An adaptive offloading framework for license plate detection in collaborative edge and cloud computing.
Mathematical biosciences and engineering : MBE(2023)
摘要
With the explosive growth of edge computing, huge amounts of data are being generated in billions of edge devices. It is really difficult to balance detection efficiency and detection accuracy at the same time for object detection on multiple edge devices. However, there are few studies to investigate and improve the collaboration between cloud computing and edge computing considering realistic challenges, such as limited computation capacities, network congestion and long latency. To tackle these challenges, we propose a new multi-model license plate detection hybrid methodology with the tradeoff between efficiency and accuracy to process the tasks of license plate detection at the edge nodes and the cloud server. We also design a new probability-based offloading initialization algorithm that not only obtains reasonable initial solutions but also facilitates the accuracy of license plate detection. In addition, we introduce an adaptive offloading framework by gravitational genetic searching algorithm (GGSA), which can comprehensively consider influential factors such as license plate detection time, queuing time, energy consumption, image quality, and accuracy. GGSA is helpful for Quality-of-Service (QoS) enhancement. Extensive experiments show that our proposed GGSA offloading framework exhibits good performance in collaborative edge and cloud computing of license plate detection compared with other methods. It demonstrate that when compared with traditional all tasks are executed on the cloud server (AC), the offloading effect of GGSA can be improved by 50.31%. Besides, the offloading framework has strong portability when making real-time offloading decisions.
更多查看译文
关键词
edge computing , internet of things , resource allocation , task offloading
AI 理解论文
溯源树
样例
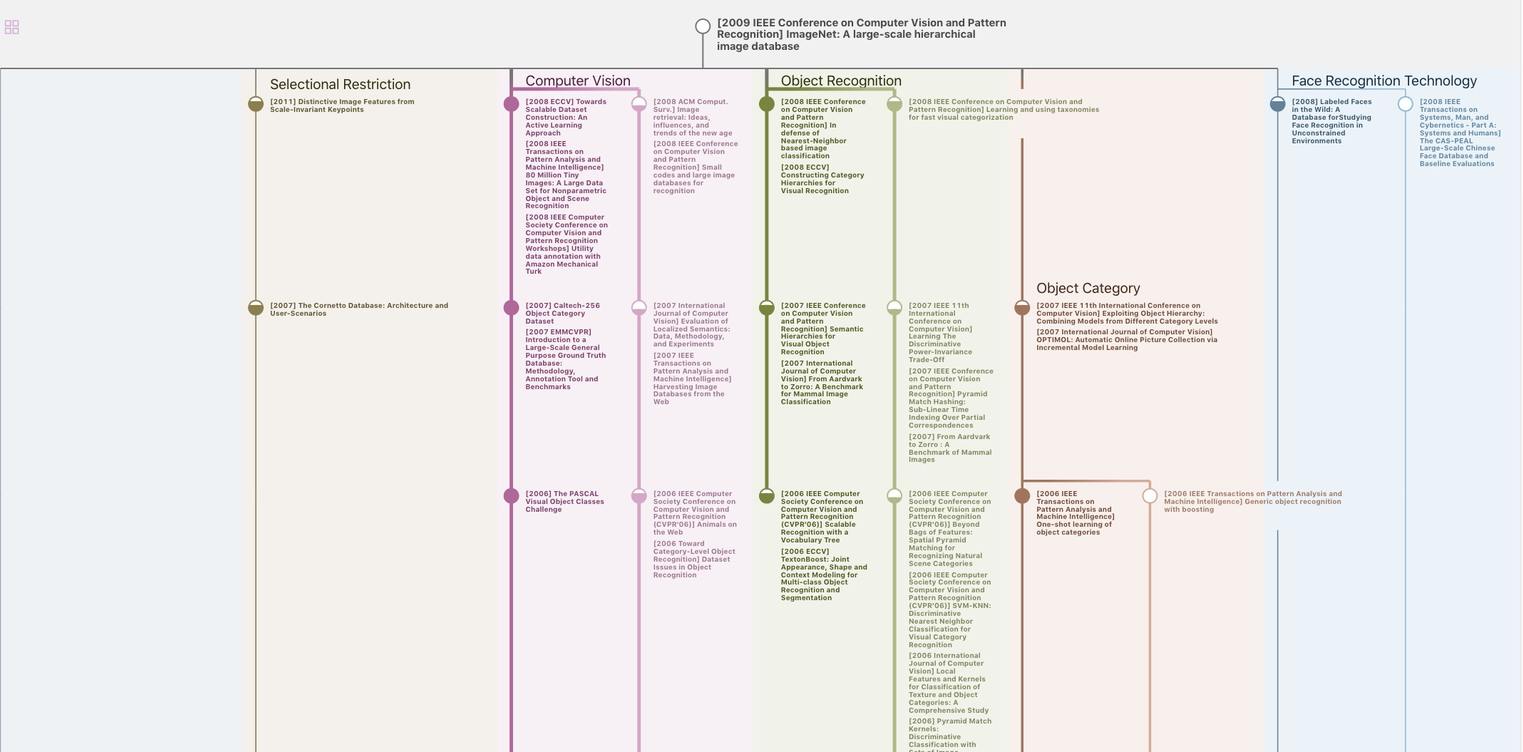
生成溯源树,研究论文发展脉络
Chat Paper
正在生成论文摘要