On Contrasting YAGO with GPT-J: An Experiment for Person-Related Attributes.
KGSWC(2022)
摘要
Language models (LMs) trained or large text corpora have demonstrated their superior performance in different language related tasks in the last years. These models automatically implicitly incorporate factual knowledge that can be used to complement existing Knowledge Graphs (KGs) that in most cases are structured from human curated databases. Here we report an experiment that attempts to gain insights about the extent to which LMs can generate factual information as that present in KGs. Concretely, we have tested such process using the English Wikipedia subset of YAGO and the GPT-J model for attributes related to individuals. Results show that the generation of correct factual information depends on the generation parameters of the model and are unevenly balanced across diverse individuals. Further, the LM can be used to populate further factual information, but it requires intermediate parsing to correctly map to KG attributes.
更多查看译文
关键词
YAGO,Ontology,Language models,GPT-J,Factual information
AI 理解论文
溯源树
样例
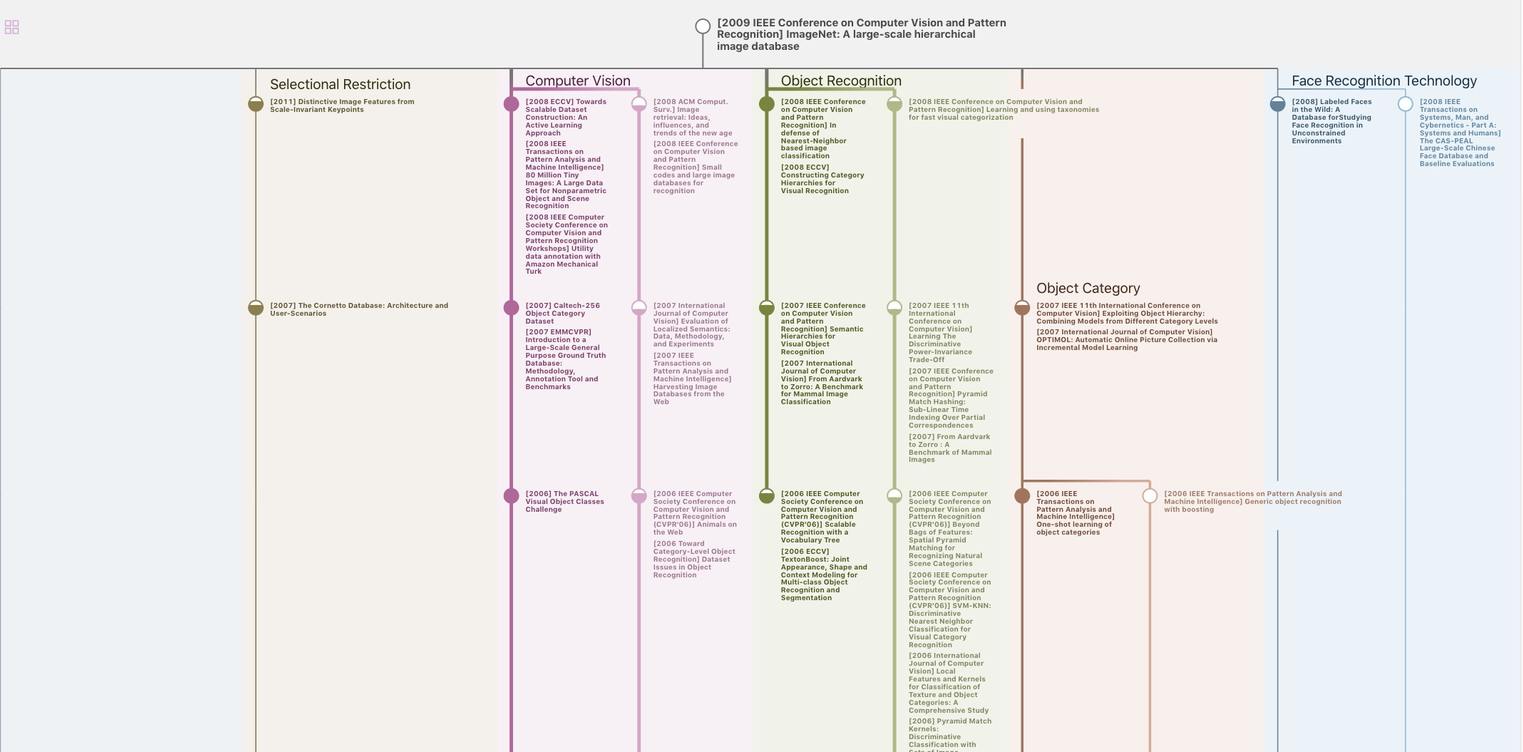
生成溯源树,研究论文发展脉络
Chat Paper
正在生成论文摘要