DDFM: A Novel Perspective on Urban Travel Demand Forecasting Based on the Ensemble Empirical Mode Decomposition and Deep Learning.
ICBDT(2022)
摘要
Urban travel demand forecasting is significant in improving traffic conditions and traffic management. Although current research focuses on urban travel demand prediction using various deep learning models, there is high-frequency noise and complex nonlinear fluctuation patterns in urban travel demand time series, and these deep learning models do not have an excellent ability to fit the various patterns and certain local extremes of urban travel demand. Therefore, a Deep Decomposition Forecasting Model (DDFM) is introduced for modeling and predicting non-stationary, non-linear urban travel demand data. The DDFM consists of two main components, which are the Ensemble Empirical Mode Decomposition (EEMD) for decomposing urban travel demand data and the Long Short-term Memory (LSTM) network. EEMD can reduce the LSTM's difficulty in fitting the non-linear fluctuation pattern of the data. LSTM can model long-term time-series data. Experiments show that the performance of DDFM is excellent. The Mean Absolute Error (MAE) and Root Mean Square Error (RMSE) of DDFM in predicting urban travel demand are significantly reduced compared to the baseline. Overall, DDFM can do a good job of predicting urban travel demand with complex nonlinear patterns.
更多查看译文
AI 理解论文
溯源树
样例
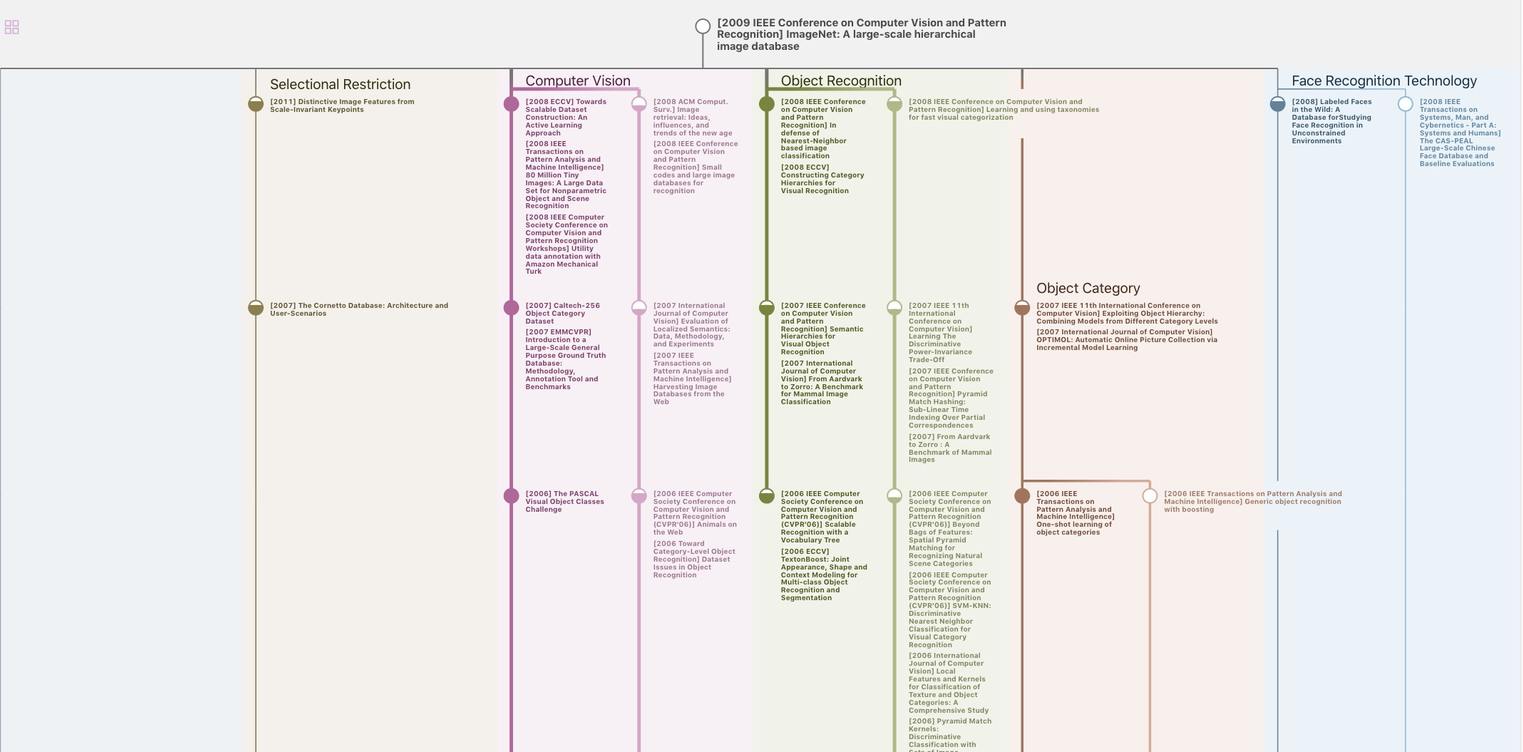
生成溯源树,研究论文发展脉络
Chat Paper
正在生成论文摘要