Delirium Prediction Using Machine Learning Interpretation Method and Its Incorporation into a Clinical Workflow
Applied Sciences(2023)
摘要
Delirium in hospitalized patients is a worldwide problem, causing a burden on healthcare professionals and impacting patient prognosis. A machine learning interpretation method (ML interpretation method) presents the results of machine learning predictions and promotes guided decisions. This study focuses on visualizing the predictors of delirium using a ML interpretation method and implementing the analysis results in clinical practice. Retrospective data of 55,389 patients hospitalized in a single acute care center in Japan between December 2017 and February 2022 were collected. Patients were categorized into three analysis populations, according to inclusion and exclusion criteria, to develop delirium prediction models. The predictors were then visualized using Shapley additive explanation (SHAP) and fed back to clinical practice. The machine learning-based prediction of delirium in each population exhibited excellent predictive performance. SHAP was used to visualize the body mass index and albumin levels as critical contributors to delirium prediction. In addition, the cutoff value for age, which was previously unknown, was visualized, and the risk threshold for age was raised. By using the SHAP method, we demonstrated that data-driven decision support is possible using electronic medical record data.
更多查看译文
关键词
learning health system,machine learning interpretation method,Shapley additive explanation,delirium,machine learning
AI 理解论文
溯源树
样例
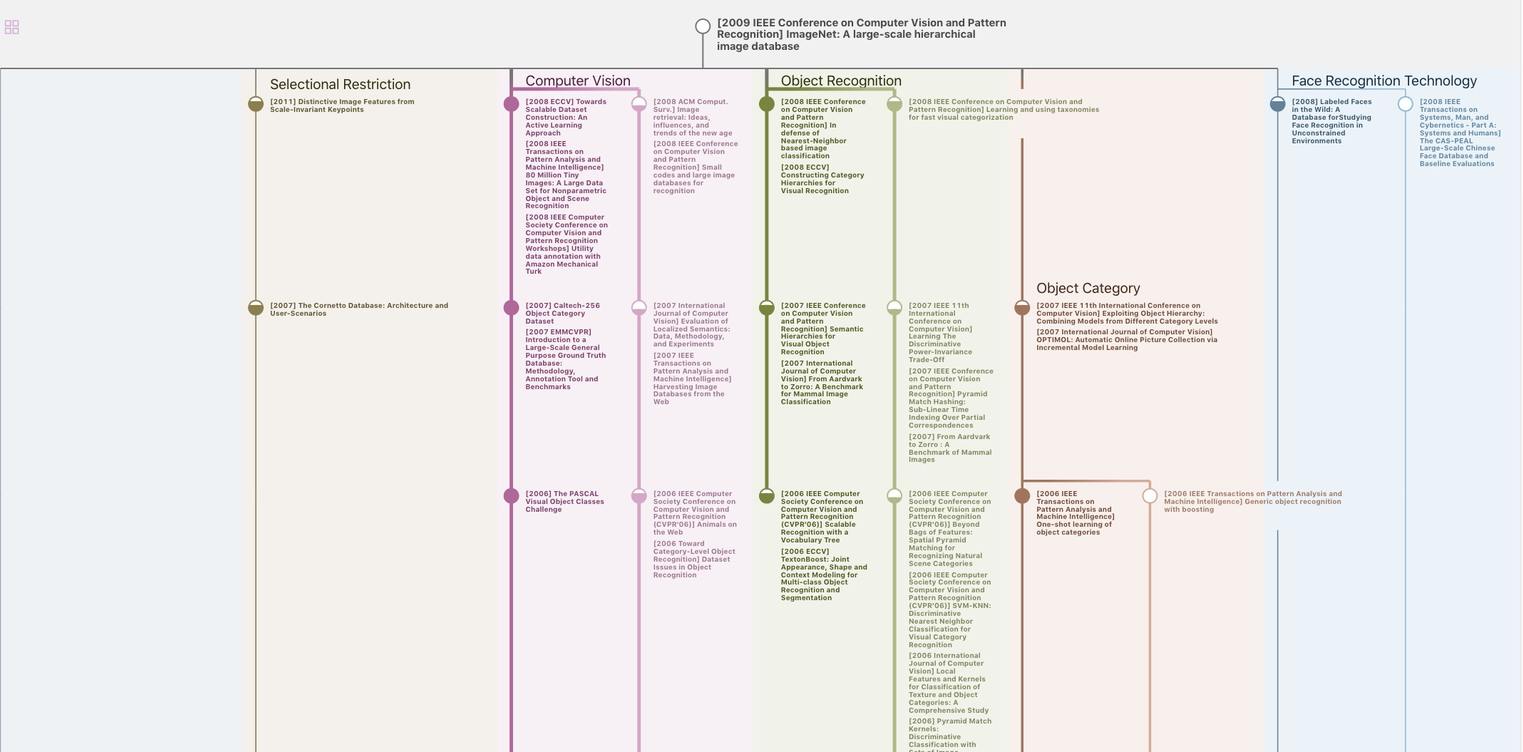
生成溯源树,研究论文发展脉络
Chat Paper
正在生成论文摘要