A two-stage deep learning architecture for model reduction of parametric time-dependent problems
COMPUTERS & MATHEMATICS WITH APPLICATIONS(2023)
摘要
Parametric time-dependent systems are of a crucial importance in modeling real phenomena, often characterized by nonlinear behaviours too. Those solutions are typically difficult to generalize in a sufficiently wide parameter space while counting on limited computational resources available. As such, we present a general two-stage deep learning framework able to perform that generalization with low computational effort in time. It consists in a separated training of two pipe-lined predictive models. At first, a certain number of independent neural networks are trained with data-sets taken from different subsets of the parameter space. Successively, a second predictive model is specialized to properly combine the first-stage guesses and compute the right predictions. Promising results are obtained applying the framework to incompressible Navier-Stokes equations in a cavity (Rayleigh-Benard cavity), obtaining a 97% reduction in the computational time comparing with its numerical resolution for a new value of the Grashof number.
更多查看译文
关键词
Reduced order modeling,Deep learning,Long-short term memory networks,Convolutional layers,Time forecasting,Time-dependent parametric PDEs
AI 理解论文
溯源树
样例
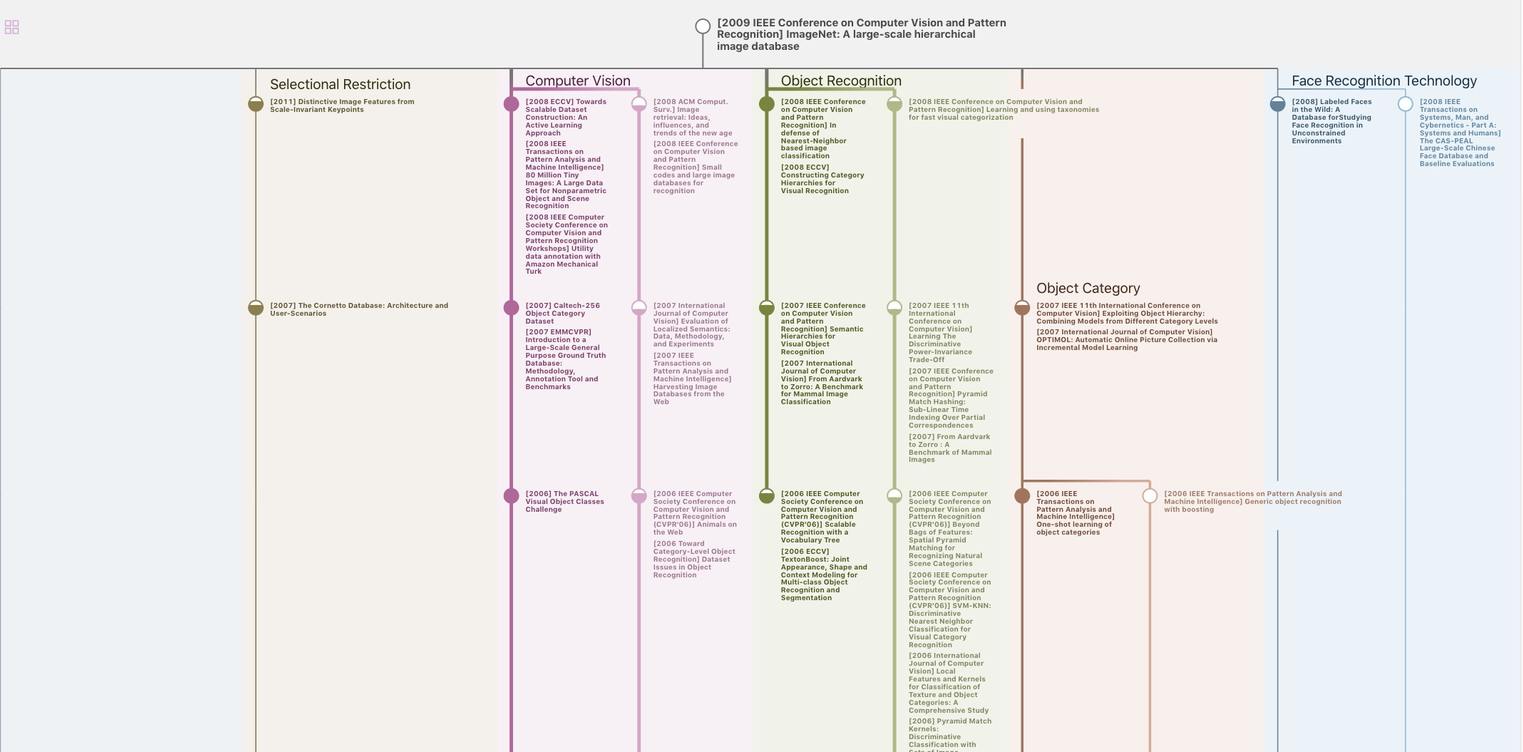
生成溯源树,研究论文发展脉络
Chat Paper
正在生成论文摘要