Forecasting Network Traffic: A Survey and Tutorial With Open-Source Comparative Evaluation.
IEEE Access(2023)
摘要
This paper presents a review of the literature on network traffic prediction, while also serving as a tutorial to the topic. We examine works based on autoregressive moving average models, like ARMA, ARIMA and SARIMA, as well as works based on Artifical Neural Networks approaches, such as RNN, LSTM, GRU, and CNN. In all cases, we provide a complete and self-contained presentation of the mathematical foundations of each technique, which allows the reader to get a full understanding of the operation of the different proposed methods. Further, we perform numerical experiments based on real data sets, which allows comparing the various approaches directly in terms of fitting quality and computational costs. We make our code publicly available, so that readers can readily access a wide range of forecasting tools, and possibly use them as benchmarks for more advanced solutions.
更多查看译文
关键词
Artificial neural networks,forecasting models,network traffic,prediction,statistical models
AI 理解论文
溯源树
样例
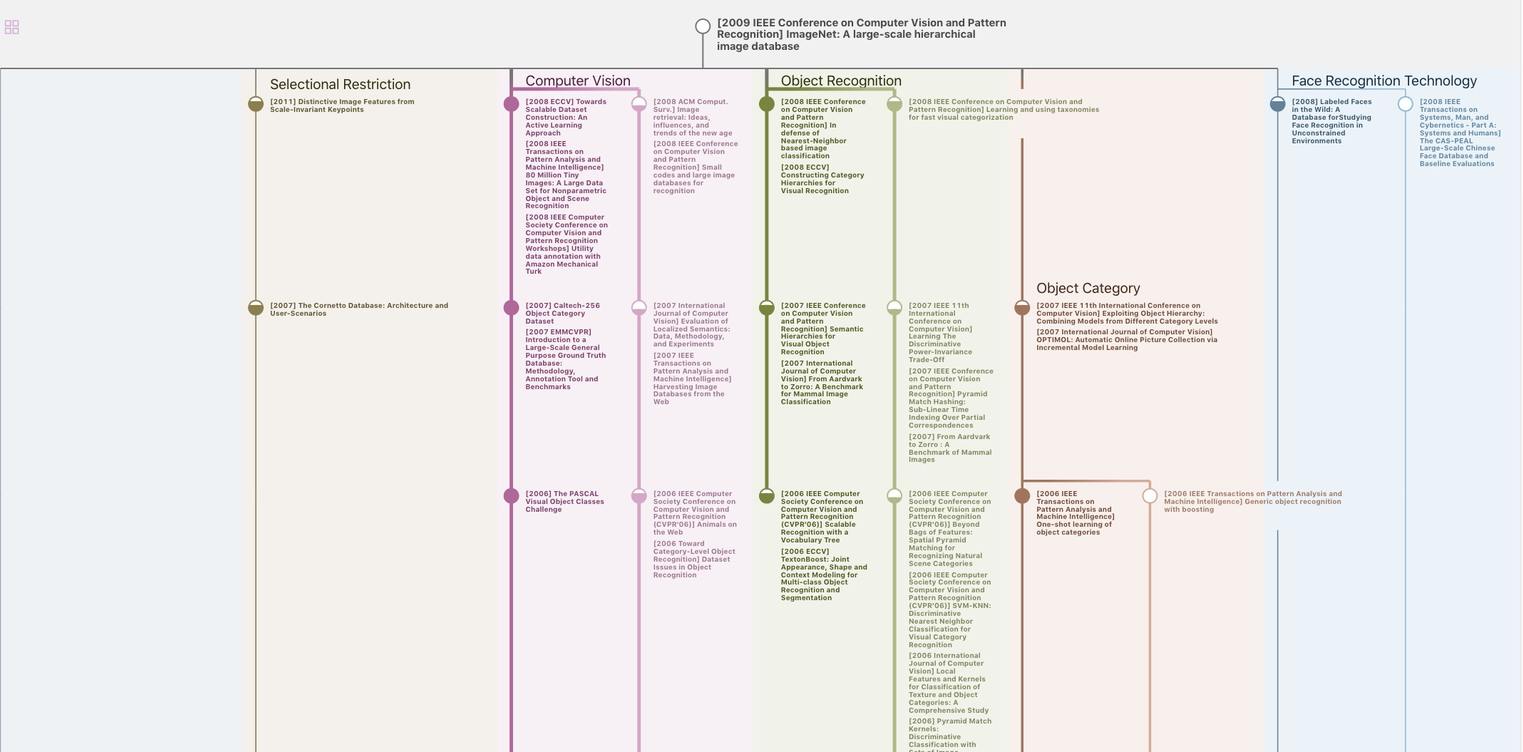
生成溯源树,研究论文发展脉络
Chat Paper
正在生成论文摘要