MTGP: Combining Metamorphic Testing and Genetic Programming
GENETIC PROGRAMMING, EUROGP 2023(2023)
关键词
Semantic Genetic Programming,Genetic Programming,Mutation Testing,Dynamic Test Generation,Program Repair
AI 理解论文
溯源树
样例
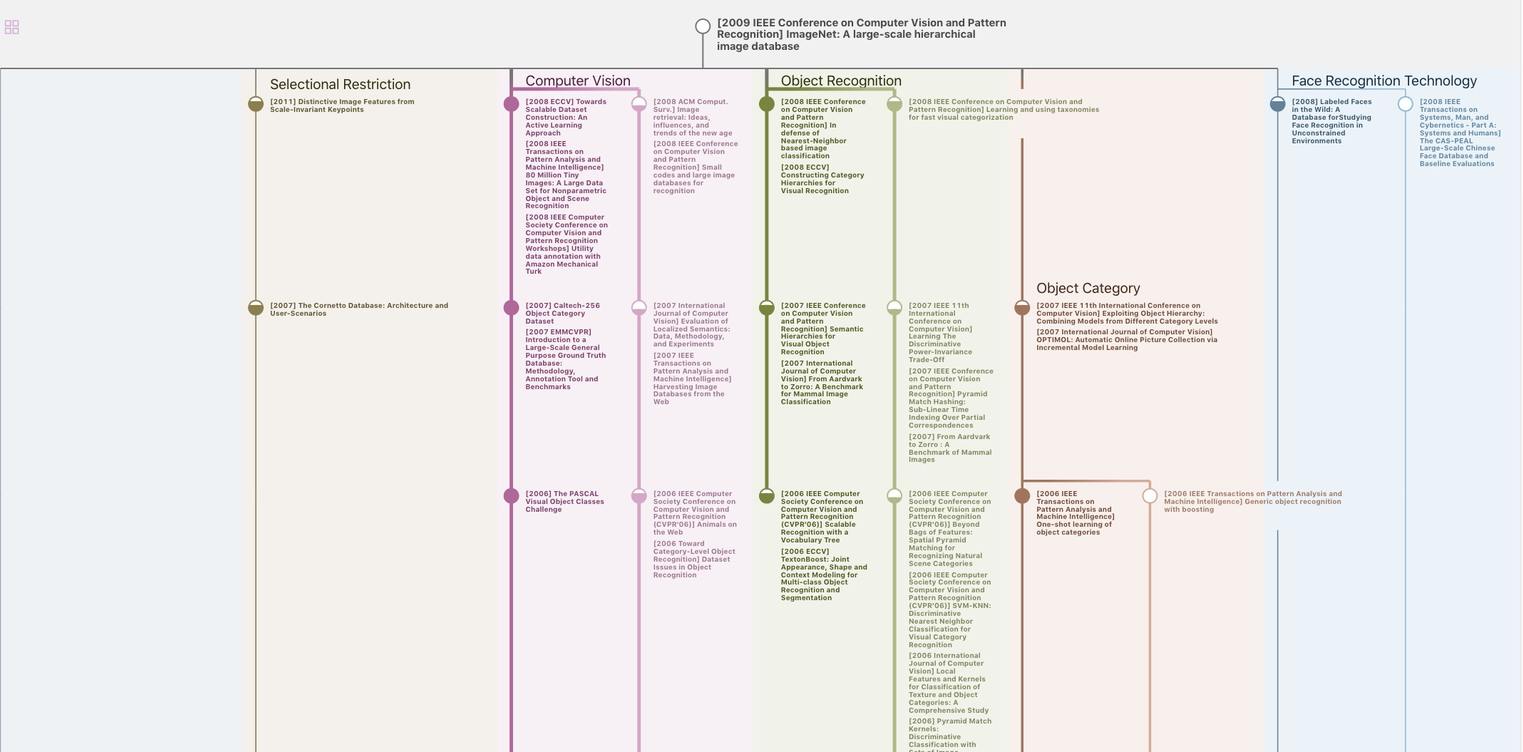
生成溯源树,研究论文发展脉络
Chat Paper
正在生成论文摘要