Unsupervised Semantic Segmentation with Feature Enhancement for Few-shot Image Classification
2022 Tenth International Conference on Advanced Cloud and Big Data (CBD)(2022)
摘要
Image classification is a typical task in big data applications. As a few-shot learning (FSL) task, the few-shot image classification attempts to learn a new visual concept from limited labelled images. The existing few-shot image classification methods usually fail to effectively eliminate the interference of image background information, thus affecting the accuracy of image classification. To overcome the shortcomings of existing methods and eliminate the interference of background information, this paper proposes a novel method named Unsupervised Semantic Segmentation with Feature Enhancement (USSFE) for few-shot image classification. USSFE uses the dense contrastive learning to train a robust unsupervised semantic segmentation model for foreground segmentation, and enhances feature representations of the foreground feature maps by extracting the shape and texture features of the foreground images. Our experimental results on two benchmark datasets, miniImageNet and tieredImageNet, show that the proposed USSFE method achieves better performance than the state-of-the-art methods.
更多查看译文
关键词
few-shot image classification,few-shot learning,N-way K-shot,metric-based learning,unsupervised semantic segmentation,feature enhancement
AI 理解论文
溯源树
样例
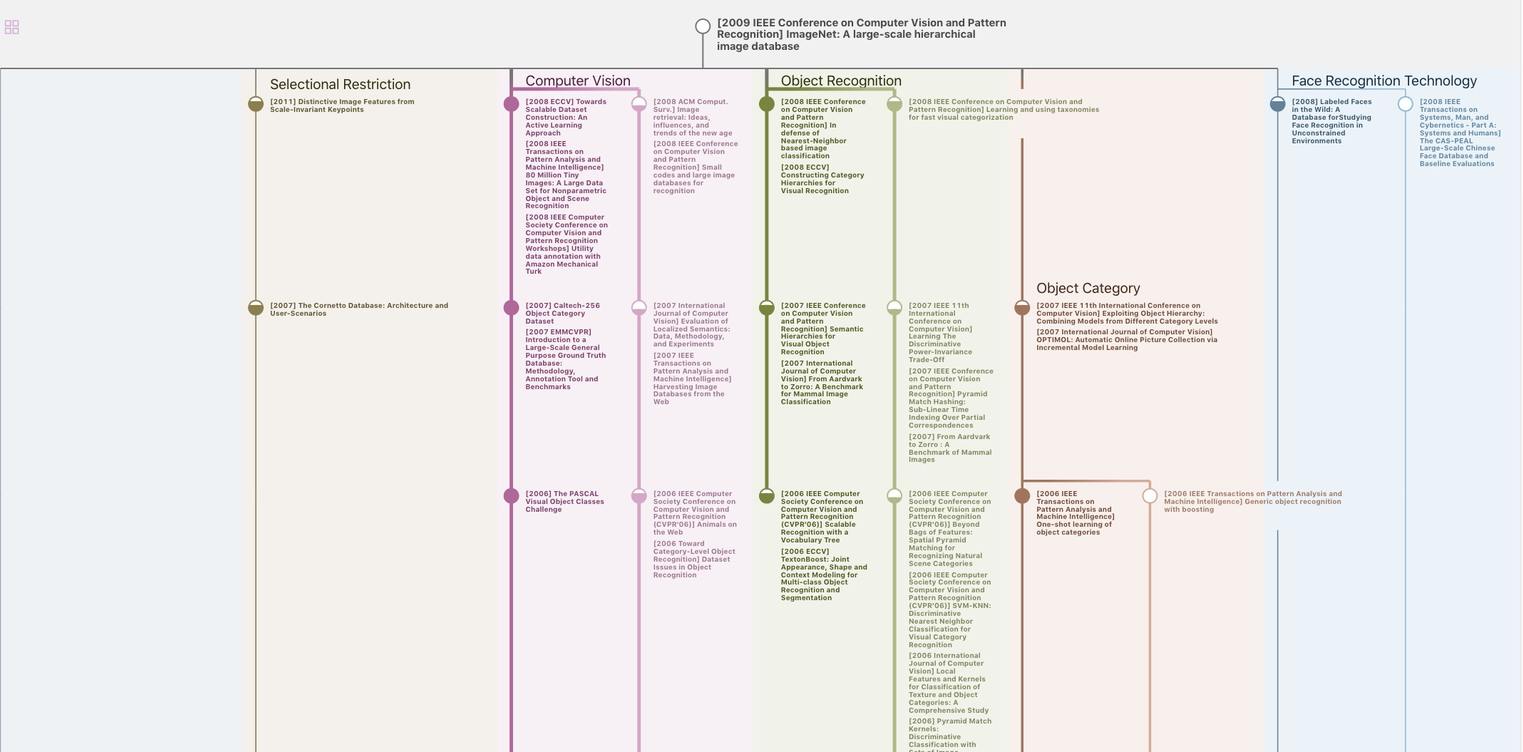
生成溯源树,研究论文发展脉络
Chat Paper
正在生成论文摘要