Individualized prescriptive inference in ischaemic stroke
arXiv (Cornell University)(2023)
Abstract
The gold standard in the treatment of ischaemic stroke is set by evidence
from randomized controlled trials. Yet the manifest complexity of the brain's
functional, connective, and vascular architectures introduces heterogeneity in
treatment susceptibility that violates the underlying statistical premisses,
potentially leading to substantial errors at both individual and population
levels. The counterfactual nature of therapeutic inference has made quantifying
the impact of this defect difficult. Combining large-scale meta-analytic
connective, functional, genetic expression, and receptor distribution data with
high-resolution maps of 4 119 acute ischaemic lesions, here we conduct a
comprehensive series of semi-synthetic virtual interventional trials,
quantifying the fidelity of the traditional approach in inferring individual
treatment effects against biologically plausible, empirically informed ground
truths, across 103 628 800 distinct simulations. Combining deep generative
models expressive enough to capture the observed lesion heterogeneity with
flexible causal modelling, we find that the richness of the lesion
representation is decisive in determining individual-level fidelity, even where
freedom from treatment allocation bias cannot be guaranteed. Our results
indicate that complex modelling with richly represented lesion data is critical
to individualized prescriptive inference in ischaemic stroke.
MoreTranslated text
Key words
ischaemic stroke,individualised prescriptive inference
AI Read Science
Must-Reading Tree
Example
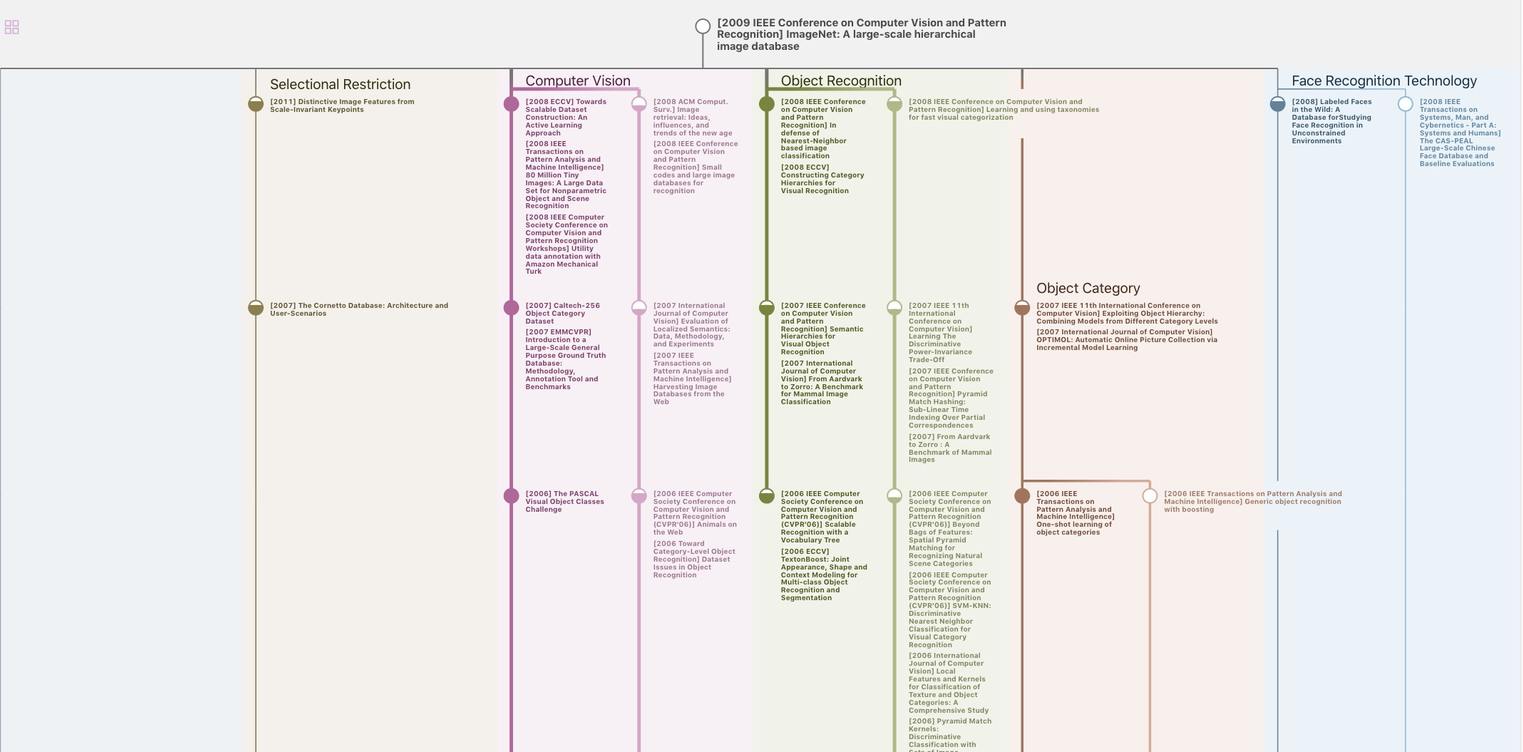
Generate MRT to find the research sequence of this paper
Chat Paper
Summary is being generated by the instructions you defined