Optimal Moving Average Estimation of Noisy Random Walks using Allan Variance-informed Window Length.
ACC(2022)
摘要
Moving averages are widely used to estimate time-varying parameters, especially when the underlying dynamic model is unknown or uncertain. However, the selection of the optimal window length over which to evaluate the moving averages remains an unresolved issue in the field. In this paper, we demonstrate the use of Allan variance to identify the characteristic timescales of a noisy random walk from historical measurements. Further, we provide a closed-form, analytical result to show that the Allan variance-informed averaging window length is indeed the optimal averaging window length in the context of moving average estimation of noisy random walks. We complement the analytical proof with numerical results that support the solution, which is also reflected in the authors' related works. This systematic methodology for selecting the optimal averaging window length using Allan variance is expected to widely benefit practitioners in a diverse array of fields that utilize the moving average estimation technique for noisy random walk signals.
更多查看译文
关键词
noisy random walks,estimation,window length,variance-informed
AI 理解论文
溯源树
样例
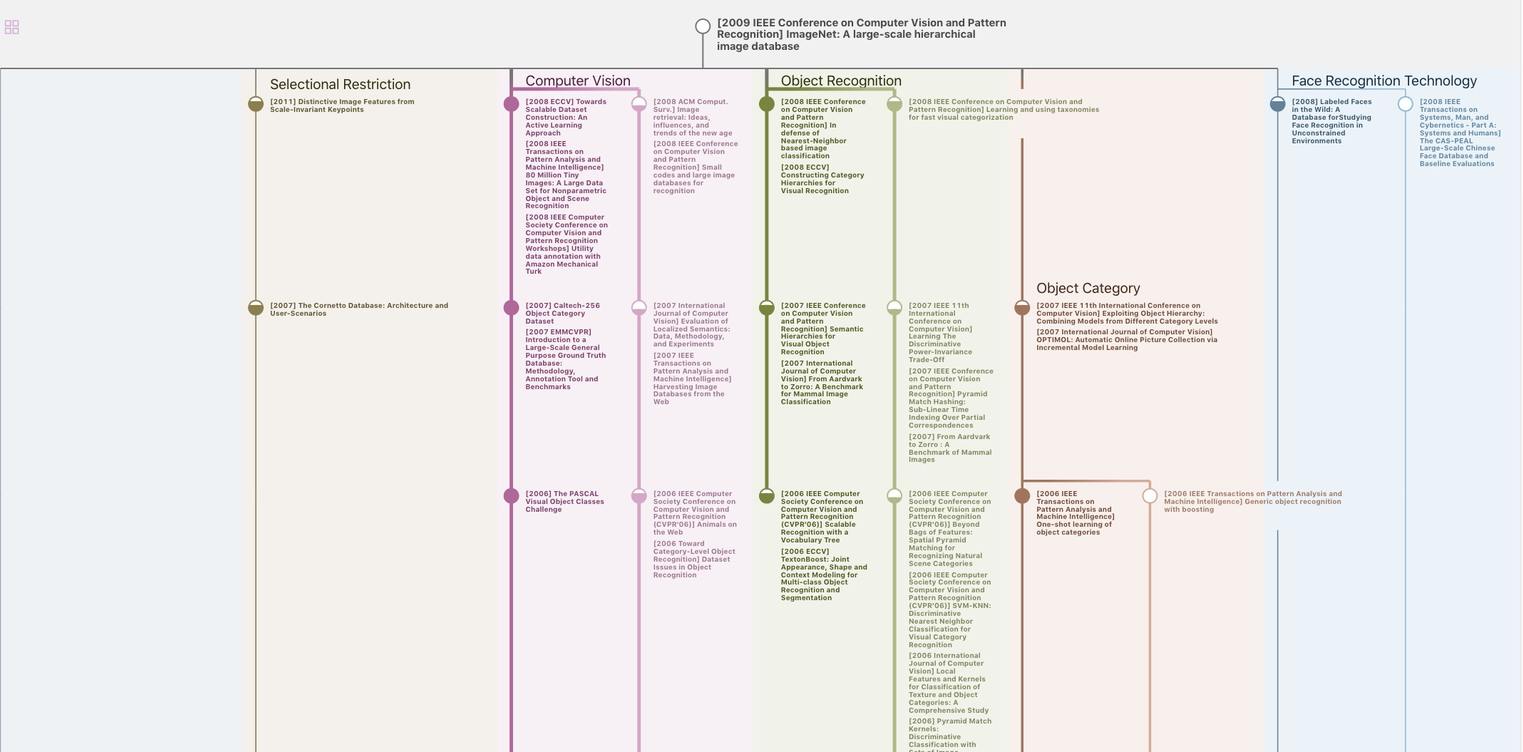
生成溯源树,研究论文发展脉络
Chat Paper
正在生成论文摘要