Impact of a Deep Learning-based Super-resolution Image Reconstruction Technique on High-contrast Computed Tomography: A Phantom Study
Academic radiology(2023)
摘要
Rationale and ObjectivesDeep-learning-based super-resolution image reconstruction (DLSRR) is a novel image reconstruction technique that is expected to contribute to improvement in spatial resolution as well as noise reduction through learning from high-resolution computed tomography (CT). This study aims to evaluate image quality obtained with DLSRR and assess its clinical potential.Materials and MethodsCT images of a Mercury CT 4.0 phantom were obtained using a 320-row multi-detector scanner at tube currents of 100, 200, and 300 mA. Image data were reconstructed by filtered back projection (FBP), hybrid iterative reconstruction (HIR), model-based iterative reconstruction (MBIR), deep-learning-based image reconstruction (DLR), and DLSRR at image reconstruction strength levels of mild, standard, and strong. Noise power spectrum (NPS), task transfer function (TTF), and detectability index were calculated.ResultsThe magnitude of the noise-reducing effect in comparison with FBP was in the order MBIRConclusionThe present results suggest that DLSRR can achieve greater noise reduction and improved spatial resolution in the high-contrast region compared with conventional DLR and iterative reconstruction techniques.
更多查看译文
关键词
deep-learning-based super-resolution image reconstruction,detectability index,iterative reconstruction,noise power spectrum,task transfer function
AI 理解论文
溯源树
样例
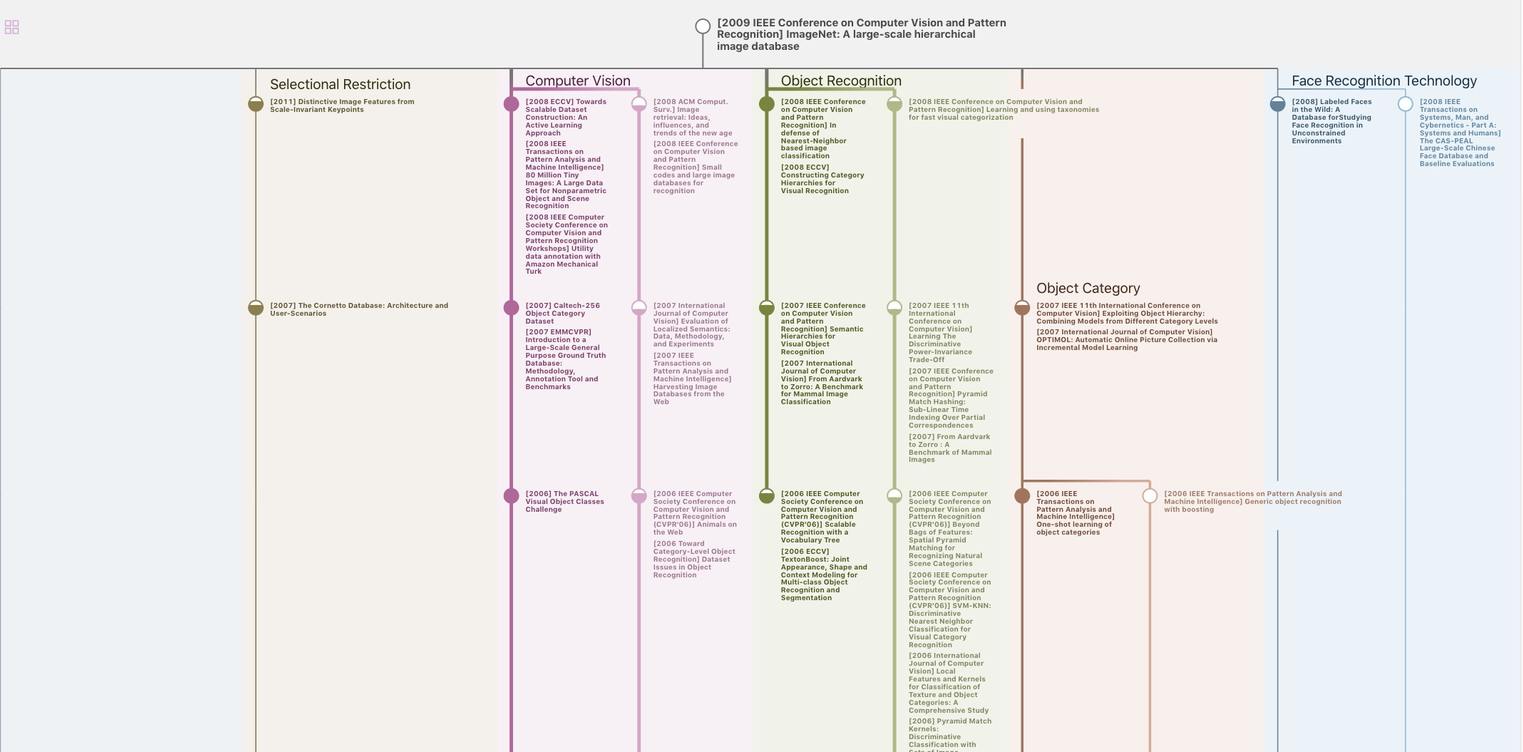
生成溯源树,研究论文发展脉络
Chat Paper
正在生成论文摘要