Tensor robust principal component analysis of lightning images: butterfly effect of blackholes
arXiv (Cornell University)(2022)
Abstract
Tensor robust principal component analysis (robust PCA) has been applied to the lightning images. Robust PCA aims to classify the images into low-rank and sparse components. The low rank and sparse components correspond to static background separation and the dynamic (lightning) part of the images correspondingly. After classification, Singular Value Decomposition (SVD) unfold technique has been applied to sparse tensor, which transforms the tensor to spatial-temporal spaces in the form of vector matrixes. Spectral evolution shows the evolution of the polarization of the UV-Vis spectra. The contour maps of 2D energy density plots reveal the zero-point energy fluctuations of the bosons, fermions, and virtual matters from early to late stages. Detection of such fluctuations in the early stages can help to remote sensing of the lightning. 3D vector(ether) field representation of the sparse tensor of the brightest lightning reveals the butterfly effect of the black holes with the signatures of flapping. There is a correlation between the approximate entropy and the chaos trends of the 3D vector field analyzed by the Lyapunov exponent. In the brightest frame, the fractal dimension measures 0.8004, which is close to the Fibonacci Hamiltonian.
MoreTranslated text
Key words
robust principal component analysis,lightning images
AI Read Science
Must-Reading Tree
Example
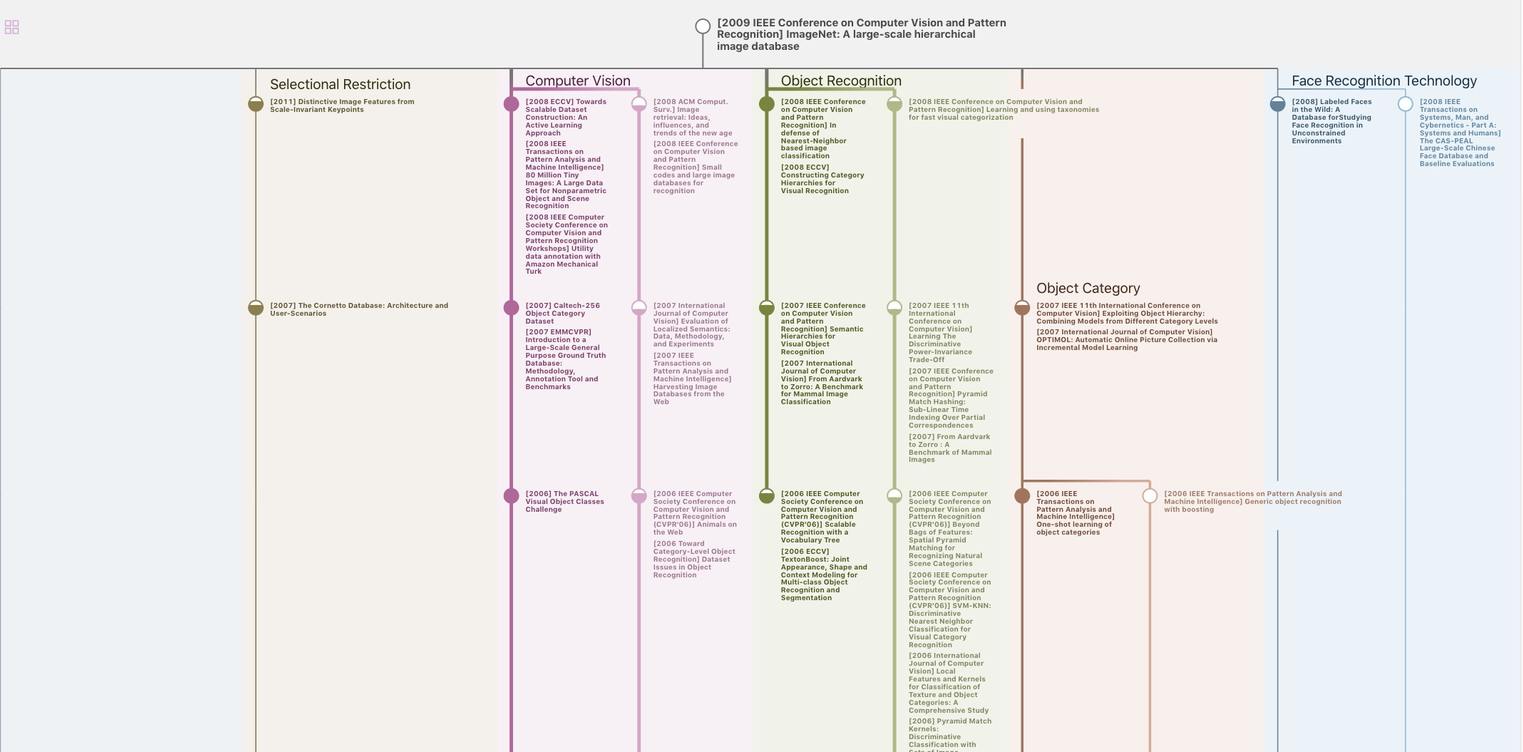
Generate MRT to find the research sequence of this paper
Chat Paper
Summary is being generated by the instructions you defined