Detection of Multiple Faults in a Low-Power Wind Turbine by using Convolutional Neural Networks
2022 IEEE International Autumn Meeting on Power, Electronics and Computing (ROPEC)(2022)
摘要
Early fault detection in wind turbines has positive impacts on the costs of repair and maintenance. If the damage is early detected, the damage can be repaired before it increases and generates a complete failure. In this work, a methodology based on convolutional neural networks and the time-frequency representation of vibration signals for the detection of imbalance and bearing faults is presented. In general, the methodology consists of the acquisition of vibration signals from two damage types (blade imbalance and bearing fault) and the healthy condition. Then, a spectrogram function is applied to get an image from the time-frequency plane of the vibration signals. This image is segmented and analyzed by the convolutional neural network to detect the wind turbine condition. A MATLAB graphic user interface is developed to implement the proposed methodology. Results demonstrate the usefulness of the proposal as 100% of accuracy in the diagnosis is achieved.
更多查看译文
关键词
Bearing fault,Convolutional neural network,Imbalance condition,Spectrogram,Vibration signals,Wind turbine
AI 理解论文
溯源树
样例
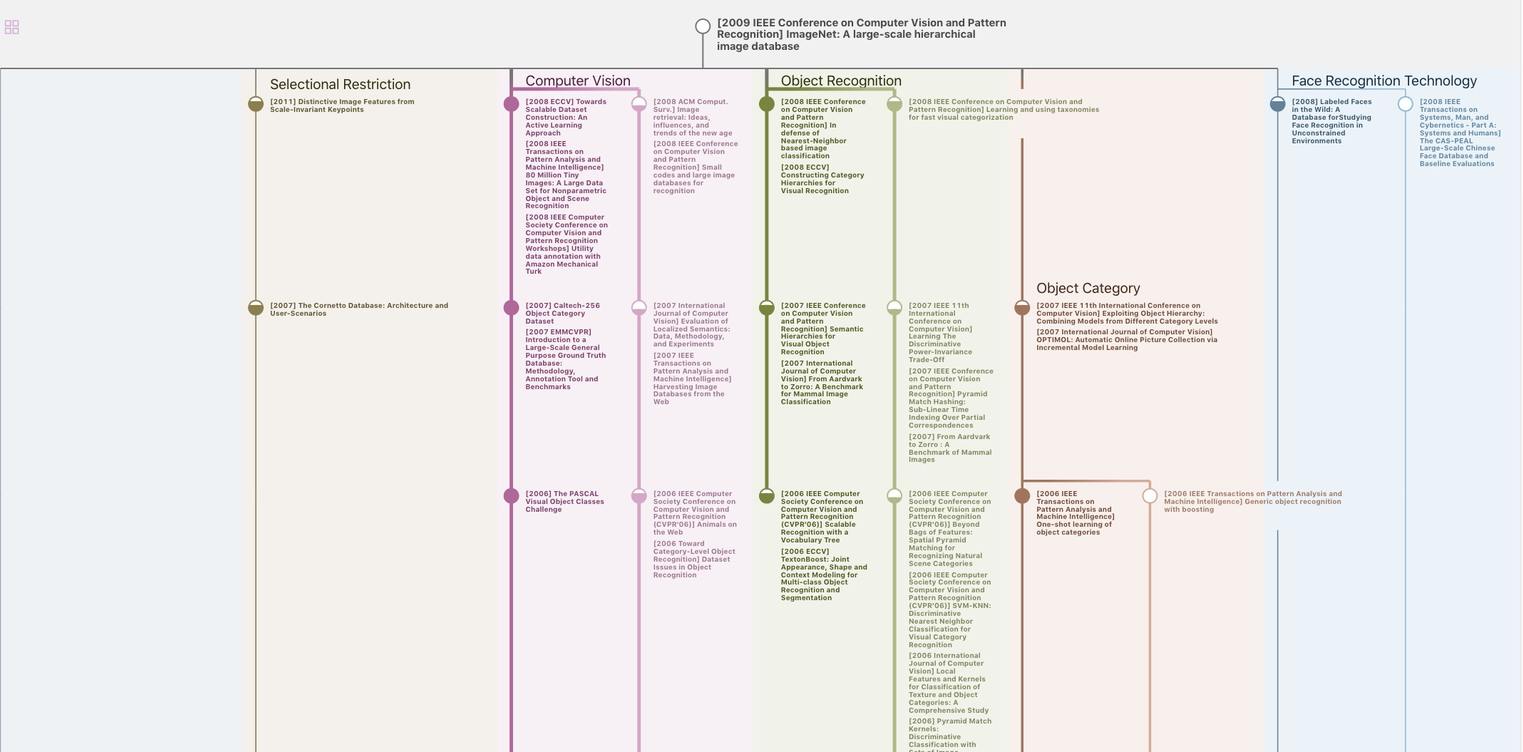
生成溯源树,研究论文发展脉络
Chat Paper
正在生成论文摘要