Classification of Chest X-Ray Images for COVID-19 Positive Patients Using Transfer Learning
Lecture Notes on Data Engineering and Communications Technologies Advances in Intelligent Systems, Computer Science and Digital Economics IV(2023)
摘要
The Coronavirus pandemic COVID-19 which has been declared as a pandemic by the World Health Organization has infected more than 212,165,567 and fatality figure of 4,436,957 as of 22nd August 2021. This infection develops into pneumonia which causes breathing problem; this can be detected using chest x-rays or CT scan. This work aims to produce an automated way of detecting the presence of COVID-19 infection using chest X-rays as a part of transfer learning strategy to extract numerical features out of an image using pre trained models as feature extractors. Then construct a secondary data set out of these features, and use these features which are simple numerical vectors represented in tabular form as an input to simple machine learning classifiers that work well with numerical data in tabular form such as SVM, KNN, Logistic regression and Naive Bayes. This work also aims to extract features using texture-based techniques such as GLCM and use the GLCM to obtain 2nd order statistical features and construct another secondary data set based on texture-based feature extraction techniques on images. These features are again fed into simple machine learning classifiers mentioned above. A comparison is done, between deep learning feature extraction strategies and texture-based feature extraction strategies and the results are compared and analyzed. Considering the deep learning strategies Mobile Net with SVM perform the best with 0.98 test accuracy, followed by logistic regression, KNN and Naive Bayes algorithm. With respect to GLCM feature extraction strategy, KNN with test accuracy with 0.96 performed the best, followed by logistic regression, SVM and naive Bayes. Overall performance wise deep learning strategies proved to be effective but in terms of calculation time and number of features, texture-based strategy of GLCM proved effective.
更多查看译文
关键词
transfer learning,classification,x-ray
AI 理解论文
溯源树
样例
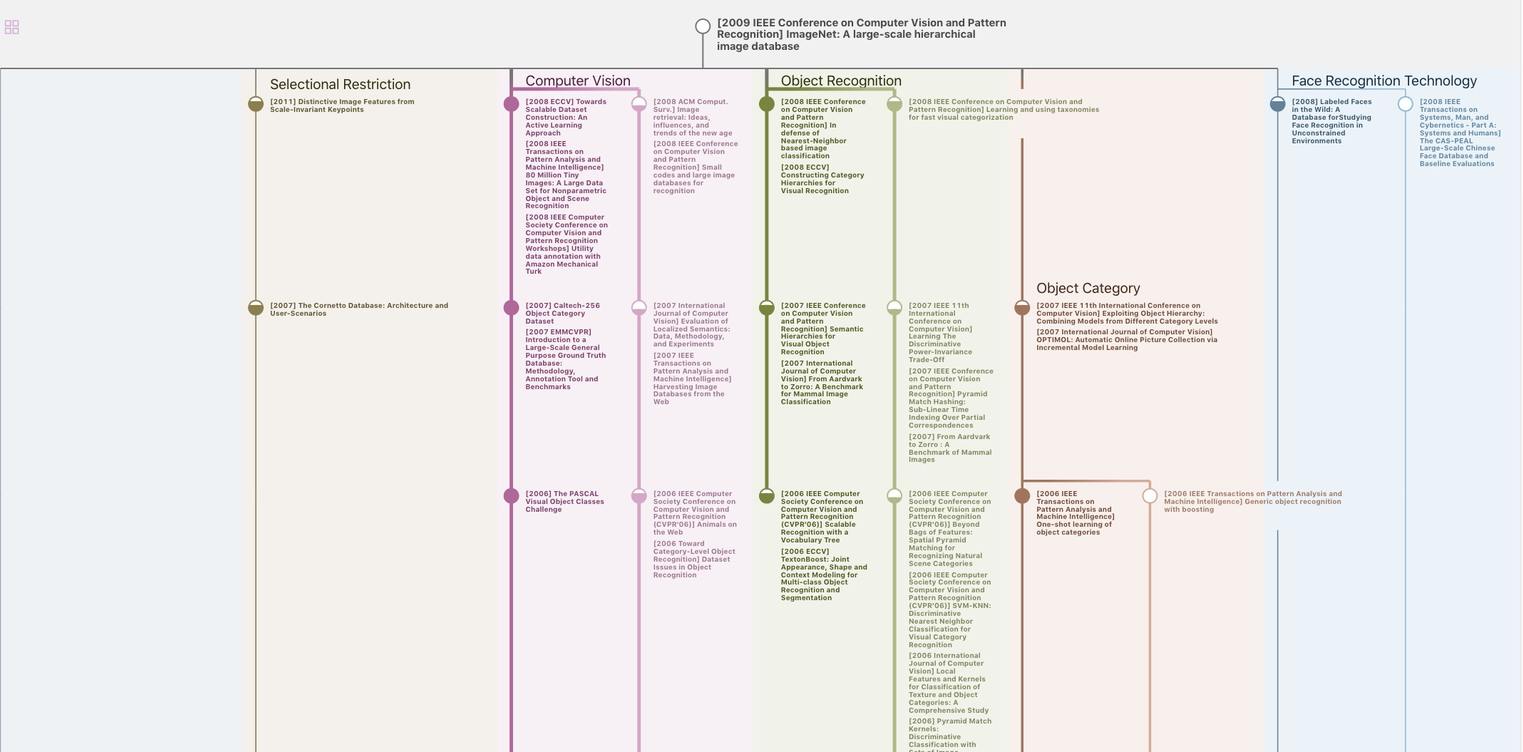
生成溯源树,研究论文发展脉络
Chat Paper
正在生成论文摘要