Boosting Item Coverage in Session-Based Recommendation.
SCC(2022)
摘要
Traditional recommender systems that rely heavily on user profiles or historical consumption of users are more susceptible to the cold start and model drift limitations due to dynamic user preferences. Recent advances in recommendations have seen a shift towards session-based recommender systems, which provide recommendations solely based on a user’s interactions in an ongoing session. As a result, the focus is placed on sequential learning, and existing algorithms are heavily impacted by accidental clicks, which ultimately limits an item’s coverage. In this work, we propose a two-stage approach to boosting item’s coverage in session-based recommendations. First, we train a skip-gram model with negative sampling to generate candidate items that co-occur with a given query set. We then apply weighting to mitigate the effects of accidental clicks during a session. Next, we use a multi-armed bandit approach to boost recommendation coverage by balancing the exploration-exploitation trade-off. Experiments with three real-world datasets show that our model’s performance is comparable to existing state-of-the-art methods and outperforms them in recommendation coverage.
更多查看译文
关键词
item coverage,session-based
AI 理解论文
溯源树
样例
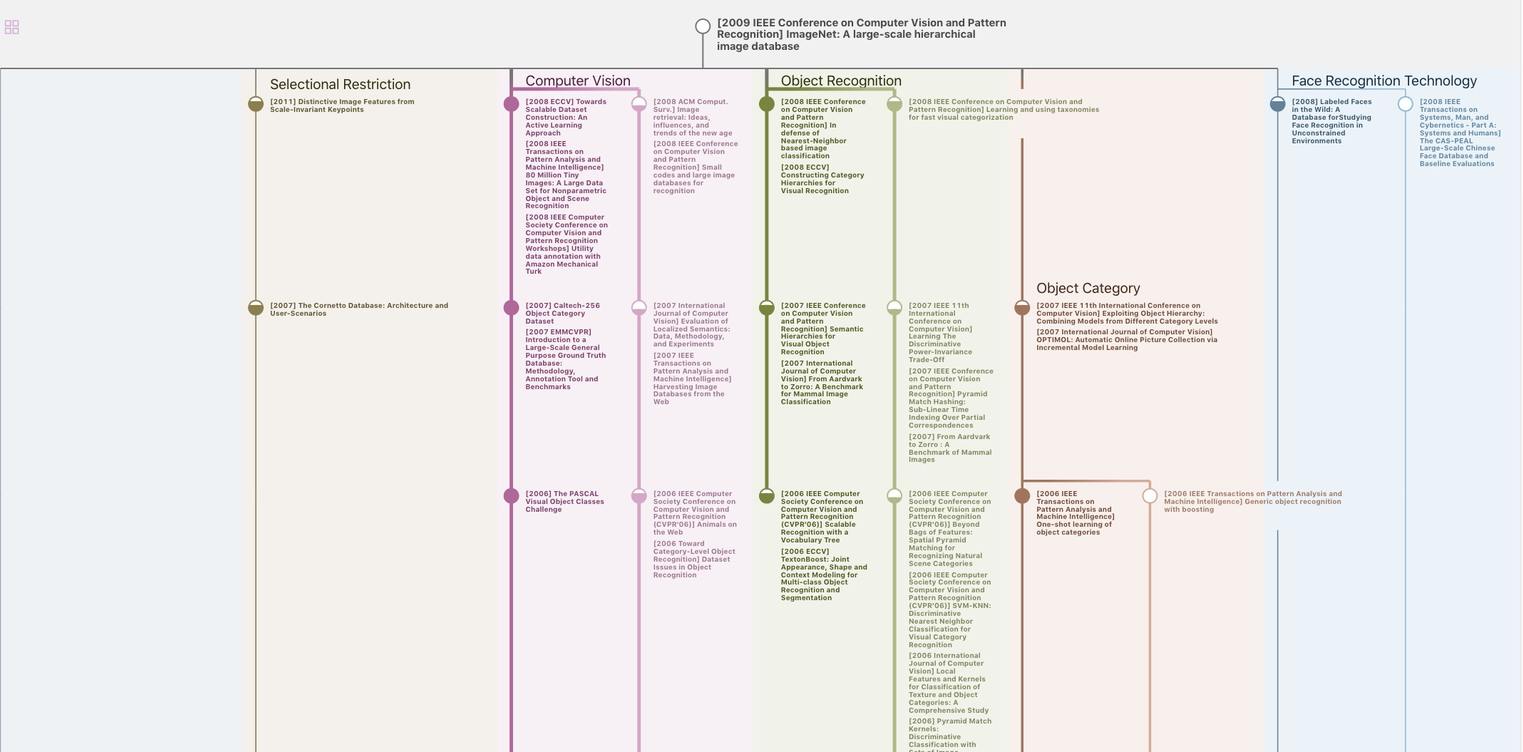
生成溯源树,研究论文发展脉络
Chat Paper
正在生成论文摘要