A Proximal Sensor-Based Approach for Clean, Fast, and Accurate Assessment of the Eucalyptus spp. Nutritional Status and Differentiation of Clones.
Plants (Basel, Switzerland)(2023)
摘要
Several materials have been characterized using proximal sensors, but still incipient efforts have been driven to plant tissues. spp. cultivation in Brazil covers approximately 7.47 million hectares, requiring faster methods to assess plant nutritional status. This study applies portable X-ray fluorescence (pXRF) spectrometry to (i) distinguish clones using pre-processed pXRF data; and (ii) predict the contents of eleven nutrients in the leaves of (B, Ca, Cu, Fe, K, Mg, Mn, N, P, S, and Zn) aiming to accelerate the diagnosis of nutrient deficiency. Nine hundred and twenty samples of leaves were collected, oven-dried, ground, and analyzed using acid-digestion (conventional method) and using pXRF. Six machine learning algorithms were trained with 70% of pXRF data to model conventional results and the remaining 30% were used to validate the models using root mean square error (RMSE) and coefficient of determination (R). The principal component analysis clearly distinguished developmental stages based on pXRF data. Nine nutrients were accurately predicted, including N (not detected using pXRF spectrometry). Results for B and Mg were less satisfactory. This method can substantially accelerate decision-making and reduce costs for foliar analysis, constituting an ecofriendly approach which should be tested for other crops.
更多查看译文
关键词
Eucalyptus cultivation,greentech analysis,leaf nutrient analysis,machine learning,plant mineral nutrition,portable X-ray fluorescence (pXRF) spectrometry,proximal sensing
AI 理解论文
溯源树
样例
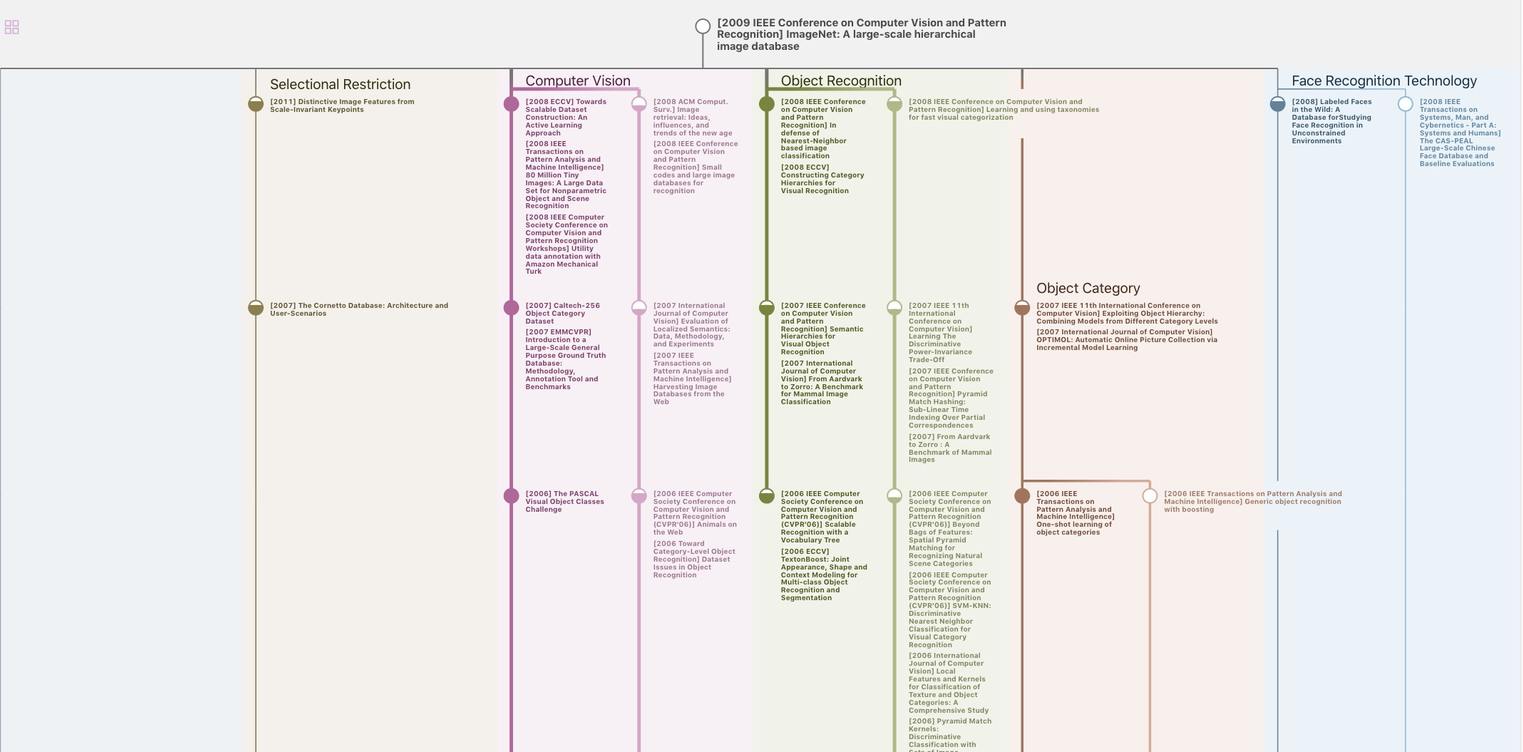
生成溯源树,研究论文发展脉络
Chat Paper
正在生成论文摘要