Design of batch process with machine learning, feature extraction, and direct inverse analysis
Case Studies in Chemical and Environmental Engineering(2023)
摘要
1In the context of process design, control, and management, it has become common to utilize time-series data measured in industrial plants to construct mathematical models based on machine learning and to design and control processes based on these models. In a batch process y, it is difficult to design time-series data for each process variable x to meet the target value of the endpoint. Therefore, in this paper, we propose a method based on machine learning to design a batch process through the direct inverse analysis of a Gaussian mixture regression (GMR) model. The GMR model is constructed after the time-series data are transformed into latent variables. Through case studies, it is confirmed that the GMR model can predict y by inputting x into the model and design x for a new batch by inputting y into the model and inverse-transforming latent variables to x. The proposed method can successfully perform the direct inverse analysis of time-series data and design new profiles in the batch process, even when multiple y variables exist.
更多查看译文
关键词
Batch process optimization,Process design,Time-series data,Machine learning,Gaussian mixture regression,Direct inverse analysis
AI 理解论文
溯源树
样例
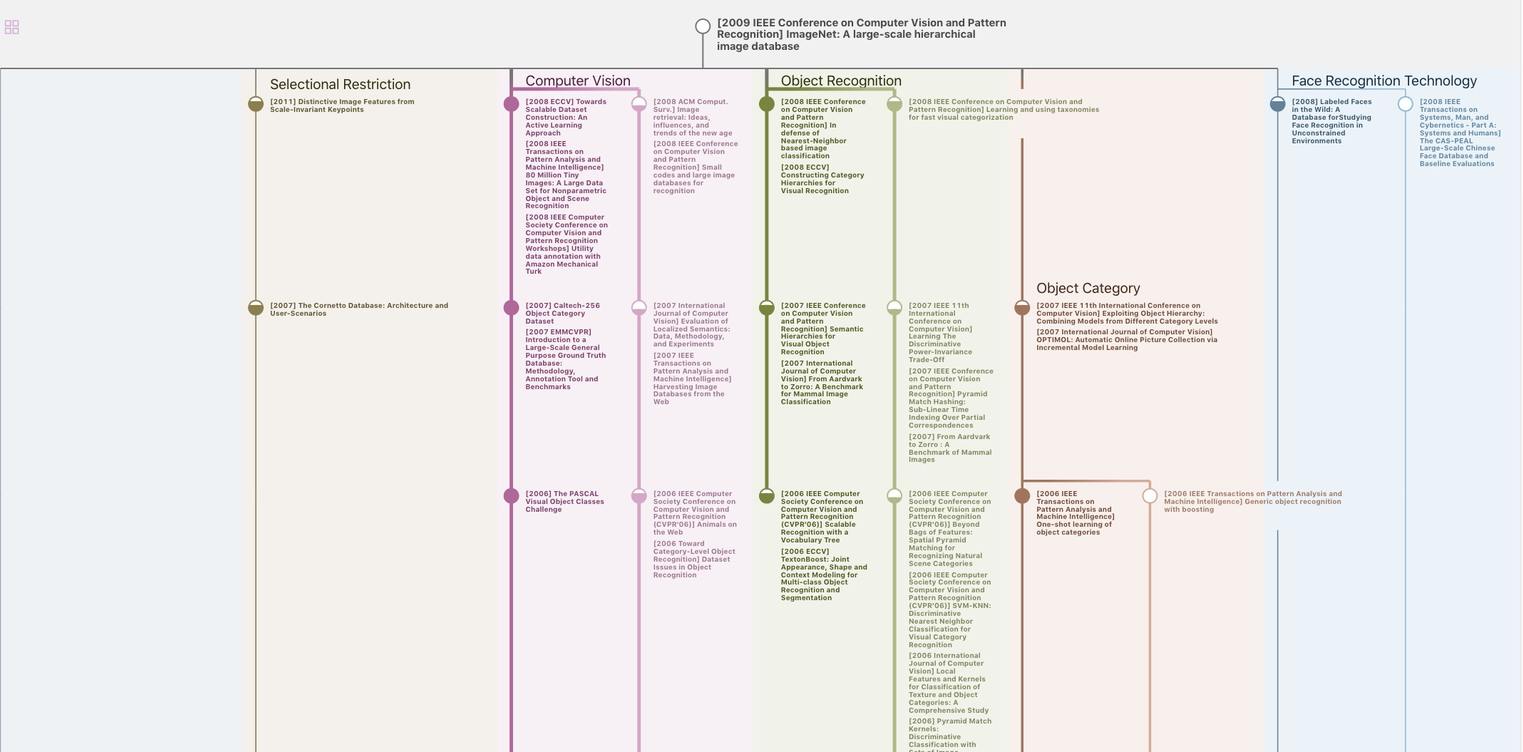
生成溯源树,研究论文发展脉络
Chat Paper
正在生成论文摘要