Employing Super Resolution to Improve Low-Quality Deepfake Detection
2022 22nd International Conference on Advances in ICT for Emerging Regions (ICTer)(2022)
摘要
The rapid progress in deepfake content generation has now come to a point where it raises significant concerns about the implications for society. Therefore, a new challenge of detecting deepfakes arises to protect individuals from potential misuse. Even though introduced detection algorithms perform well on high-quality deepfakes, detecting low-quality deepfakes has been challenging. As a remedy, researchers try to feed more training data to increase detection ability. HoWever, providing more data and processing them is not always feasible in a practical scenario. Thus, for the first time in this domain, we propose to employ super-resolution (SR) as a preprocessing step instead of feeding more data to improve low-quality deepfake detection. Extensive experiments were conducted on the FaceForensics++ deepfake dataset. Initially, three baseline models, Meso-4, MesoInception-4, and XceptionNet, were trained and tested on the dataset without any preprocessing mechanism. XceptionNet outperformed with 90.54% accuracy revealing deeper networks detect low-quality depfakes adequately. Then those baseline models were trained with SR preprocessing. To do that, we employed two SR networks, called VDSR and RSRGAN. RESRGAN+XceptionNet outperformed the previous baseline models by obtaining 96.05% accuracy, showing SR preprocessing usefulness in low-quality deepfake detection. Further experiments utilizing performance metrics, statistical tests, and visualization of activation maps showed that SR preprocessing is promising when applied to deepfake detection networks and detection algorithms experience a significant performance.
更多查看译文
关键词
Deepfake,deep learning (DL),detection,low-quality,super-resolution (SR)
AI 理解论文
溯源树
样例
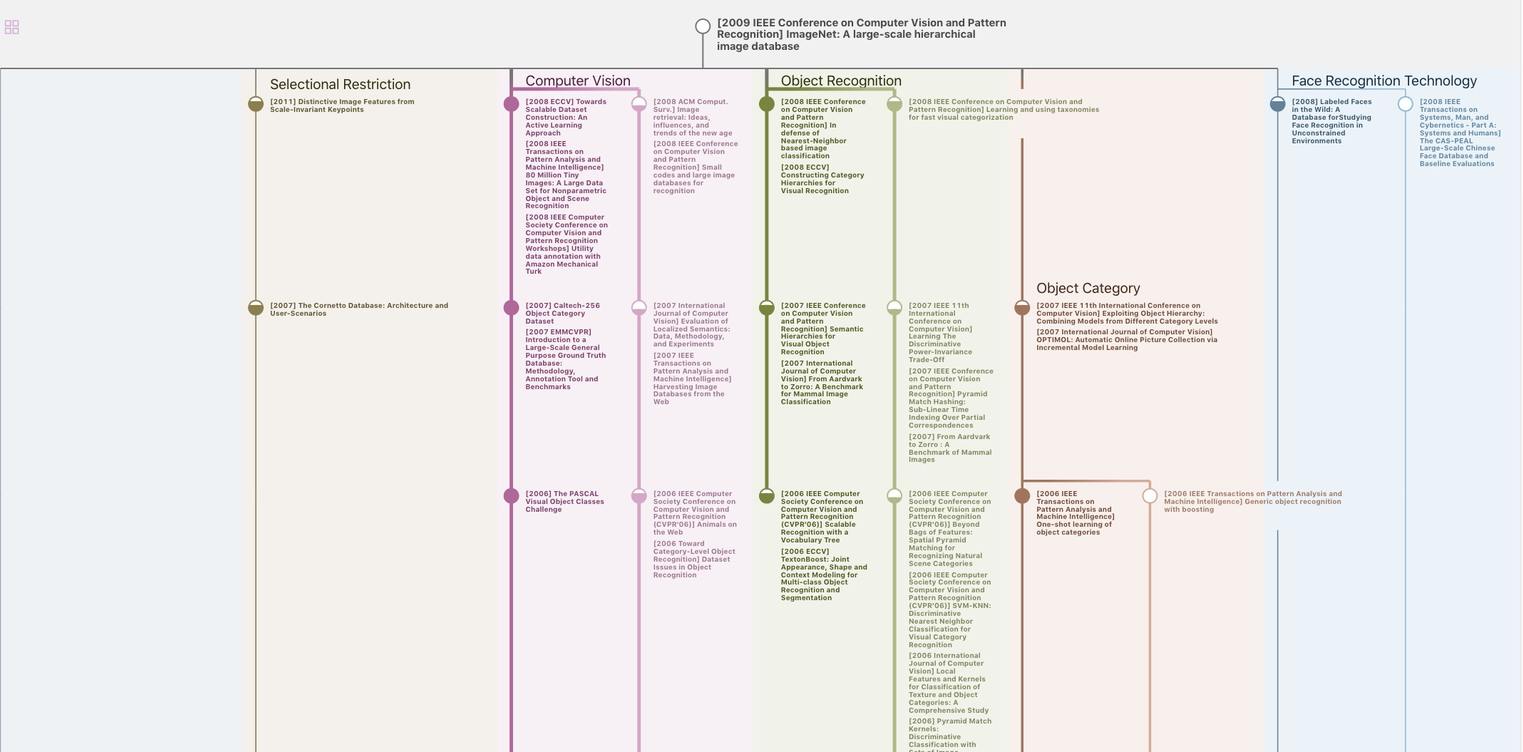
生成溯源树,研究论文发展脉络
Chat Paper
正在生成论文摘要