Implementation of the critical wave groups method with computational fluid dynamics and neural networks
OCEAN ENGINEERING(2024)
摘要
ABS T R A C T Accurate and efficient prediction of extreme ship responses continues to be a challenging problem in ship hy-drodynamics. Probabilistic frameworks in conjunction with computationally efficient numerical hydrodynamic tools have been developed that allow researchers and designers to better understand extremes. However, the ability of these hydrodynamic tools to represent the physics quantitatively during extreme events is limited. Previous research successfully implemented the critical wave groups (CWG) probabilistic method with computational fluid dynamics (CFD). Although the CWG method allows for less simulation time than a Monte Carlo approach, the large quantity of simulations required is cost prohibitive. The objective of the present paper is to reduce the computational cost of implementing CWG with CFD, through the construction of long short-term memory (LSTM) neural networks. After training the models with a limited quantity of simulations, the models can provide a larger quantity of predictions to calculate the probability. The new framework is demonstrated with a 2-D midship section of the Office of Naval Research Tumblehome (ONRT) hull in Sea State 7 and beam seas at zero speed. The new framework is able to produce predictions that are representative of a purely CFD-driven CWG framework, with two orders of magnitude of computational cost savings.
更多查看译文
关键词
Computational fluid dynamics,Neural networks,Extreme events,Wave groups,Machine learning,Seakeeping,Ship hydrodynamics
AI 理解论文
溯源树
样例
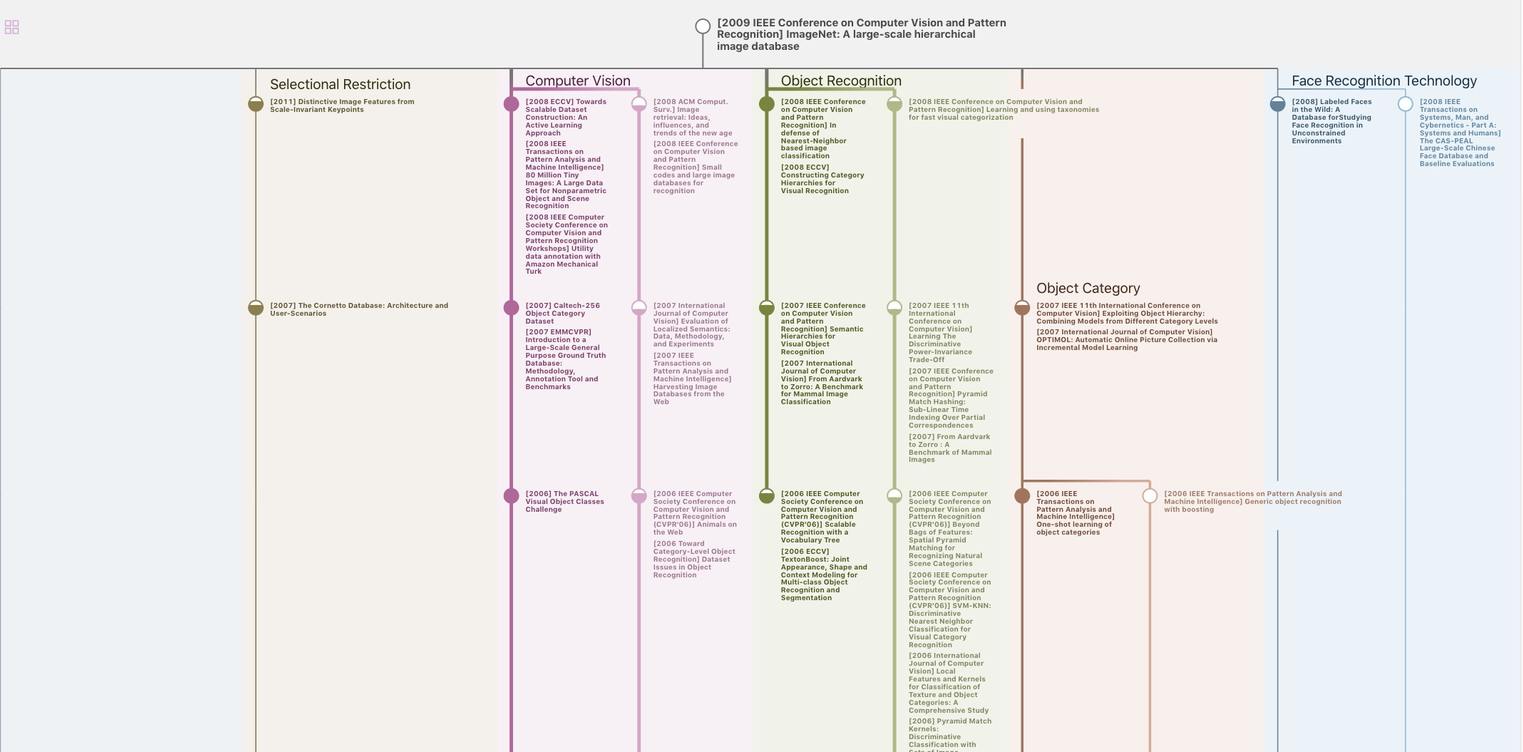
生成溯源树,研究论文发展脉络
Chat Paper
正在生成论文摘要