Simulating Scenarios to Evaluate Data Filtering Techniques for Mobile Users.
MoMM(2022)
摘要
Citizens are nowadays being flooded with huge amounts of information, which will keep growing as the physical spaces become more intelligent, with the proliferation of sensors (e.g., pollution sensors, traffic sensors, etc.), mobile apps, and information services of different types (e.g., malls providing offers and other kinds of information to nearby customers). To actually become resilient modern citizens, people need to be able to handle all this highly-dynamic information and act upon it by taking suitable decisions. In this context, the development of suitable data management techniques to help citizens in their daily life plays a major role. Motivated by this, we focus on the design of novel data management techniques for mobile users (pedestrians) and for drivers, which are two key areas in the daily life of citizens. More specifically, we consider the problem of recommending relevant items to pedestrians (e.g., tourists) and the challenges of drivers when they try to find an available parking space. As evaluating data management strategies in a real environment in a large-scale is very challenging, in this paper we propose suitable simulation approaches that facilitate the evaluation task. Through simulations, we obtain some initial experimental results that show the additional difficulties that appear when we want to satisfy additional constraints such as the desire to minimize the risk of virus spread in a COVID-19 scenario.
更多查看译文
关键词
Data management,Mobile computing,Recommender systems,Parking spaces
AI 理解论文
溯源树
样例
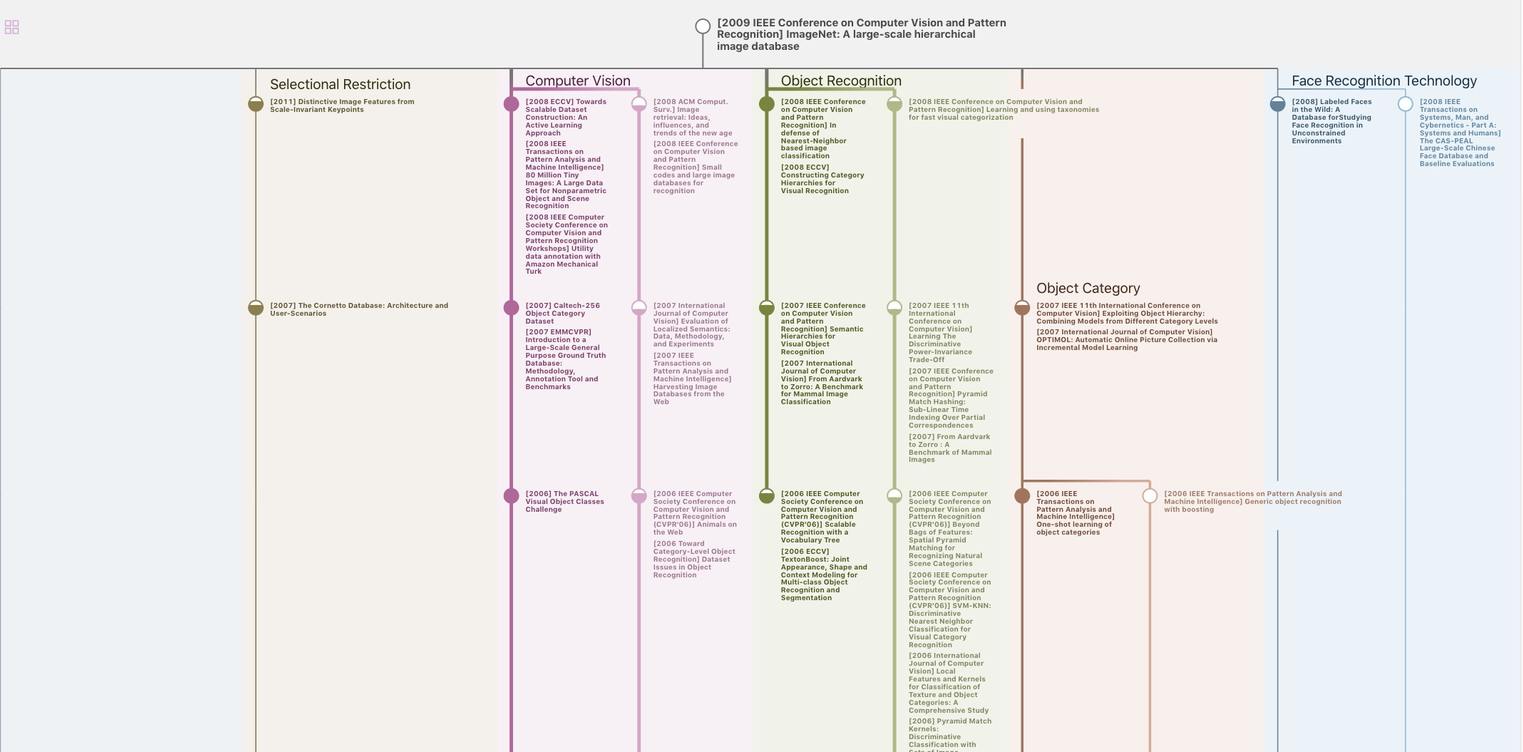
生成溯源树,研究论文发展脉络
Chat Paper
正在生成论文摘要