Exploring uplift modeling with high class imbalance
Data Mining and Knowledge Discovery(2023)
摘要
Uplift modeling refers to individual level causal inference. Existing research on the topic ignores one prevalent and important aspect: high class imbalance. For instance in online environments uplift modeling is used to optimally target ads and discounts, but very few users ever end up clicking an ad or buying. One common approach to deal with imbalance in classification is by undersampling the dataset. In this work, we show how undersampling can be extended to uplift modeling. We propose four undersampling methods for uplift modeling. We compare the proposed methods empirically and show when some methods have a tendency to break down. One key observation is that accounting for the imbalance is particularly important for uplift random forests, which explains the poor performance of the model in earlier works. Undersampling is also crucial for class-variable transformation based models.
更多查看译文
关键词
High class imbalance,Undersampling,Uplift modeling,Heterogeneous treatment effect
AI 理解论文
溯源树
样例
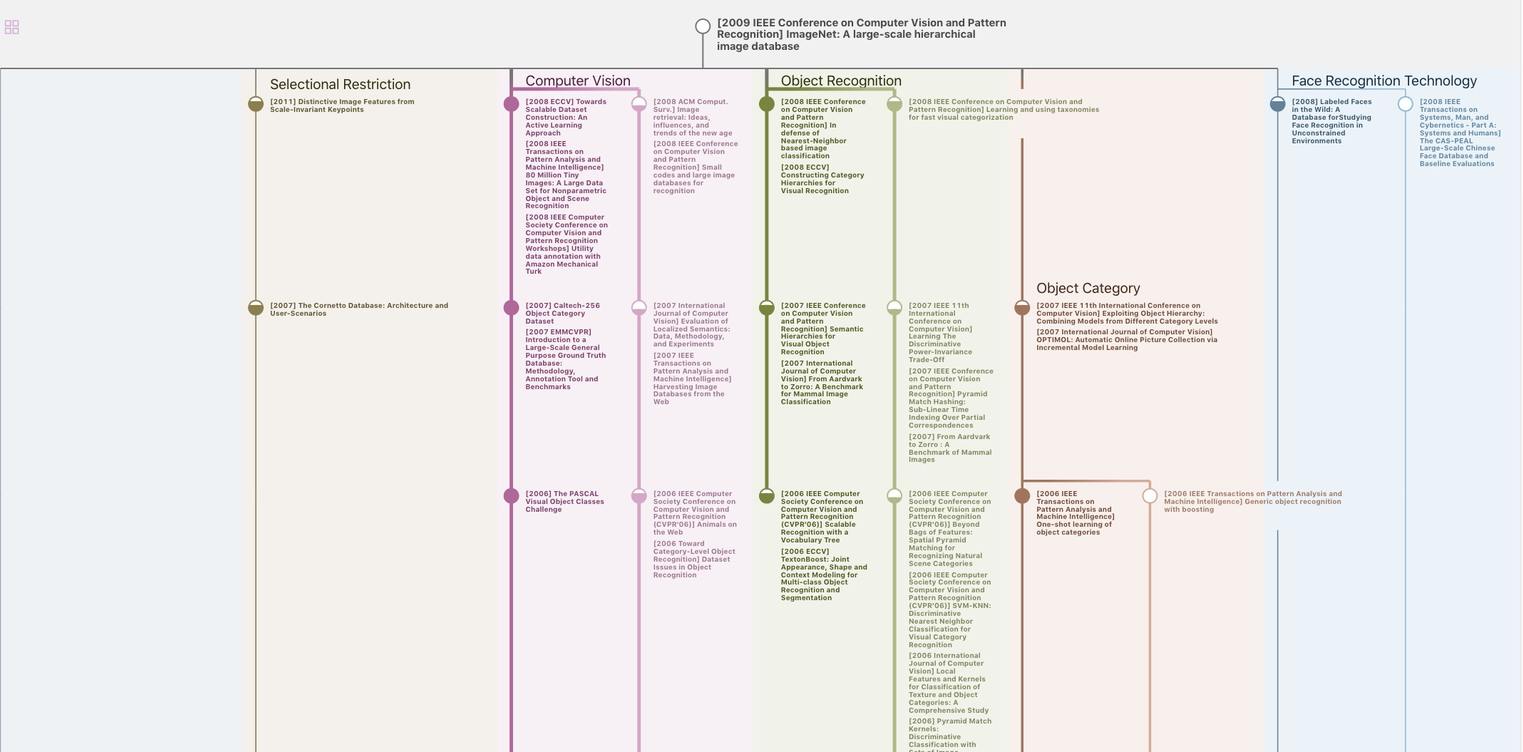
生成溯源树,研究论文发展脉络
Chat Paper
正在生成论文摘要