A System For Hybrid 4DVar-EnKF Data Assimilation Based On Deep Learning.
SMC(2022)
摘要
The accuracy of the initial field is crucial to the forecast results of numerical weather prediction (NWP). Data assimilation (DA) is a method to provide the initial field to the NWP. Currently, the hybrid 4DVar-EnKF DA method is the primary DA method used by operational NWP centres. The technique requires the derivation of the tangent linear and adjoint models for the nonlinear model, but it’s challenging to get the tangent linear and the adjoint models. Furthermore, this method usually adopts empirical coefficients to combine the four-dimensional variational assimilation (4DVar) and the ensemble Kalman filter (EnKF), which reduces the accuracy of assimilation results. This paper builds a hybrid DA system based on a deep learning model (DL-HDA) in response to the above problems. First, we establish a forecast model based on the bilinear neural network (BNN) and use the tangent linear and adjoint models of the BNN for the 4DVar. Then, we utilize the ResNet model to combine the analysis of the 4DVar and the EnKF. The experiments are carried out on the Lorenz-96 model, and then the DL-HDA is compared with the traditional method. The experimental results show that the DL-HDA can improve the precision of assimilation results and decrease the system’s running time.
更多查看译文
关键词
deep learning,numerical weather prediction,data assimilation,tangent linear and adjoint models
AI 理解论文
溯源树
样例
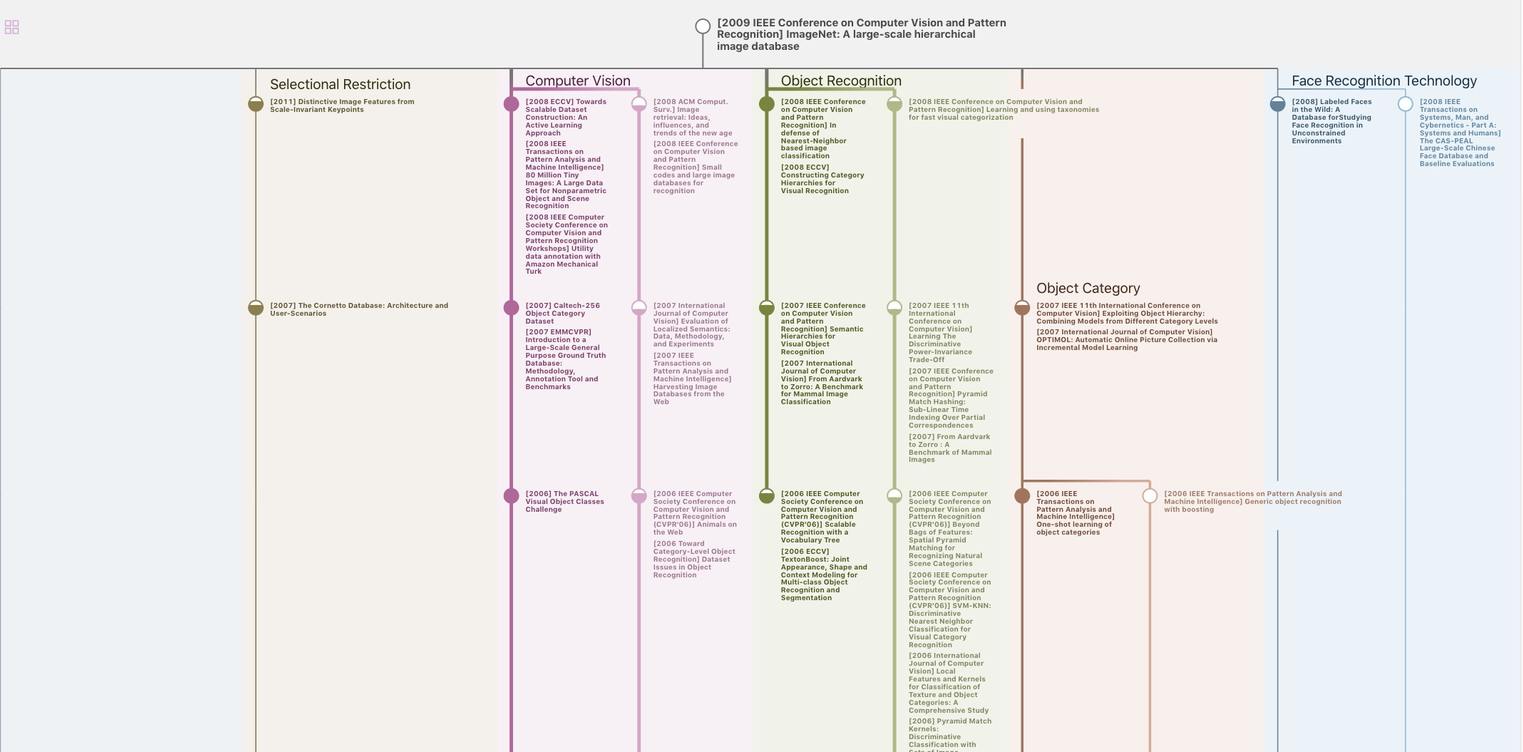
生成溯源树,研究论文发展脉络
Chat Paper
正在生成论文摘要