A study on skeleton-based action recognition and its application to physical exercise recognition.
SoICT(2022)
Abstract
In recent years, human action recognition (HAR) has been an attractive research topic in computer vision since HAR has been widely applied in various fields, such as gaming, healthcare, surveillance, and human-machine interaction. In this paper, we present a feasible solution to develop a real-time HAR application that helps one monitor physical exercises. As an end-to-end solution, the proposed framework consists of techniques that recognize the physical exercises, spot each in real-time and assess the quality of the practicing users. Firstly, we define nine common physical exercises, such as arm circles, squats, jumping jacks, etc. We then construct a dataset named COMVIS-FITNESS consisting of the physical exercises dataset of nine subjects. We argue the advantages of two different deep neural networks for HAR to recognize the exercises. One is a compact HAR neuronal network named DD-Net, and another is a high-performance graph convolutional neural network called FF-AAGCN. For spotting the exercises from an image sequence, a sliding window method is combined with these two networks to make a real-time classification. A technique to evaluate the workout of the practicing user is proposed. Experimental results on COMVIS-FITNESS show that deep neural networks can recognize all exercises in the datasets with high accuracy. The accuracies and F1-scores of DD-Net are 99.24% and 99.23%, while those obtained by FF-AAGCN are 98.48% and 98.32%, respectively. The completed pipeline of the proposed method is integrated in an application. The application works in real-time on edge device as Jetson Xavier AGX. The proposed techniques and dataset are made publicly available and can be downloaded from https://bit.ly/3F9J1qb.
MoreTranslated text
AI Read Science
Must-Reading Tree
Example
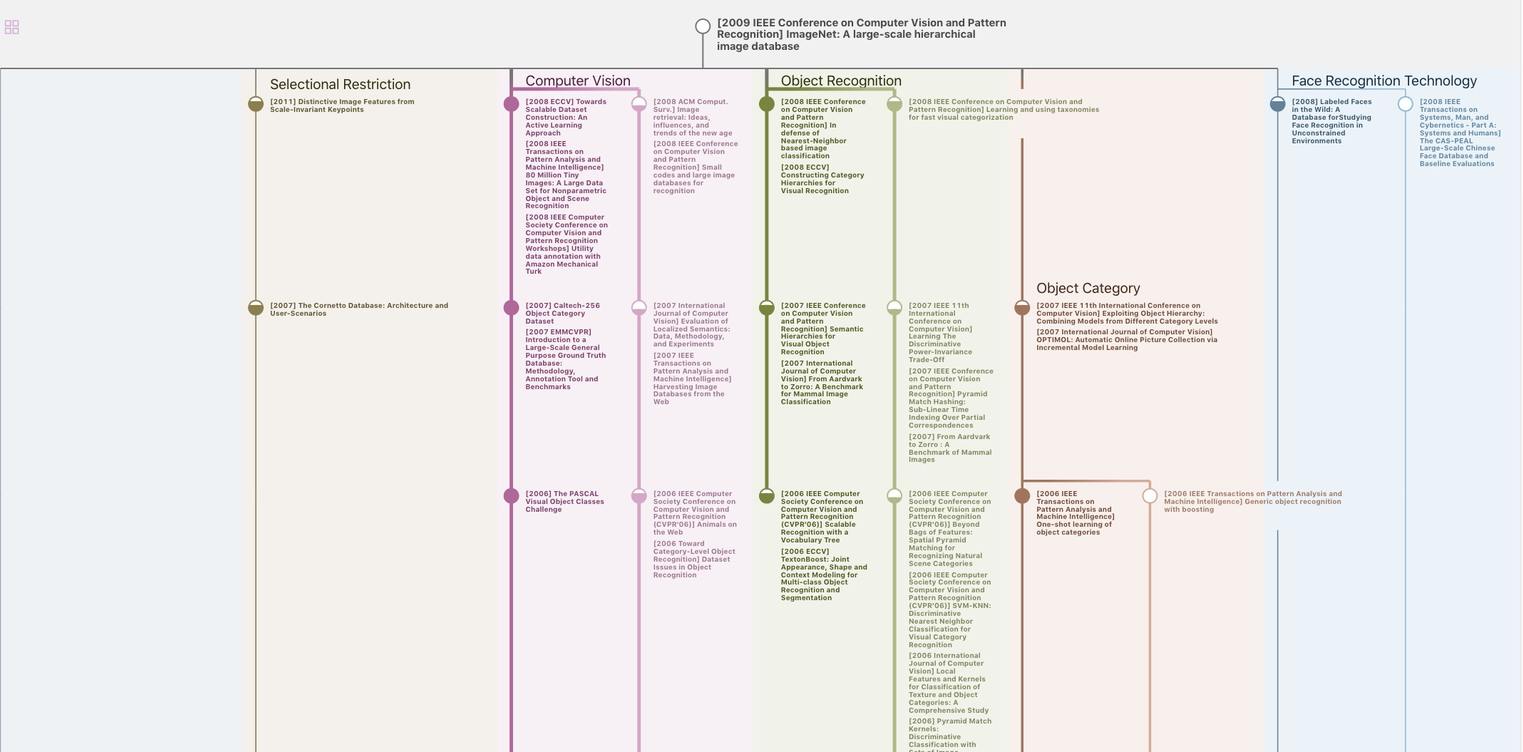
Generate MRT to find the research sequence of this paper
Chat Paper
Summary is being generated by the instructions you defined