Double Deep Reinforcement Learning Techniques for Low Dimensional Sensing Mapless Navigation of Terrestrial Mobile Robots
arxiv(2023)
摘要
In this work, we present two Deep Reinforcement Learning (Deep-RL) approaches to enhance the problem of mapless navigation for a terrestrial mobile robot. Our methodology focus on comparing a Deep-RL technique based on the Deep Q-Network (DQN) algorithm with a second one based on the Double Deep Q-Network (DDQN) algorithm. We use 24 laser measurement samples and the relative position and angle of the agent to the target as information for our agents, which provide the actions as velocities for our robot. By using a low-dimensional sensing structure of learning, we show that it is possible to train an agent to perform navigation-related tasks and obstacle avoidance without using complex sensing information. The proposed methodology was successfully used in three distinct simulated environments. Overall, it was shown that Double Deep structures further enhance the problem for the navigation of mobile robots when compared to the ones with simple Q structures.
更多查看译文
关键词
sensing mapless navigation,terrestrial mobile robots,reinforcement learning
AI 理解论文
溯源树
样例
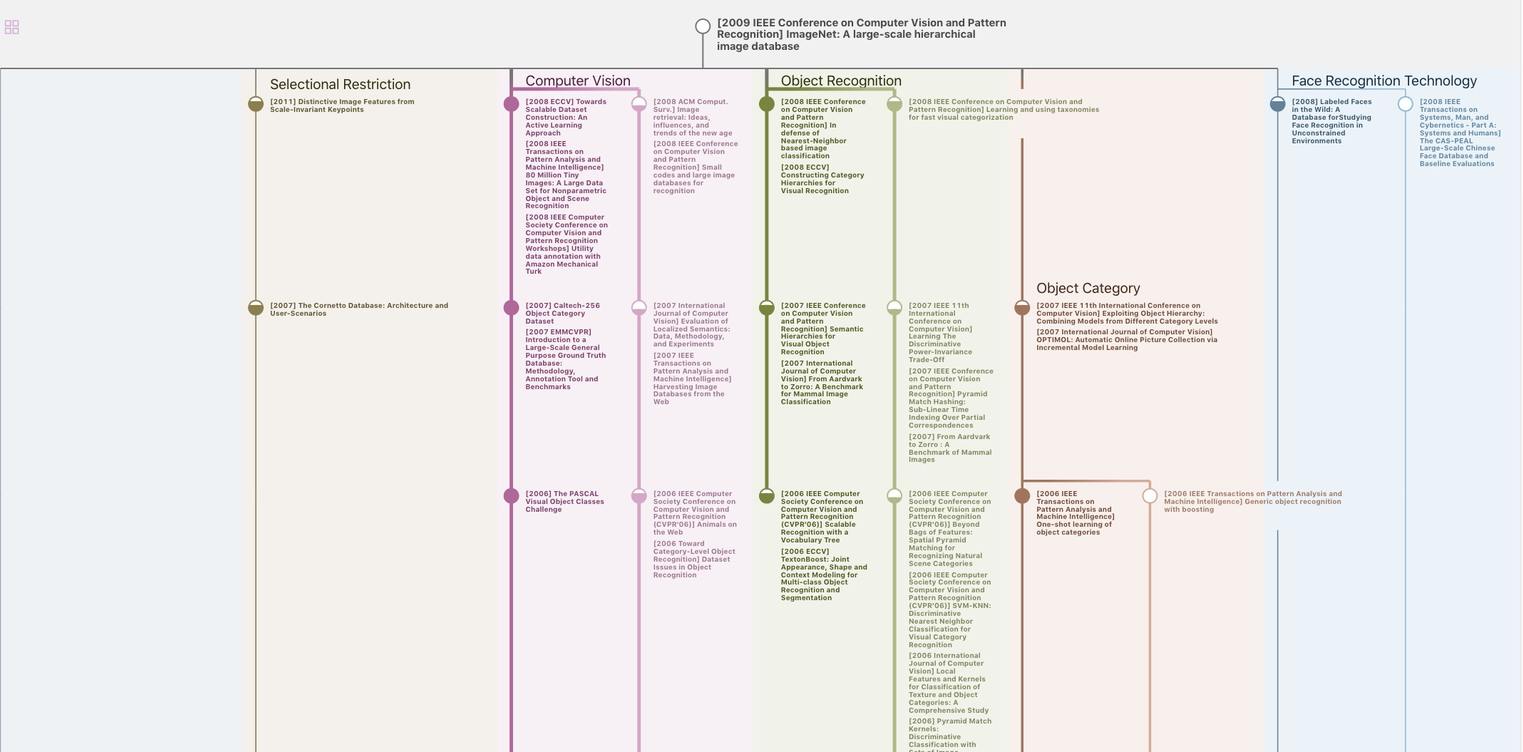
生成溯源树,研究论文发展脉络
Chat Paper
正在生成论文摘要