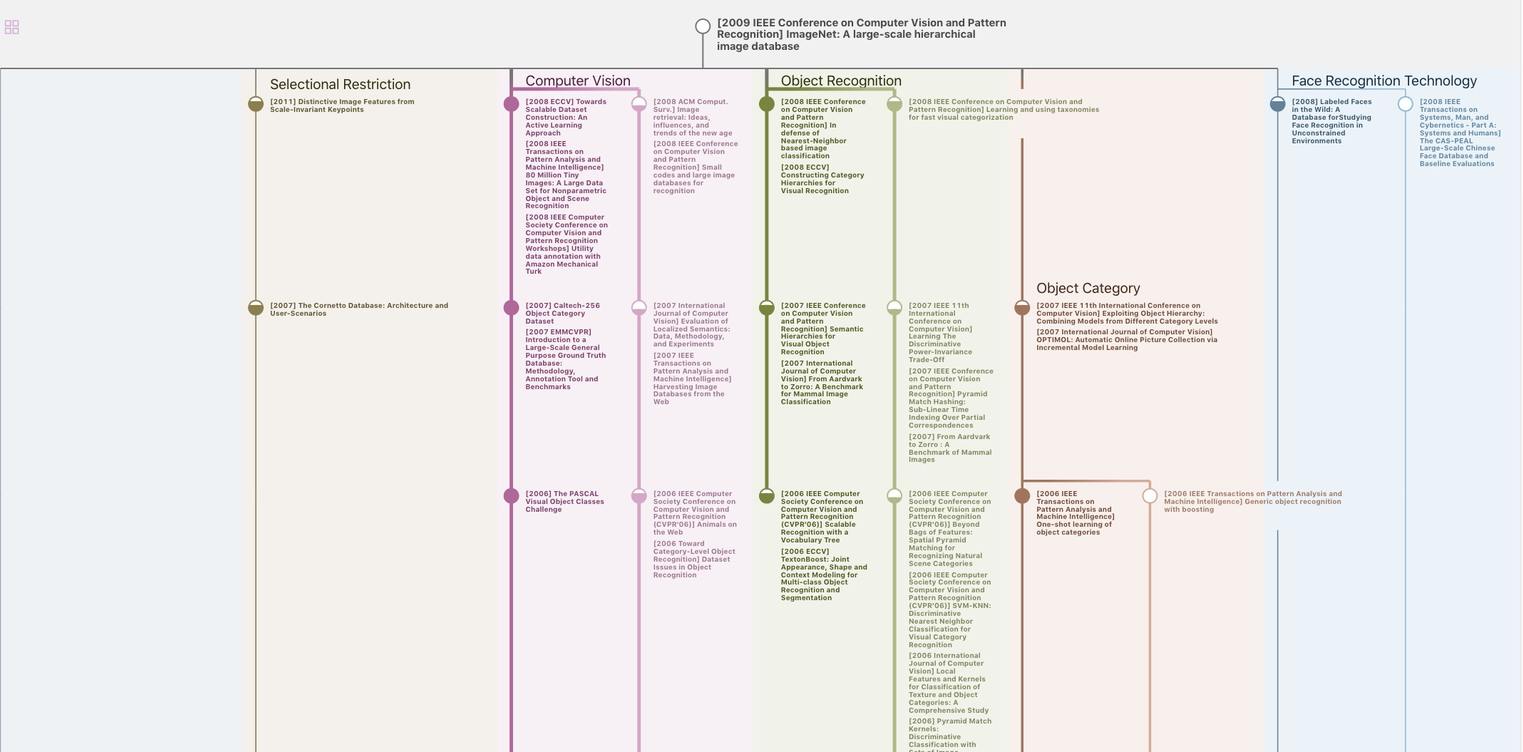
生成溯源树,研究论文发展脉络
Chat Paper
正在生成论文摘要