Practical Sketching Algorithms for Low-Rank Tucker Approximation of Large Tensors
Journal of Scientific Computing(2023)
Abstract
Low-rank approximation of tensors has been widely used in high-dimensional data analysis. It usually involves singular value decomposition (SVD) of large-scale matrices with high computational complexity. Sketching is an effective data compression and dimensionality reduction technique applied to the low-rank approximation of large matrices. This paper presents two practical randomized algorithms for low-rank Tucker approximation of large tensors based on sketching and power scheme, with a rigorous error-bound analysis. Numerical experiments on synthetic and real-world tensor data demonstrate the competitive performance of the proposed algorithms.
MoreTranslated text
Key words
Tensor sketching,Randomized algorithm,Tucker decomposition,Subspace power iteration,High-dimensional data
AI Read Science
Must-Reading Tree
Example
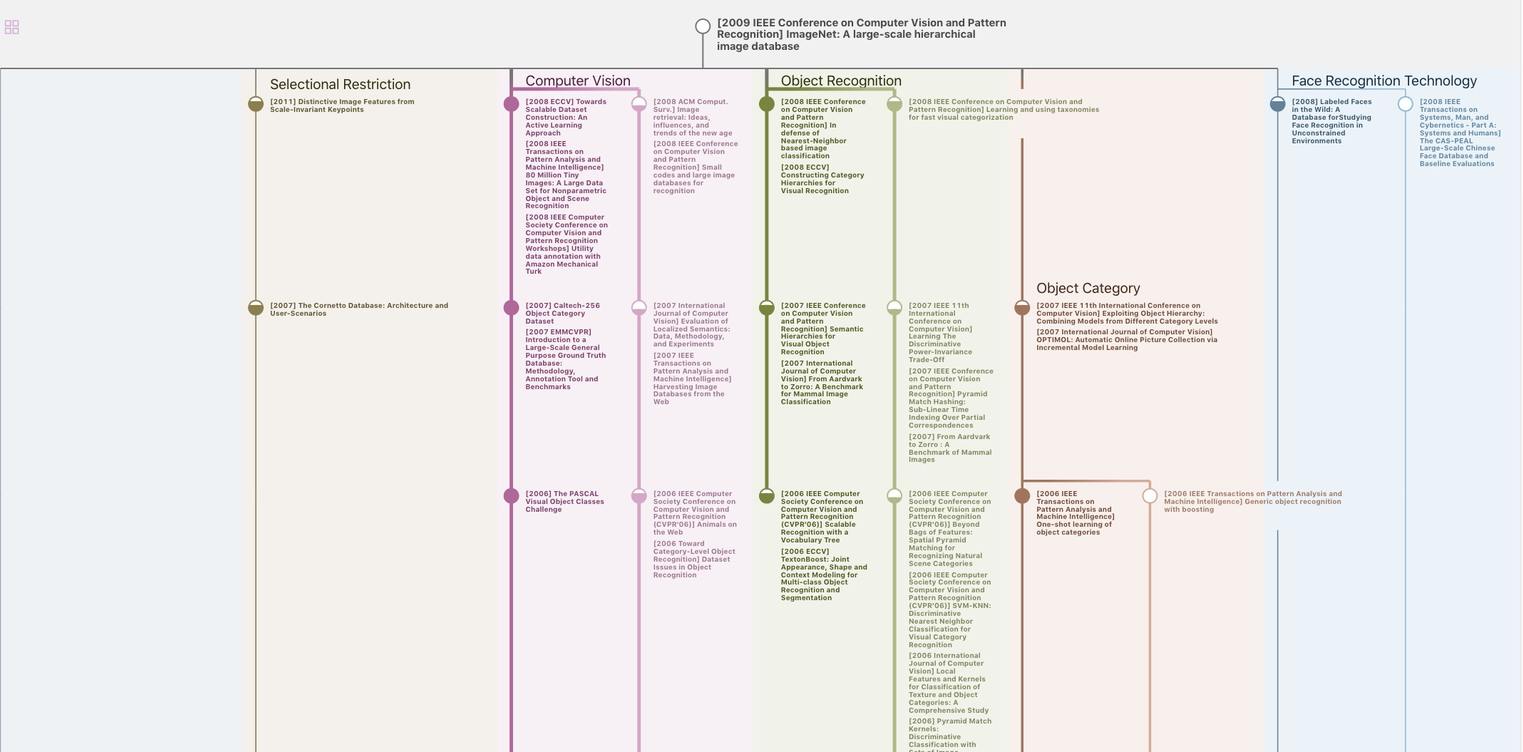
Generate MRT to find the research sequence of this paper
Chat Paper
Summary is being generated by the instructions you defined