NeSIG: A Neuro-Symbolic Method for Learning to Generate Planning Problems
arxiv(2023)
摘要
In the field of Automated Planning there is often the need for a set of
planning problems from a particular domain, e.g., to be used as training data
for Machine Learning or as benchmarks in planning competitions. In most cases,
these problems are created either by hand or by a domain-specific generator,
putting a burden on the human designers. In this paper we propose NeSIG, to the
best of our knowledge the first domain-independent method for automatically
generating planning problems that are valid, diverse and difficult to solve. We
formulate problem generation as a Markov Decision Process and train two
generative policies with Deep Reinforcement Learning to generate problems with
the desired properties. We conduct experiments on three classical domains,
comparing our approach against handcrafted, domain-specific instance generators
and various ablations. Results show NeSIG is able to automatically generate
valid and diverse problems of much greater difficulty (15.5 times more on
geometric average) than domain-specific generators, while simultaneously
reducing human effort when compared to them. Additionally, it can generalize to
larger problems than those seen during training.
更多查看译文
AI 理解论文
溯源树
样例
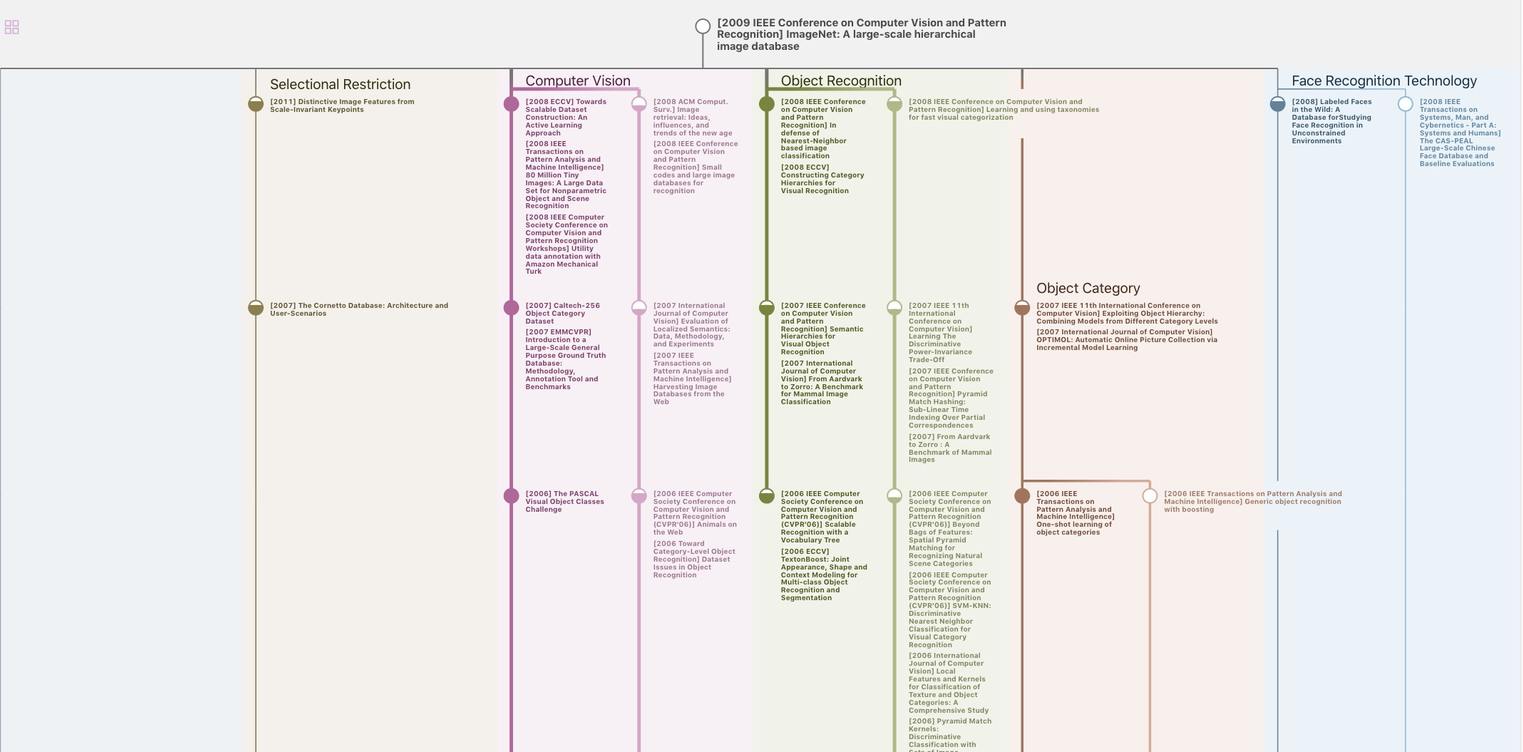
生成溯源树,研究论文发展脉络
Chat Paper
正在生成论文摘要