Encoder-Decoder Architectures for Silent Speech Recognition based on High-density Surface Electromyogram.
ICARM(2022)
摘要
Silent speech based on surface electromyogram(sEMG) has become an important interaction method. However, existing systems are highly dependent on time-alignment data, which is not conducive to the wide application of silent speech recognition (SSR) systems. In this study, we propose a convolutional Long Short-Term Memory-based encoder-decoder architecture to characterize and decode silent speech without time-alignment training data. The encoder can map the sEMG feature maps into a fixed-length feature vector, and the decoder can decode this vector back to the target sequence. To verify the effectiveness of the proposed method, the experimental data of 33 utterances from 7 subjects were collected from high-density electrode arrays with 64 channels from face and neck muscles. The performance of the proposed method was superior to the benchmark LSTM-based encoder-decoder architecture both on word error rate and utterance classification accuracy. These findings of this work indicate that the proposed method has the potential to achieve a rapid establishment of SSR system.
更多查看译文
关键词
benchmark LSTM-based encoder-decoder architecture,convolutional Long Short-Term Memory-based encoder-decoder architecture,encoder can,encoder-decoder architectures,fixed-length feature vector,high-density electrode arrays,high-density surface electromyogram,important interaction method,sEMG feature maps,silent speech recognition systems,SSR system,time-alignment data,time-alignment training data
AI 理解论文
溯源树
样例
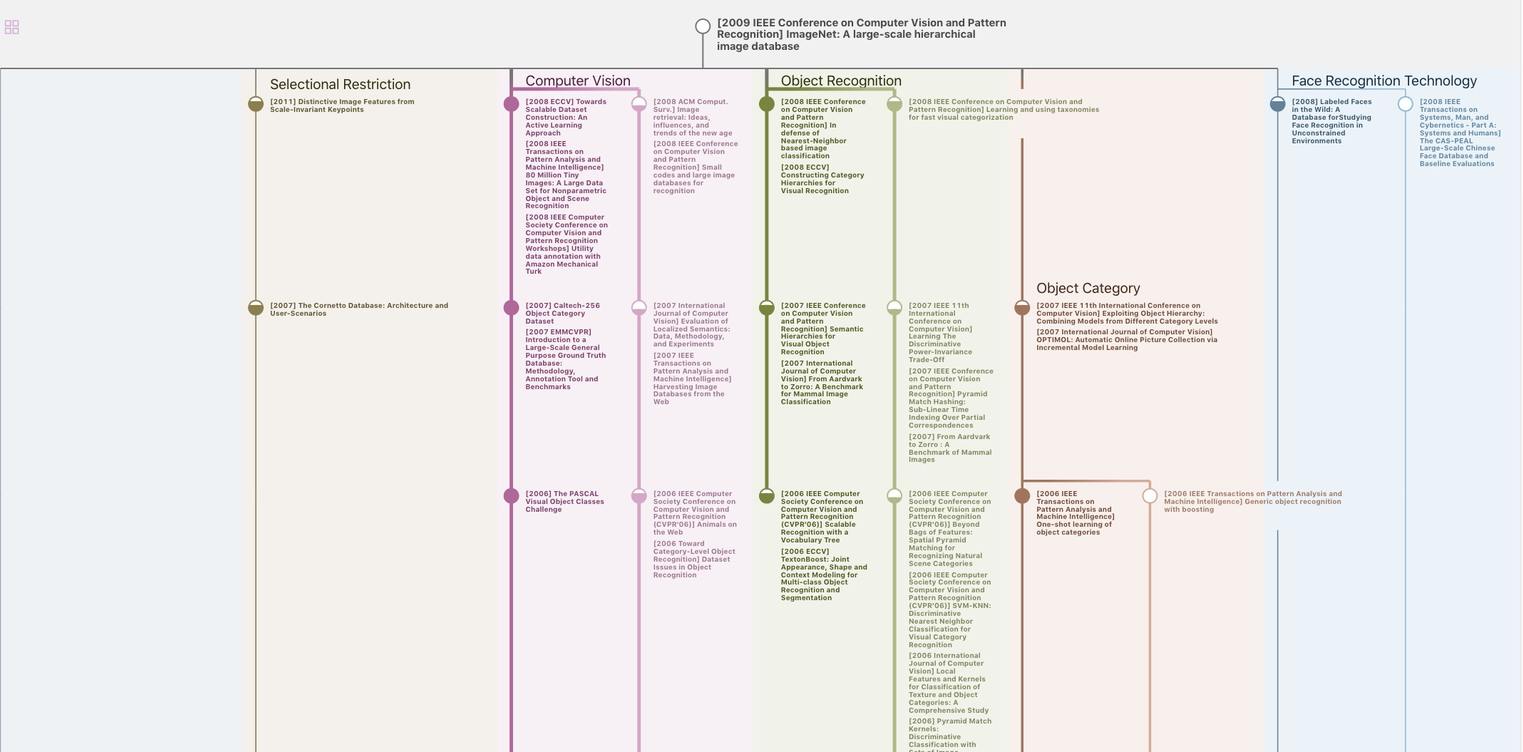
生成溯源树,研究论文发展脉络
Chat Paper
正在生成论文摘要