The framing of time-dependent machine learning models improves risk estimation among young individuals with acute coronary syndromes
Scientific reports(2023)
摘要
Acute coronary syndrome (ACS) is a common cause of death in individuals older than 55 years. Although younger individuals are less frequently seen with ACS, this clinical event has increasing incidence trends, shows high recurrence rates and triggers considerable economic burden. Young individuals with ACS (yACS) are usually underrepresented and show idiosyncratic epidemiologic features compared to older subjects. These differences may justify why available risk prediction models usually penalize yACS with higher false positive rates compared to older subjects. We hypothesized that exploring temporal framing structures such as prediction time, observation windows and subgroup-specific prediction, could improve time-dependent prediction metrics. Among individuals who have experienced ACS (n global_cohort = 6341 and n yACS = 2242), the predictive accuracy for adverse clinical events was optimized by using specific rules for yACS and splitting short-term and long-term prediction windows, leading to the detection of 80% of events, compared to 69% by using a rule designed for the global cohort.
更多查看译文
关键词
acute coronary syndromes,risk estimation,machine learning models,time-dependent
AI 理解论文
溯源树
样例
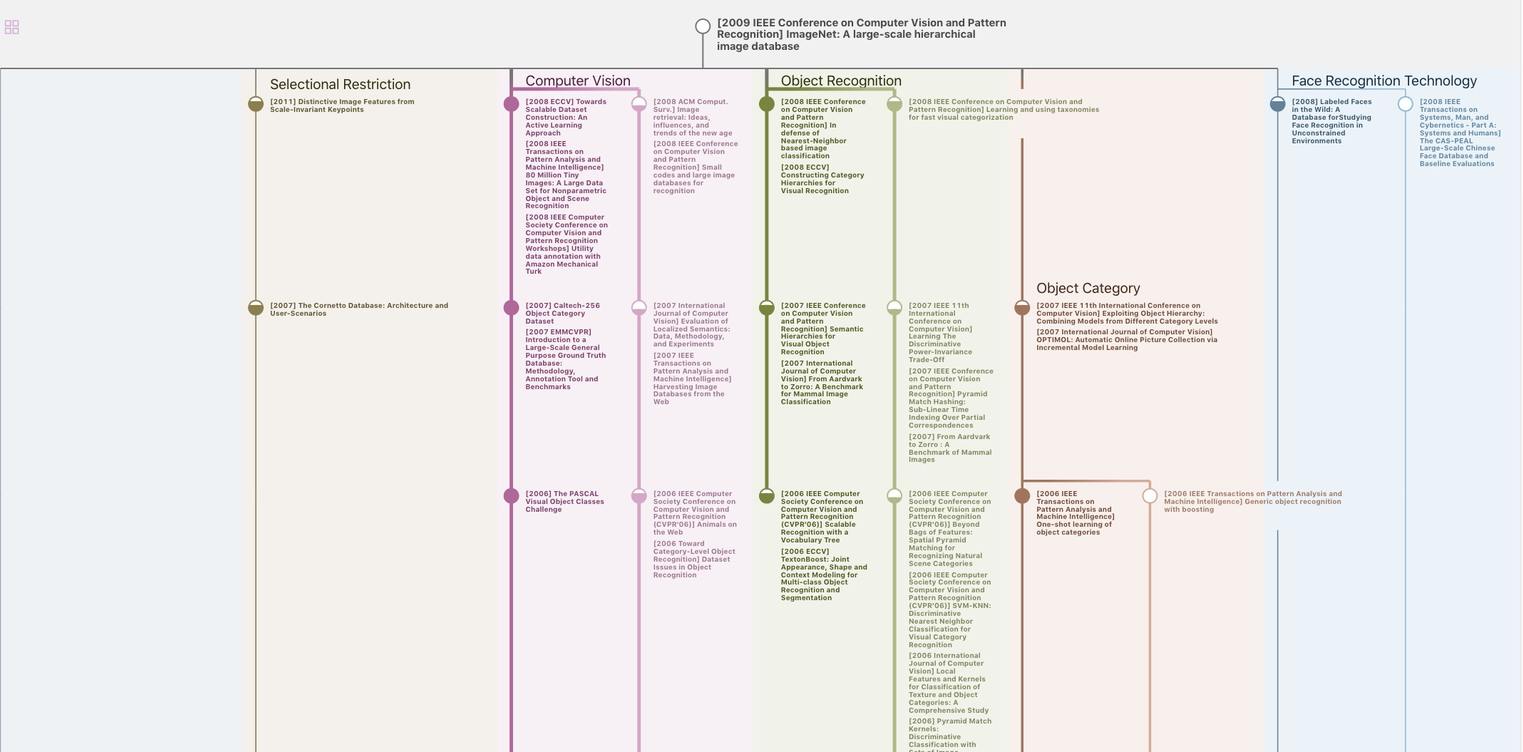
生成溯源树,研究论文发展脉络
Chat Paper
正在生成论文摘要