Self-Supervised RGB-T Tracking with Cross-Input Consistency
CoRR(2023)
摘要
In this paper, we propose a self-supervised RGB-T tracking method. Different from existing deep RGB-T trackers that use a large number of annotated RGB-T image pairs for training, our RGB-T tracker is trained using unlabeled RGB-T video pairs in a self-supervised manner. We propose a novel cross-input consistency-based self-supervised training strategy based on the idea that tracking can be performed using different inputs. Specifically, we construct two distinct inputs using unlabeled RGB-T video pairs. We then track objects using these two inputs to generate results, based on which we construct our cross-input consistency loss. Meanwhile, we propose a reweighting strategy to make our loss function robust to low-quality training samples. We build our tracker on a Siamese correlation filter network. To the best of our knowledge, our tracker is the first self-supervised RGB-T tracker. Extensive experiments on two public RGB-T tracking benchmarks demonstrate that the proposed training strategy is effective. Remarkably, despite training only with a corpus of unlabeled RGB-T video pairs, our tracker outperforms seven supervised RGB-T trackers on the GTOT dataset.
更多查看译文
关键词
tracking,consistency,self-supervised,cross-input
AI 理解论文
溯源树
样例
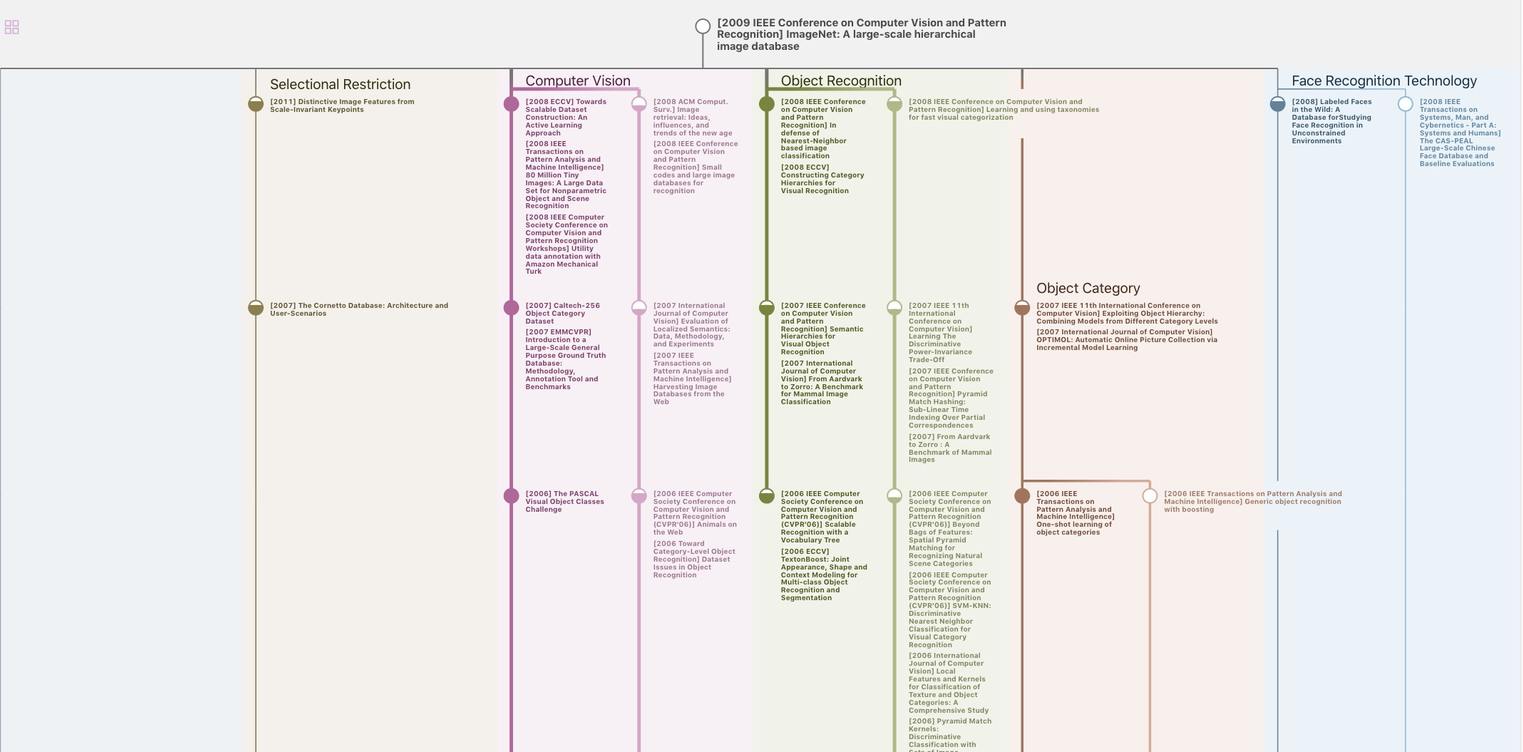
生成溯源树,研究论文发展脉络
Chat Paper
正在生成论文摘要