VIMTS: Variational-based Imputation for Multi-modal Time Series
2022 IEEE International Conference on Big Data (Big Data)(2022)
摘要
Multi-modal time series data in real applications often contain data of different dimensionalities, e.g., high-dimensional modality such as image data series, and low-dimensional univariate time series. Multi-modal time series data with missing high-dimensional modal values are ubiquitous in real-world classification and regression applications. To accurately predict the target labels, it is important to appropriately impute the high-dimensional modal missing values. However, most existing imputation methods focus on multivariate time series, fail to simultaneously consider temporal dependencies within each series and the correlations across the series, and also lack a probabilistic interpretation. In this paper, we propose a novel method, which uses a new structured variational approximation technique for the imputation of missing values in multi-modal time series. Instead of directly imputing high-dimensional modal missing values, we use the variational approximation technique to impute intermediate lower-dimensional feature representations of high-dimensional modal missing values from simple modalities related to high-dimensional modality and then feed them into a dynamical model. The dynamical model captures the temporal dependencies of the feature representations and finally predicts the target labels. In order to address the optimization difficulties caused by the lack of ground truth values of lower-dimensional feature representations, we also propose a two-stage isolated optimization strategy for better convergence. We evaluate our method on a real-world stream monitoring dataset. Our extensive experiments demonstrate that the proposed method outperforms several state-of-the-art methods in both data imputation and prediction performance.
更多查看译文
关键词
time series,multi-modal,imputation,missing data
AI 理解论文
溯源树
样例
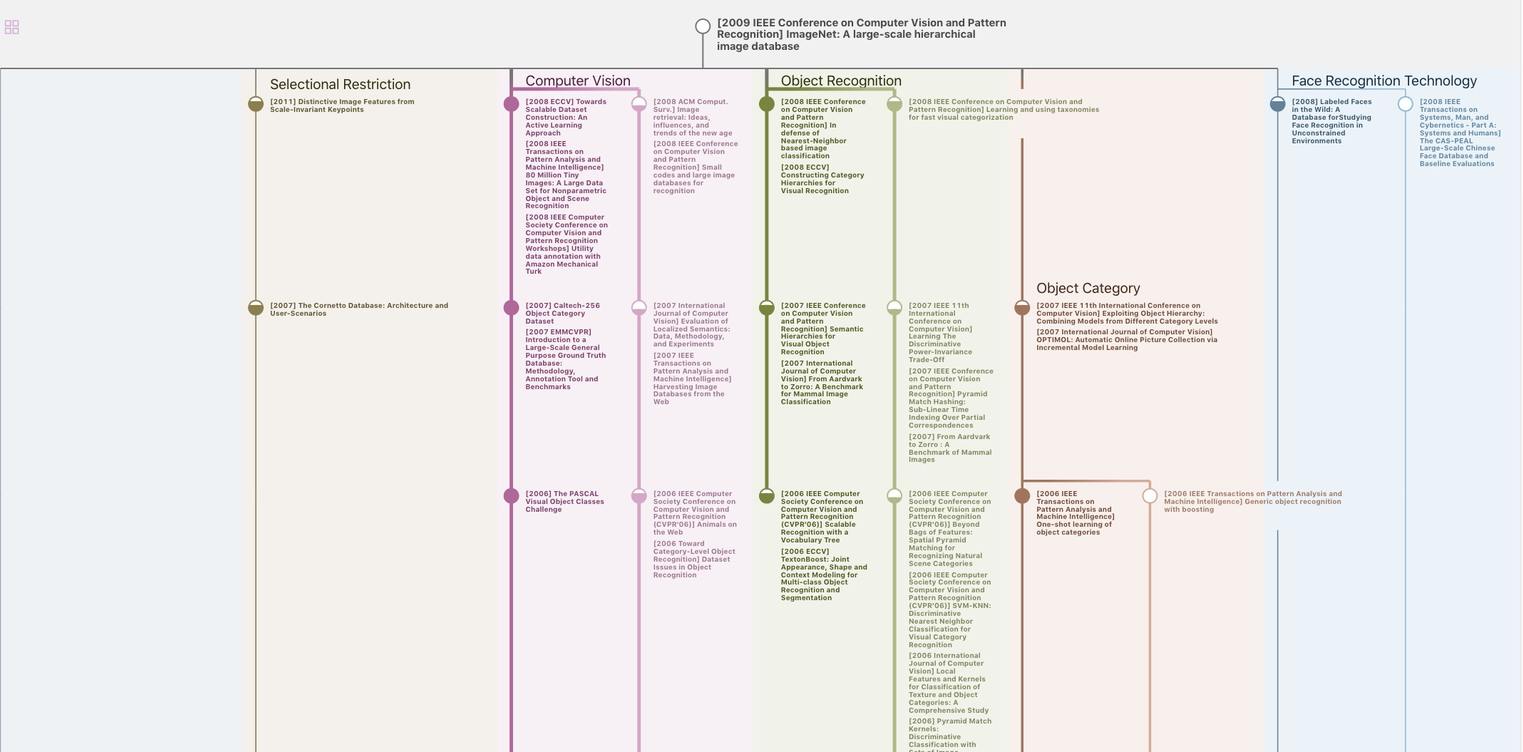
生成溯源树,研究论文发展脉络
Chat Paper
正在生成论文摘要