High-Efficient and Few-shot Adaptive Encrypted Traffic Classification with Deep Tree
MILCOM 2022 - 2022 IEEE Military Communications Conference (MILCOM)(2022)
Abstract
Although network traffic classification has been investigated for decades, the core challenges, including the complex and capricious conditions of network traffic, and the practical application of models, remain unsolved. Meanwhile, the extensive usage of encryption protocols makes encrypted traffic classification become a new challenge. The rapid iteration of network traffic brings the scale drift of encrypted traffic classification. While bulky deep-learning-based methods can barely satisfy the lightweight demand in real-world scenarios. To solve this, we propose a efficient encrypted traffic classification method using Deep-Tree with multi-grained scanning and cascade tree to perform high-speed learning and multi classification task. It has the classification accuracy and representation ability of depth model with lightweight computing expenses. The self-adaption and expandable ability of the model make it suit different traffic scenarios without specific model adaptation. The experimental results show that the proposed method achieves superior performance compared with state-of-the-art methods. Particularly, our method can dynamically adapt traffic classification tasks at different scales.
MoreTranslated text
Key words
Encrypted Traffic Classification,Model Adaption,Lightweight Computation,High-Efficient Implementation
AI Read Science
Must-Reading Tree
Example
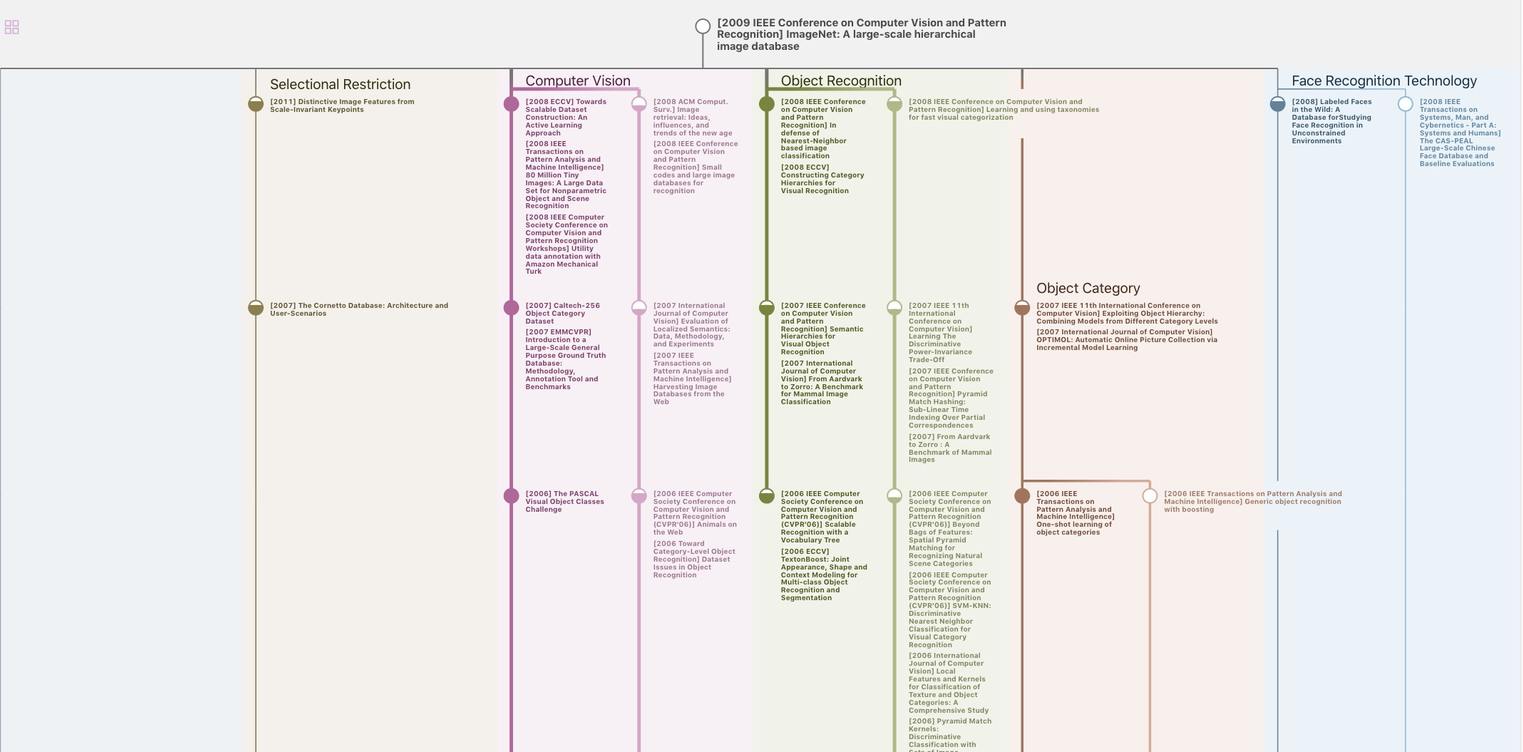
Generate MRT to find the research sequence of this paper
Chat Paper
Summary is being generated by the instructions you defined