Using Physics-Informed Neural Networks to Solve Inverse Heat Conduction Problems
AIAA SCITECH 2023 Forum(2023)
摘要
This work investigates the potential of physics-informed neural networks (PINNs) for solving inverse problems in non-homogeneous one-dimensional transient heat conduction equations with limited measurement points. The inverted parameters include constant thermal diffusivity, a pair of volumetric heat capacity and thermal conductivity, a heat generation that varies with space and time, and a spatially varying thermal diffusivity. Several variations in the number of measurement locations, sample sizes, and activation functions used for the neural networks are studied. The results show that only a few measurement points are needed to estimate the unknown quantities with acceptable accuracy, even when measurement errors are considered. However, it is important to use an adequate number of measurement points to capture the physics of the inverse problem correctly. In addition, it is also observed that the choice of activation function can significantly impact the inversion result, in which tanh and Swish yield good accuracy and high robustness.
更多查看译文
关键词
neural networks,heat,physics-informed
AI 理解论文
溯源树
样例
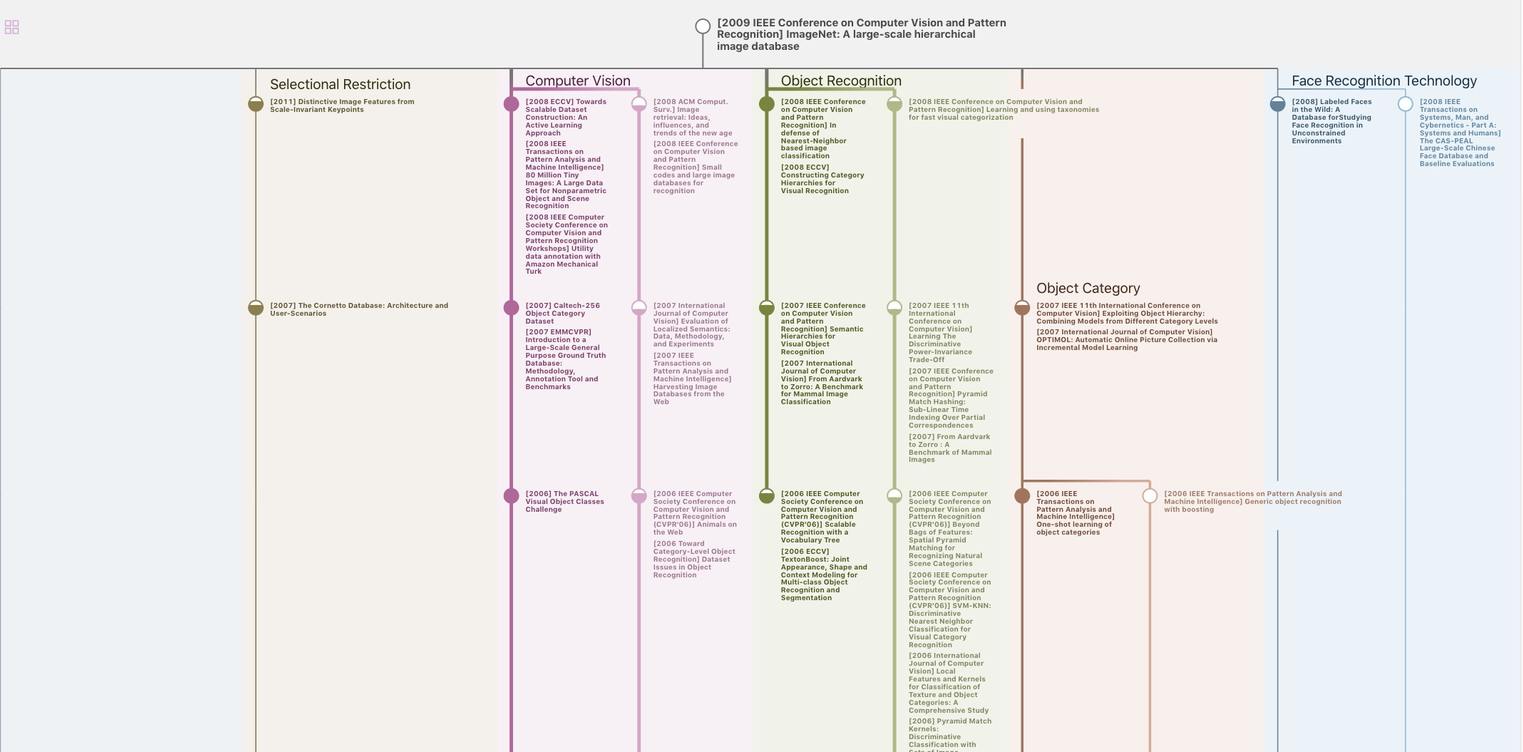
生成溯源树,研究论文发展脉络
Chat Paper
正在生成论文摘要