Ensemble Processing and Synthetic Image Generation for Abnormally Shaped Nuclei Segmentation
biorxiv(2023)
摘要
Abnormalities in biological cell nuclei morphology are correlated with cell cycle stages, disease states, and various external stimuli. There have been many deep learning approaches that have described nuclei segmentation and analysis of nuclear morphology. One problem with many deep learning methods is acquiring large amounts of annotated nuclei data, which is generally expensive to obtain. In this paper, we propose a system to segment abnormally shaped nuclei with a limited amount of training data. We first generate specific shapes of synthetic nuclei groundtruth. We randomly sample these synthetic groundtruth images into training sets to train several Mask R-CNNs. We design an ensemble strategy to combine or fuse segmentation results from the Mask R-CNNs. We also design an oval nuclei removal by StarDist to reduce the false positives and improve the overall segmentation performance. Our experiments indicate that our method outperforms other methods in segmenting abnormally shaped nuclei.
### Competing Interest Statement
The authors have declared no competing interest.
更多查看译文
关键词
nuclei,segmentation,synthetic image generation
AI 理解论文
溯源树
样例
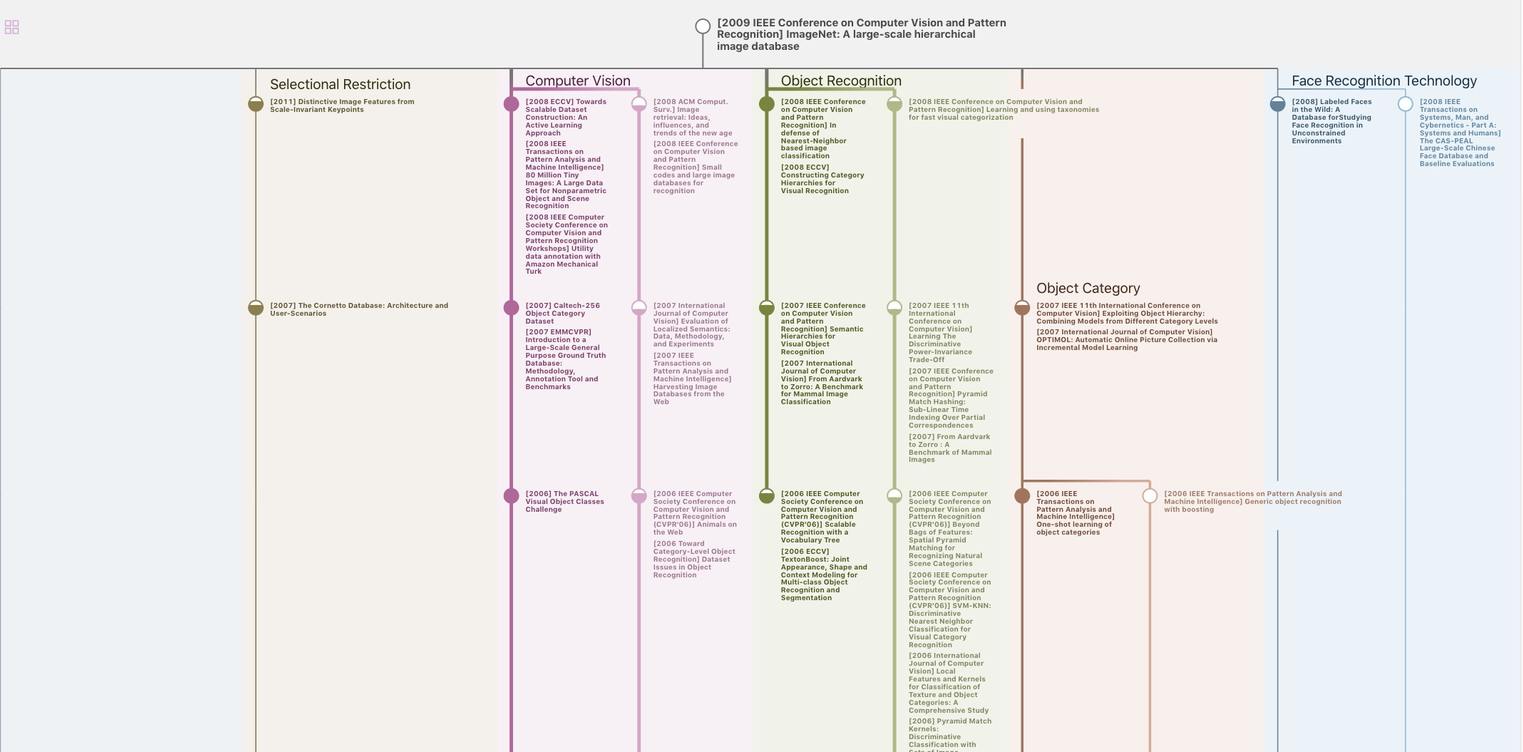
生成溯源树,研究论文发展脉络
Chat Paper
正在生成论文摘要