Friction-Adaptive Stochastic Predictive Control for Trajectory Tracking of Autonomous Vehicles.
ACC(2022)
摘要
This paper addresses the trajectory-tracking problem under uncertain road-surface conditions for autonomous vehicles. We develop a stochastic nonlinear model-predictive controller (SNMPC) that learns the tire-road friction relationship online using standard automotive-grade sensors. We learn the tire-friction function using a Bayesian approach, where the friction curve is modeled as a Gaussian process. The estimator outputs the estimate of the tire-friction model as well as the uncertainty function of the estimate, which expresses the confidence in the model for different driving regimes. The SNMPC exploits the uncertainty estimate in its prediction model to take proper action. We validate the approach using the high-fidelity vehicle simulator CarSim and compare against various nominal NMPC approaches. The results indicate more than six times better performance for the proposed adaptive SNMPC in closed-loop cost over the simulation time.
更多查看译文
关键词
nominal NMPC approaches,adaptive SNMPC,friction-adaptive stochastic predictive control,autonomous vehicles,trajectory-tracking problem,uncertain road-surface conditions,stochastic nonlinear model-predictive controller,automotive-grade sensors,tire-friction function,Bayesian approach,friction curve,Gaussian process,tire-friction model,uncertainty function,driving regimes,uncertainty estimate,prediction model,high-fidelity vehicle simulator CarSim,tire-road friction relationship
AI 理解论文
溯源树
样例
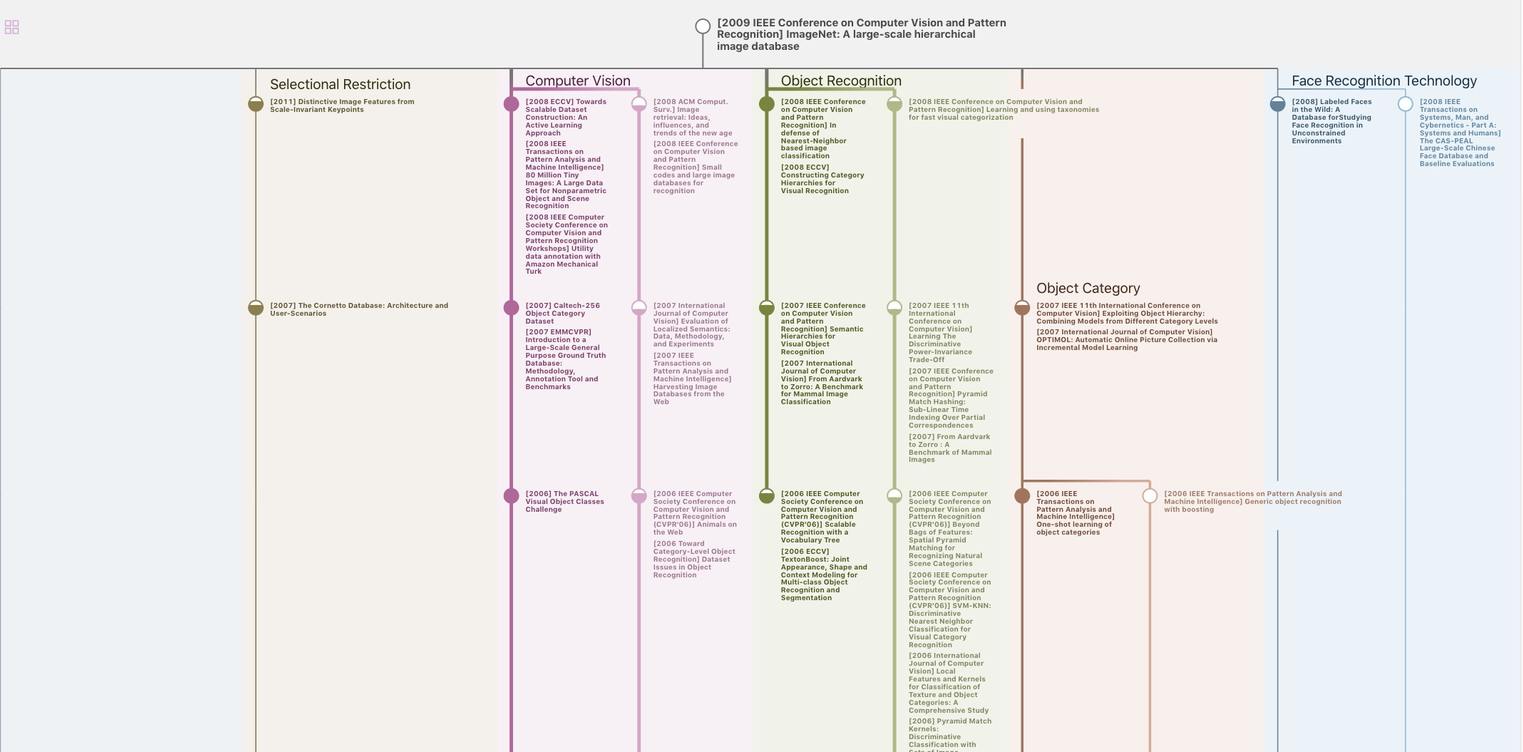
生成溯源树,研究论文发展脉络
Chat Paper
正在生成论文摘要