Deviation distance entropy: A method for quantifying the dynamic features of biomedical time series
Chaos, Solitons & Fractals(2023)
摘要
Physiological system time series (signals) usually follow a pattern of fluctuations over time. Mining the potential dynamic features of physiological system time series is the key to understanding changes in the state and behavior of physiological systems. In this paper, we propose a new method to measure the complexity of the dynamic features of physiological system time series, namely deviation distance entropy (DE). It achieves the modeling of dynamic features by considering the relationship between current and future segments of the time series and further quantifies their complexity. Through simulation and analysis, we show that DE enables accurate extraction of key features of the signal. Applying the DE method to real electrocardiogram (ECG) signals, we find that DE has a better ability to distinguish between signals from healthy individuals and atrial fibrillation (AF) patients than other methods for measuring sequence irregularities, such as approximate entropy, sample entropy and fuzzy entropy. Further, we propose the idea of "clarity"for the curve of dynamic features. Using "clarity", we can graphically grade patients with AF according to their ECG signals. According to our numerical analysis, deviation distances for patients with AF follow two different power laws. The magnitude of the difference between these two power laws is positively correlated with the severity of AF onset in the corresponding patients. An in-depth analysis of this phenomenon reveals that it is essentially the development of chaos in the corresponding system, while fluctuations in the corresponding trajectory periods of the mapped attractors can also be observed, which may explain how AF starts and develops. Our study provides a novel perspective for characterizing the time series dynamics of physiological systems.
更多查看译文
关键词
Time series,Dynamic features,Entropy
AI 理解论文
溯源树
样例
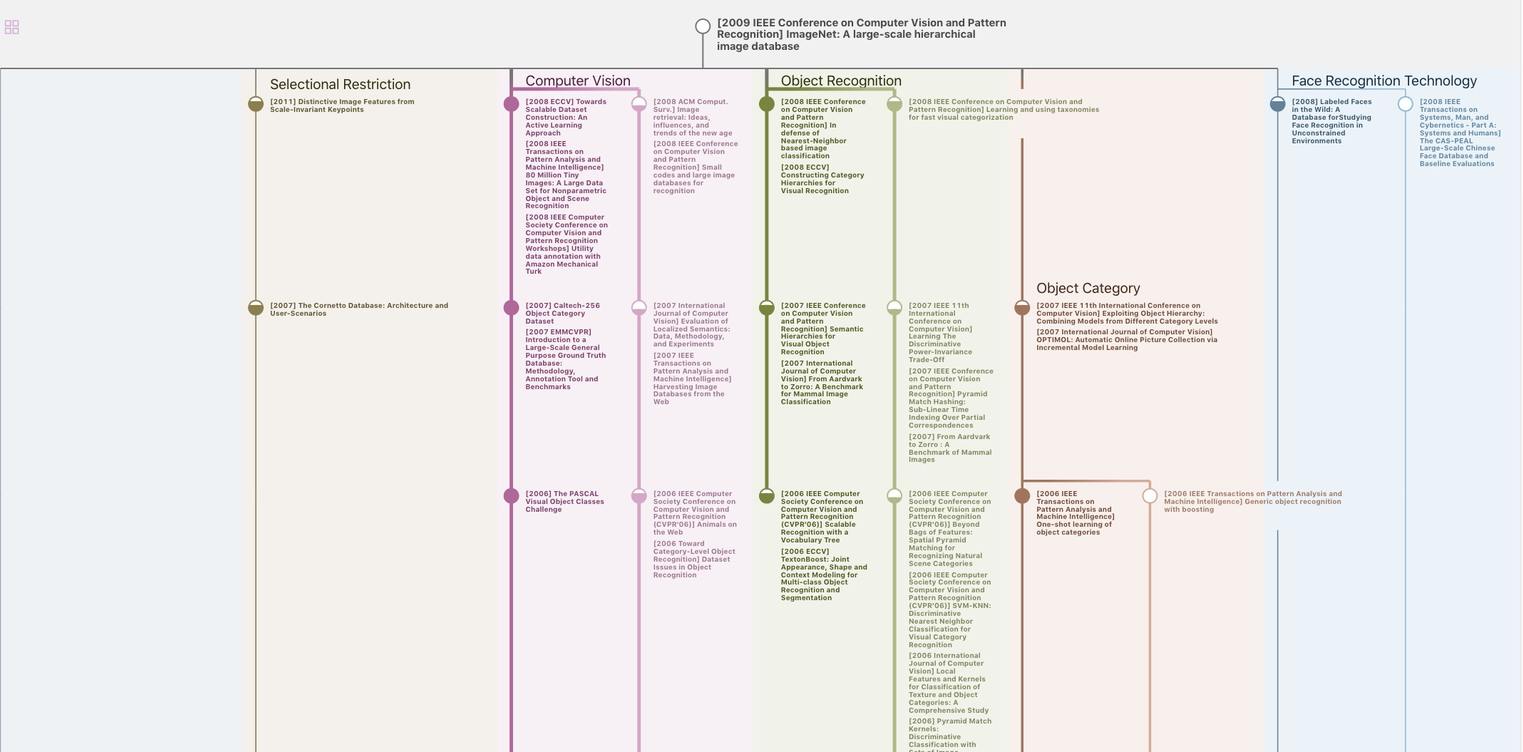
生成溯源树,研究论文发展脉络
Chat Paper
正在生成论文摘要