Comparing Unsupervised Detection Algorithms for Audio Adversarial Examples.
SPECOM(2022)
摘要
Recent works on automatic speech recognition (ASR) systems have shown that the underlying neural networks are vulnerable to so-called adversarial examples. In order to avoid these attacks, different defense mechanisms have been proposed. Most defense mechanisms discussed so far are based on supervised learning, which requires a lot of resources. In this research, we present and compare various unsupervised learning methods for the detection of audio adversarial examples (including autoencoder, VAE, OCSVM, and isolation forest), requiring no adversarial examples in the training data. Our experimental results show that some of the considered methods successfully defend against a simple adversarial attack, e.g., with isolation forest. Even in a more elaborate attack scenario that considers human psychoacoustics, we still achieve a high detection rate with the cost of slightly increased false positive rate, e.g., with an autoencoder. We expect our detailed analysis to be a helpful baseline for further research in the area of defense methods against audio adversarial examples.
更多查看译文
关键词
Speech recognition,Adversarial examples,Adversarial detection
AI 理解论文
溯源树
样例
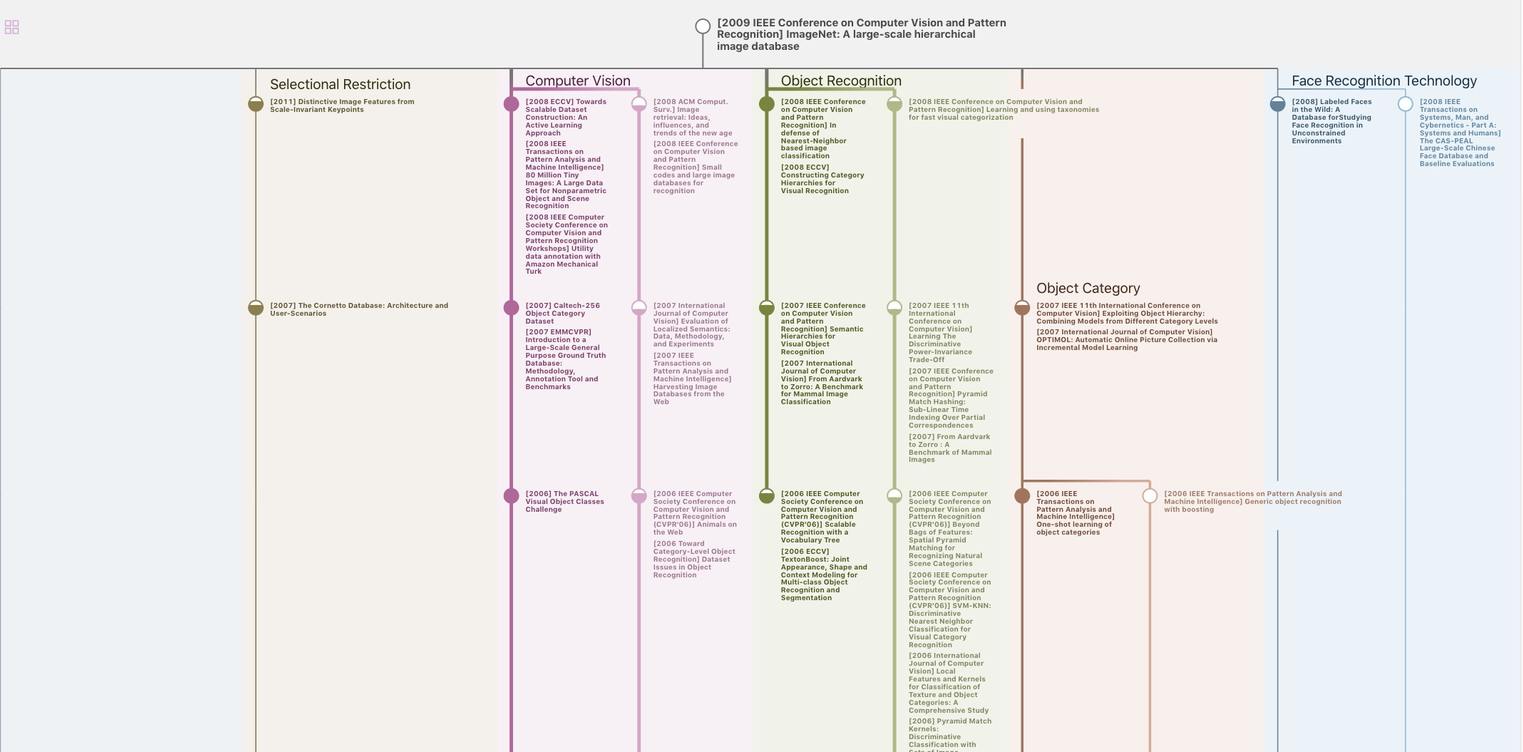
生成溯源树,研究论文发展脉络
Chat Paper
正在生成论文摘要