Design and Implementation of Web Page Classification Method Based on XLNet Fusing Hierarchical Attention and CNN.
ICBDT(2022)
摘要
The explosive growth in the number of web pages makes web page classification crucial for web information retrieval, content filtering, and topic crawling, etc. To address the problems that traditional web page classification models have incomplete web text feature extraction and difficulty in capturing and utilizing both global semantic features and local semantic features, we propose a web page classification model XLNet-HAC based on the pre-trained model XLNet fusing Hierarchical Attention and CNN. For web page text extracted using URLs, the pre-trained model XLNet is used as an embedding layer to obtain a feature matrix representation with rich contextual relationships, using word attention and sentence attention mechanisms of Hierarchical Attention to capture the words and sentences that highly contribute to the classification, thus generates a global feature representation of the web page text, and the multi-channel CNN with convolutional kernels of different sizes is used to extract local features at multiple granularities of web page text. Finally, the outputs of Hierarchical Attention and CNN are applied to the softmax classifier respectively, and the classification results are fused to obtain the final classification result. The comparison experimental results on THUCNews dataset and DMOZ dataset show that the XLNet-HAC model proposed in this study outperforms the other comparison models in terms of classification accuracy and F1-score.
更多查看译文
AI 理解论文
溯源树
样例
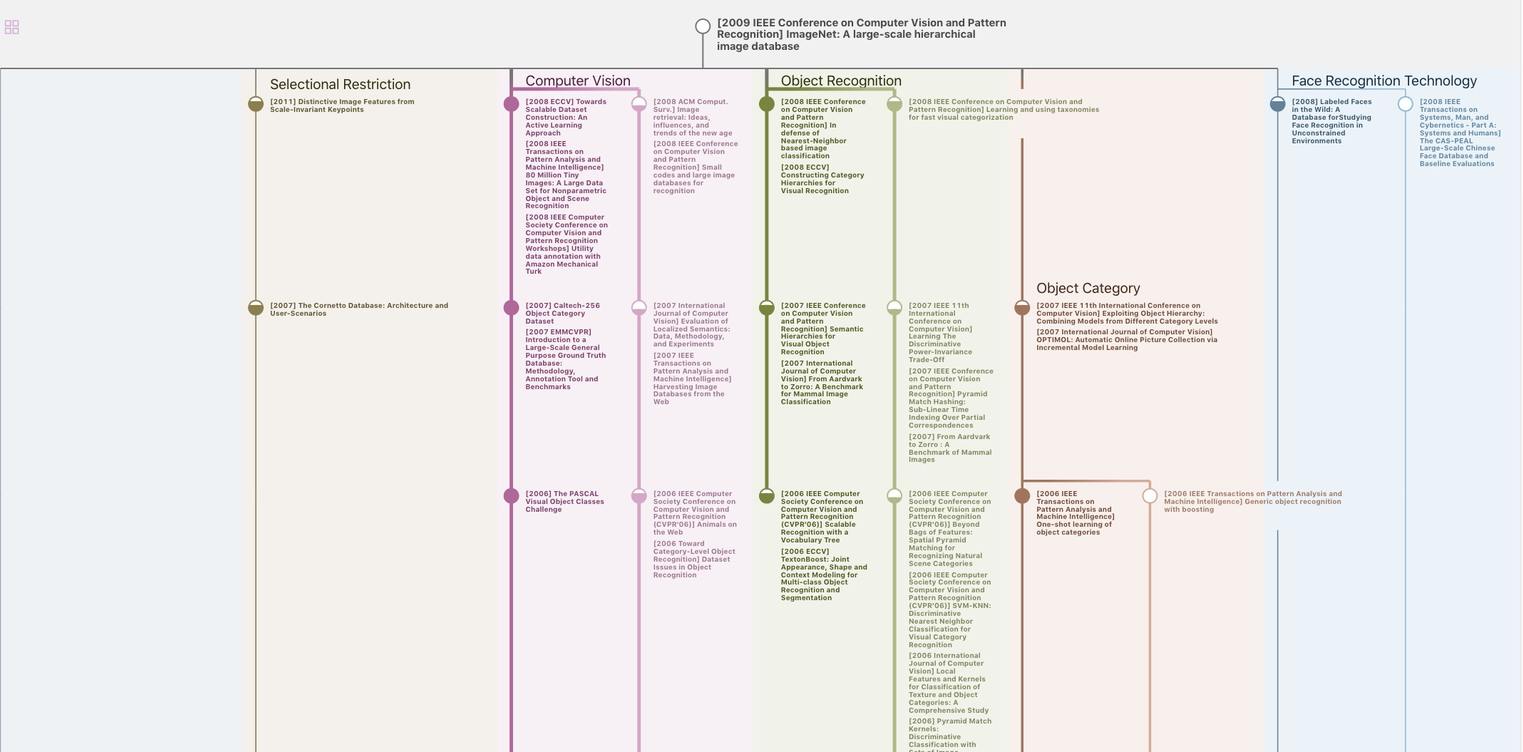
生成溯源树,研究论文发展脉络
Chat Paper
正在生成论文摘要