"I do not know": quantifying uncertainty in neural network based approaches for non-intrusive load monitoring.
BuildSys@SenSys(2022)
Abstract
Non-intrusive load monitoring (NILM) refers to the task of disaggregating total household power consumption into the constituent appliances. In recent years, various neural network (NN) based approaches have emerged as state-of-the-art for NILM. In conventional settings, NN(s) provide point estimates for appliance power. In this paper, we explore the question - can we learn models that tell when they are unsure? Or, in other words, can we learn models that provide uncertainty estimates? We explore recent advances in uncertainty for NN(s), evaluate 14 model variants on the publicly available REDD dataset, and find that our models can accurately estimate uncertainty without compromising on traditional metrics. We also find that different appliances in their different states have varying performance of uncertainty. We also propose "recalibration" methods and find they can improve the uncertainty estimation.
MoreTranslated text
Key words
Neural networks, Non-Intrusive Load Monitoring, Bayesian analysis, Uncertainty, Calibration
AI Read Science
Must-Reading Tree
Example
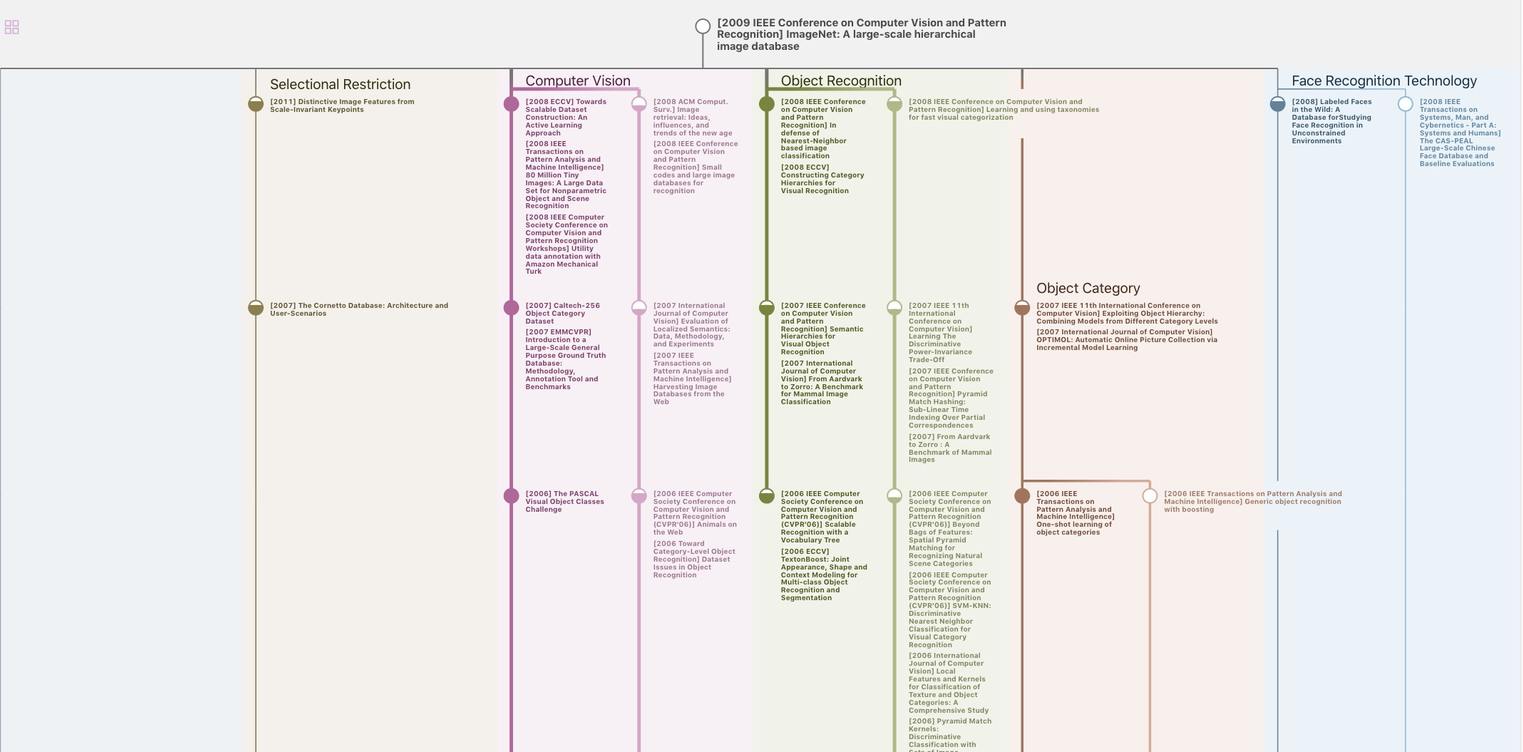
Generate MRT to find the research sequence of this paper
Chat Paper
Summary is being generated by the instructions you defined