An Efficient Adversarial Defiance Towards Malware Detection System (MDS)
2022 IEEE 19th International Conference on Smart Communities: Improving Quality of Life Using ICT, IoT and AI (HONET)(2022)
摘要
Machine learning (ML) based Malware Detection Systems (MDS) are the potential target of Hackers. Malware authors usually have no information regarding the MDS's classifier and its parameters. Therefore, such closed MDSs system are exposed to blind black-box attacks and can easily be bypassed with adversarial payloads. This vulnerability has attracted the focus of scholars. However, in existing research, adversarial payloads used for blind attacks are generated using static gradient approaches and dynamic features (e.g., API calls) of Portable Executables (PEs). To the best of our knowledge, there is no work on dynamic generation of adversarial payload using static features. To this end, we propose a novel adversarial attack framework. This novel framework is based on Generative Adversarial Networks (GANs) and static attributes of PEs. We designed feed-forward neural networks both for Generator and Discriminator. The Generator is devised to learn distribution modeling of dataset based on static features. Moreover, it dynamically generates adversarial payloads using uniform noise to evade MDS. The proposed model outperformed the traditional static gradient-based generators. A Discriminator is also devised to approximate the MDS. The proposed model demonstrated to generate high quality adversarial instances with zero True Positive Rate (TPR). It is also demonstrated that defense-based retraining of MDS is vulnerable to adversarial payload.
更多查看译文
关键词
Distribution Modeling,Adversarial Examples,Static Analysis,Generative Adversarial Network (GAN),Portable Executable (PE)
AI 理解论文
溯源树
样例
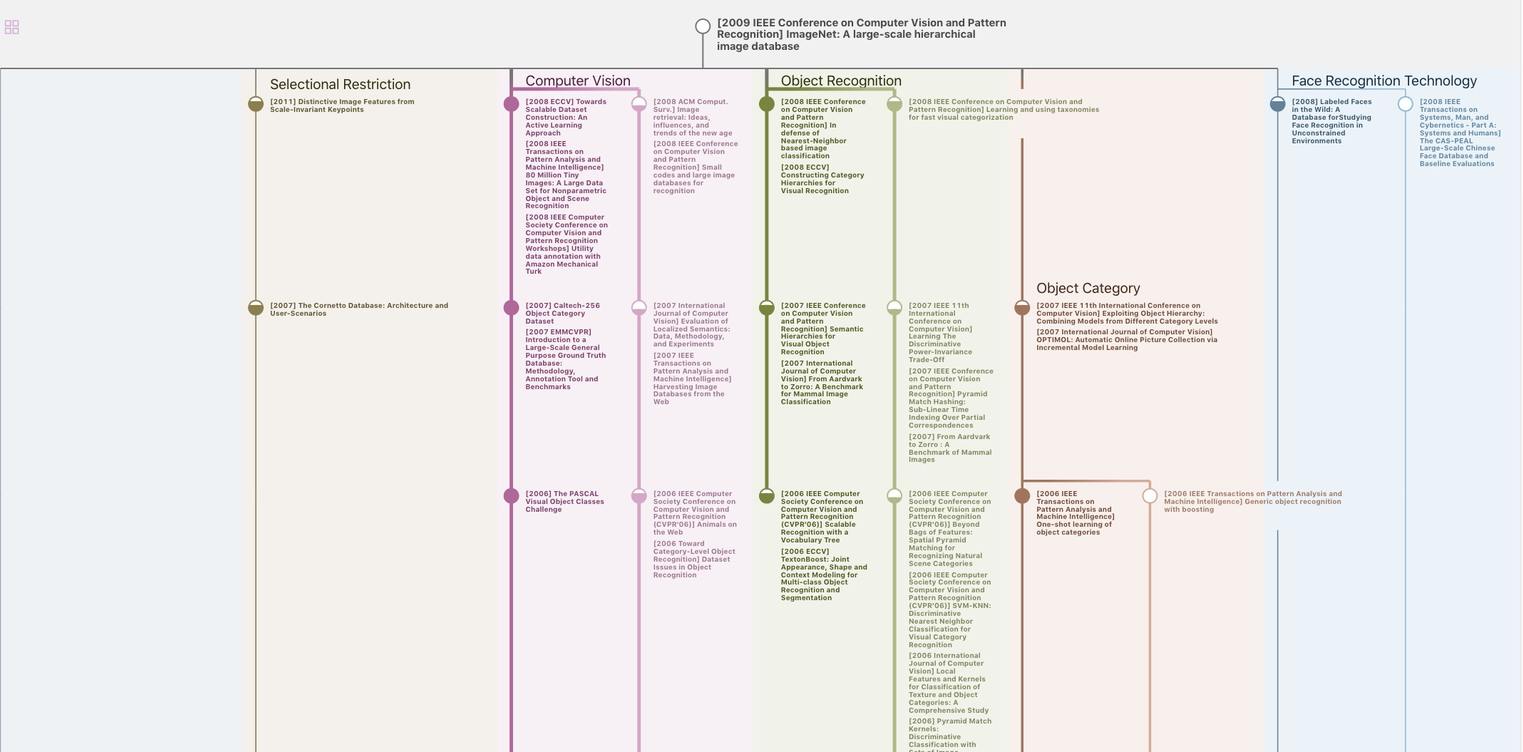
生成溯源树,研究论文发展脉络
Chat Paper
正在生成论文摘要