A Deep Reinforcement Learning Based Efficient Optimization Solution Method for Inverse Kinematics of Hyper-redundant Robot
2022 IEEE International Conference on Robotics and Biomimetics (ROBIO)(2022)
摘要
The hyper-redundant robot has remarkable flexibility and obstacle-avoiding ability because of its ultrahigh redundancy. Due to the complicated nonlinear property with high dimension, the inverse kinematics solving is difficult and inefficient. In order to improve the solution efficiency, the deep reinforcement learning based efficient optimization (DRL-PSO) solution method is proposed. The deep reinforcement learning (DRL) algorithm is used to fleetly determine the initial inverse kinematics solution. Based on the initial solution, the particle swarm optimization (PSO) is applied to quickly provide the optimized inverse kinematics solution and guarantee a relatively smooth configuration of the hyper-redundant robot. The results of the simulation tests show that the solution time of the proposed method is reduced by more 90% than of the traditional pseudo-inverse method. Finally, the proposed method is applied to a 20-DOF hyper-redundant robot to verify its practicability.
更多查看译文
关键词
inverse kinematics,reinforcement learning,robot,optimization,hyper-redundant
AI 理解论文
溯源树
样例
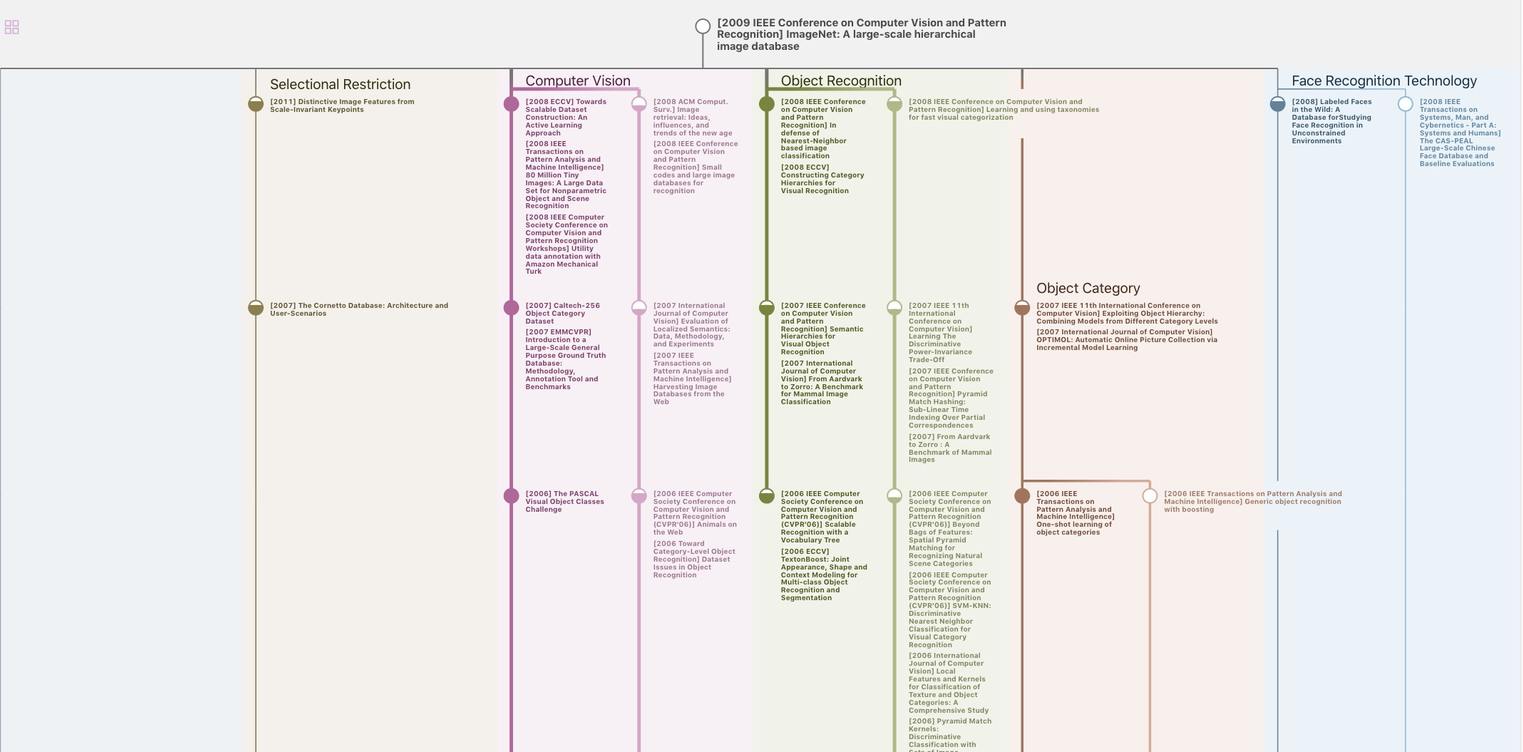
生成溯源树,研究论文发展脉络
Chat Paper
正在生成论文摘要