Assessment of the Atrial Fibrillation Burden in Holter ECG Recordings using Artificial Intelligence
SWISS MEDICAL WEEKLY(2023)
摘要
Abstract Funding Acknowledgements Type of funding sources: Foundation. Main funding source(s): Swiss National Science Foundation, Swiss Heart Foundation Background Emerging evidence indicates that a high atrial fibrillation (AF) burden is associated with adverse outcome. However, AF burden is not routinely measured in clinical practice. An artificial intelligence (AI)-based tool could facilitate the assessment of AF burden. Purpose We aimed to compare the assessment of AF burden performed manually by physicians with that measured by an AI-based tool. Methods We analysed 7-day Holter ECG recordings of AF patients included in a prospective multicentre cohort study. AF burden was defined as percentage of time in AF, and was assessed manually by physicians and by an AI-based tool. We evaluated the agreement between both techniques by means of Pearson`s correlation coefficient, linear regression model, and Bland-Altman plot. Results We assessed the AF burden in 100 Holter ECG recordings of 82 patients. We identified 53 Holter ECGs with 0% or 100% AF burden, where we found a 100% correlation. For the remaining 47 Holter ECGs with an AF burden between 0.01% and 81,53%, Pearson’s correlation coefficient was 0.998. The calibration intercept was -0.001 (95% CI -0.008; 0.006), and the calibration slope was 0.975 (95% CI 0.954; 0.995; multiple R2 0.995, residual standard error 0.017). Bland-Altman analysis resulted in a bias of -0.006 (95% limits of agreement -0.042 to 0.030). Conclusion The assessment of AF burden with an AI-based tool provides very similar results compared to manual assessment. An AI-based tool may therefore be an accurate and efficient option for the assessment of AF burden.
更多查看译文
关键词
holter ecg recordings,atrial fibrillation burden,atrial fibrillation,artificial intelligence
AI 理解论文
溯源树
样例
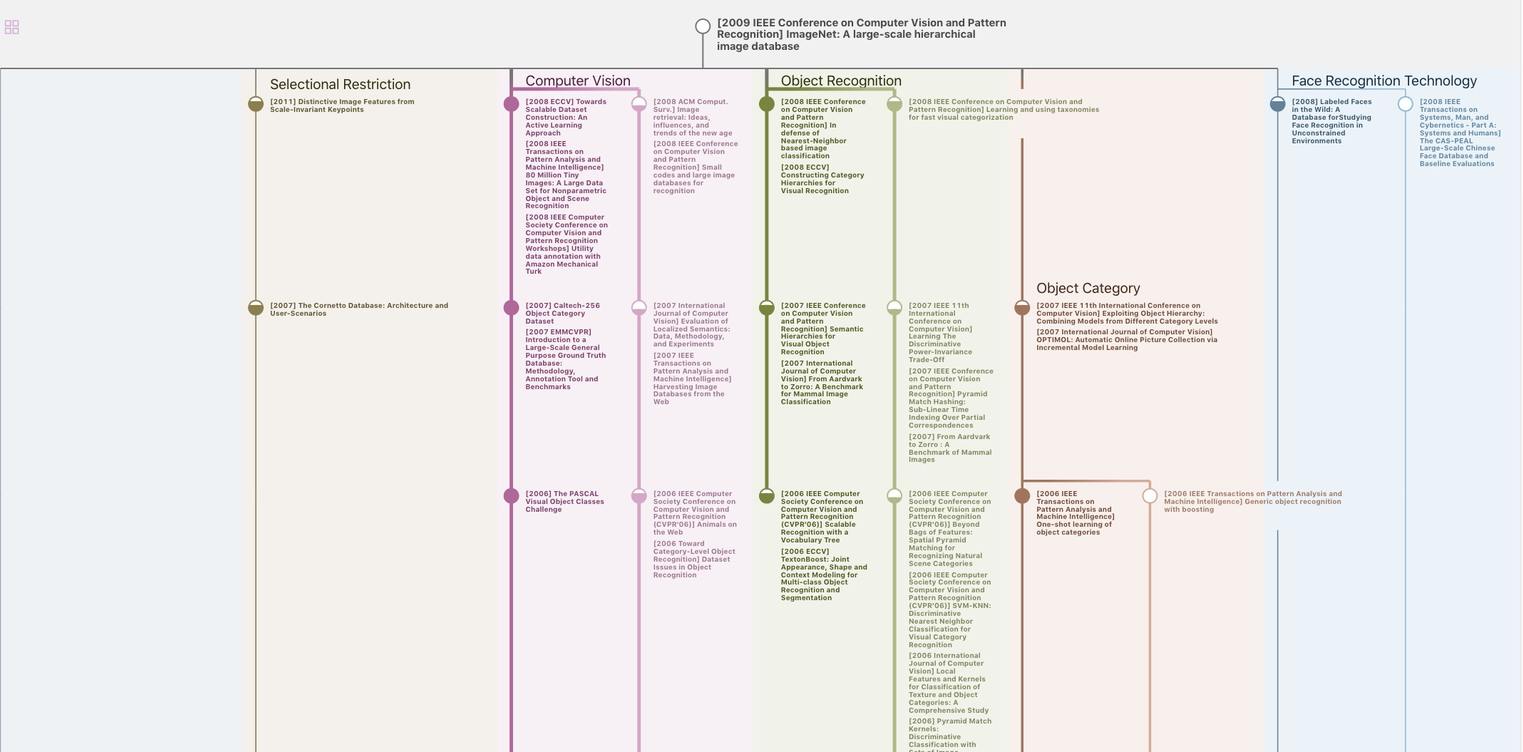
生成溯源树,研究论文发展脉络
Chat Paper
正在生成论文摘要