Deep-Learning Electron Diffractive Imaging
Physical review letters(2023)
摘要
We report the development of deep-learning coherent electron diffractive imaging at subangstrom resolution using convolutional neural networks (CNNs) trained with only simulated data. We exper-imentally demonstrate this method by applying the trained CNNs to recover the phase images from electron diffraction patterns of twisted hexagonal boron nitride, monolayer graphene, and a gold nanoparticle with comparable quality to those reconstructed by a conventional ptychographic algorithm. Fourier ring correlation between the CNN and ptychographic images indicates the achievement of a resolution in the range of 0.70 and 0.55 angstrom. We further develop CNNs to recover the probe function from the experimental data. The ability to replace iterative algorithms with CNNs and perform real-time atomic imaging from coherent diffraction patterns is expected to find applications in the physical and biological sciences.
更多查看译文
关键词
electron,imaging,deep-learning
AI 理解论文
溯源树
样例
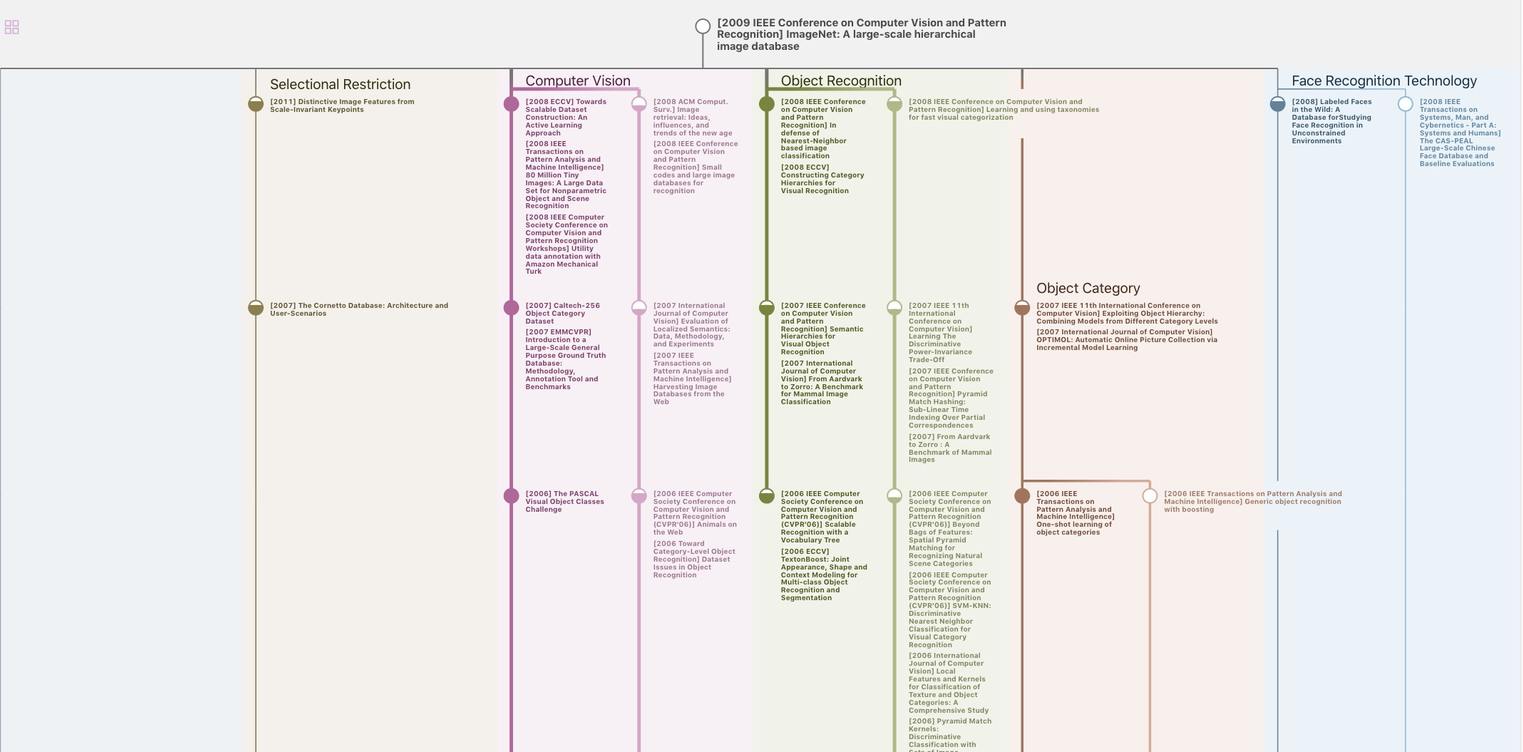
生成溯源树,研究论文发展脉络
Chat Paper
正在生成论文摘要