Comparative Study of Convolutional Neural Network in Multi-category Aliasing Hardware Recognition.
ICARM(2022)
Abstract
In the actual factory site, multi-category metal workpieces of different sizes are always stacked together, and the lighting conditions on site are always complicated, which makes the existing vision detection algorithms unable to work properly. To propose an algorithm suitable for this occasion, five representative convolutional neural networks, Faster R-CNN, SSD, EfficientDet, YOLOv4, and YOLOX, are studied in this paper for recognizing three typical metal stamping workpieces. And then, their performance is comparatively studied from five indicators: FPS, AP, mAP, Precision, and Recall. According to the experimental results, YOLOv4, YOLOX, and SSD are more suitable for dealing with multi-category stacked metal workpieces recognition tasks. At last, some suggestions are suggested to have an improvement in the performance of the existing algorithm as well as to design a novel algorithm. The study work can be used as a reference for the research of multi-category metal workpieces recognition and localization algorithms in complex aliasing scenes.
MoreTranslated text
Key words
convolutional neural network,recognition,hardware,multi-category
AI Read Science
Must-Reading Tree
Example
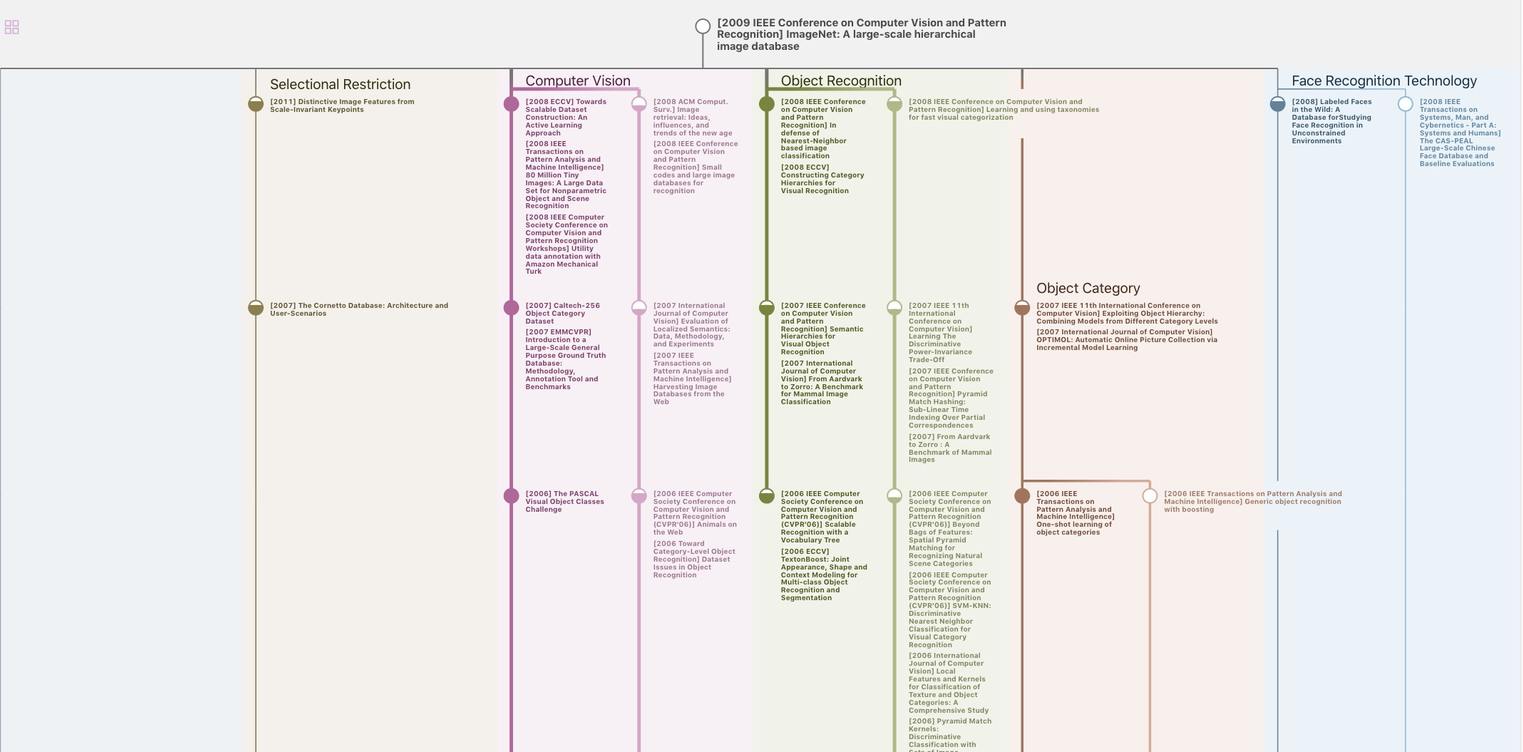
Generate MRT to find the research sequence of this paper
Chat Paper
Summary is being generated by the instructions you defined