Performance Enhancement of Satellite-to-Ground FSO System using Deep Learning-Based Detection
2022 9th NAFOSTED Conference on Information and Computer Science (NICS)(2022)
摘要
This paper proposes to use deep learning to improve the performance of satellite-to-ground free-space optical (FSO) communication system under the negative impact of many physical impairments such as atmospheric turbulence, atmospheric attenuation, and free-space path loss. We first implement a simulation of the satellite-to-ground FSO system over Gamma-Gamma turbulence channel using a conventional detection to obtain the binary data at the receiver. This data is subsequently utilized for training the deep learning (DL)-based detection model via a convolutional neural network (CNN) model. Simulation results show that bit-error rate (BER) of the satellite-to-ground FSO communication system is significantly lower than that of the traditional systems. Moreover, we demonstrate that the proposed DL detection model is especially effective in term of the performance gain.
更多查看译文
关键词
free-space optics,atmospheric turbulence,convolutional neural network,semantic segmentation
AI 理解论文
溯源树
样例
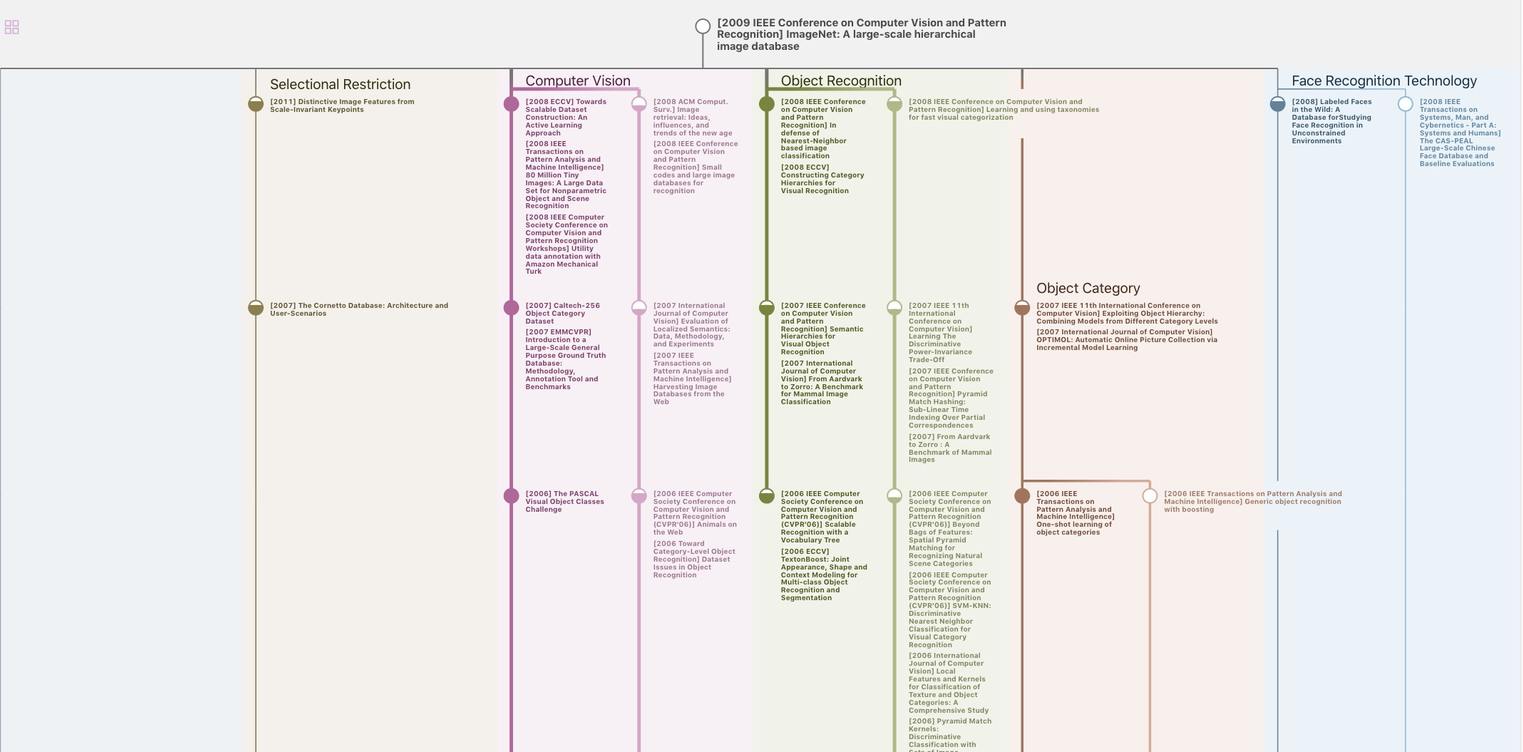
生成溯源树,研究论文发展脉络
Chat Paper
正在生成论文摘要