ProT-VAE: Protein Transformer Variational AutoEncoder for Functional Protein Design
biorxiv(2023)
Abstract
The data-driven design of protein sequences with desired function is challenged by the absence of good theoretical models for the sequence-function mapping and the vast size of protein sequence space. Deep generative models have demonstrated success in learning the sequence to function relationship over natural training data and sampling from this distribution to design synthetic sequences with engineered functionality. We introduce a deep generative model termed the Protein Transformer Variational AutoEncoder (ProT-VAE) that furnishes an accurate, generative, fast, and transferable model of the sequence-function relationship for data-driven protein engineering by blending the merits of variational autoencoders to learn interpretable, low-dimensional latent embeddings and fully generative decoding for conditional sequence design with the expressive, alignment-free featurization offered by transformers. The model sandwiches a lightweight, task-specific variational autoencoder between generic, pre-trained transformer encoder and decoder stacks to admit alignment-free training in an unsupervised or semi-supervised fashion, and interpretable low-dimensional latent spaces that facilitate understanding, optimization, and generative design of functional synthetic sequences. We implement the model using NVIDIA’s BioNeMo framework and validate its performance in retrospective functional prediction and prospective design of novel protein sequences subjected to experimental synthesis and testing. The ProT-VAE latent space exposes ancestral and functional relationships that enable conditional generation of novel sequences with high functionality and substantial sequence diversity. We anticipate that the model can offer an extensible and generic platform for machine learning-guided directed evolution campaigns for the data-driven design of novel synthetic proteins with “super-natural” function.
### Competing Interest Statement
The authors have declared no competing interest.
MoreTranslated text
Key words
protein transformer variational autoencoder,functional protein design,prot-vae
AI Read Science
Must-Reading Tree
Example
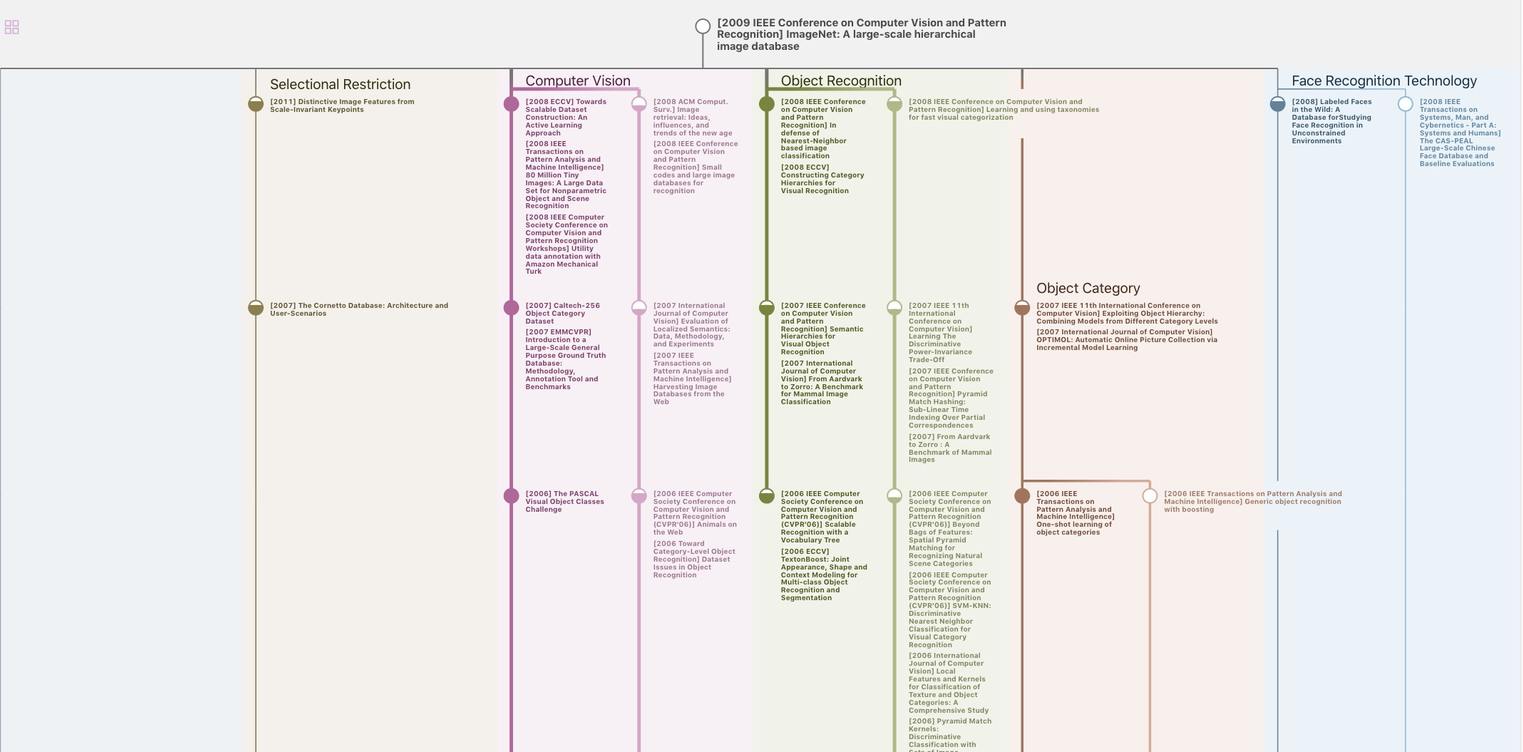
Generate MRT to find the research sequence of this paper
Chat Paper
Summary is being generated by the instructions you defined